pennylane
PennyLane is a cross-platform Python library for quantum computing, quantum machine learning, and quantum chemistry. Train a quantum computer the same way as a neural network.
Top Related Projects
Qiskit is an open-source SDK for working with quantum computers at the level of extended quantum circuits, operators, and primitives.
A Python framework for creating, editing, and invoking Noisy Intermediate Scale Quantum (NISQ) circuits.
A Python library for quantum programming using Quil.
Quick Overview
PennyLane is an open-source software framework for quantum machine learning, quantum computing, and quantum chemistry. It provides a way to train quantum circuits using automatic differentiation and integrates with popular machine learning libraries like PyTorch and TensorFlow. PennyLane enables users to easily design and optimize quantum algorithms on various quantum hardware and simulators.
Pros
- Seamless integration with classical machine learning frameworks
- Support for multiple quantum hardware providers and simulators
- Extensive library of built-in quantum operations and templates
- Active development and community support
Cons
- Steep learning curve for those new to quantum computing
- Performance can be limited by the underlying quantum hardware
- Documentation can be overwhelming for beginners
- Some advanced features may require in-depth quantum knowledge
Code Examples
- Creating a simple quantum circuit:
import pennylane as qml
dev = qml.device('default.qubit', wires=2)
@qml.qnode(dev)
def circuit(params):
qml.RX(params[0], wires=0)
qml.RY(params[1], wires=1)
qml.CNOT(wires=[0, 1])
return qml.expval(qml.PauliZ(0))
result = circuit([0.1, 0.2])
print(result)
- Optimizing a variational quantum circuit:
import pennylane as qml
import pennylane.numpy as np
dev = qml.device('default.qubit', wires=2)
@qml.qnode(dev)
def circuit(params):
qml.RX(params[0], wires=0)
qml.RY(params[1], wires=1)
qml.CNOT(wires=[0, 1])
return qml.expval(qml.PauliZ(0))
def cost(params):
return circuit(params)
opt = qml.GradientDescentOptimizer(stepsize=0.4)
params = np.array([0.011, 0.012], requires_grad=True)
for i in range(100):
params = opt.step(cost, params)
print(f"Optimized parameters: {params}")
- Quantum machine learning with PyTorch:
import pennylane as qml
import torch
from torch.autograd import Variable
n_qubits = 4
dev = qml.device("default.qubit", wires=n_qubits)
@qml.qnode(dev, interface="torch")
def quantum_circuit(inputs, weights):
qml.templates.AngleEmbedding(inputs, wires=range(n_qubits))
qml.templates.StronglyEntanglingLayers(weights, wires=range(n_qubits))
return [qml.expval(qml.PauliZ(i)) for i in range(n_qubits)]
layer_1 = torch.nn.Linear(4, 8)
layer_2 = torch.nn.Linear(8, 4)
weights = Variable(torch.randn(3, n_qubits, 3))
def hybrid_model(x):
x = torch.relu(layer_1(x))
x = torch.relu(layer_2(x))
x = quantum_circuit(x, weights)
return torch.sum(x)
x = Variable(torch.randn(4))
output = hybrid_model(x)
print(f"Model output: {output}")
Getting Started
To get started with PennyLane, follow these steps:
-
Install PennyLane and its dependencies:
pip install pennylane
-
Import PennyLane in your Python script:
import pennylane as qml
-
Create a quantum device:
dev = qml.device('default.qubit', wires=2)
-
Define a quantum circuit using the
@qml.qnode
decorator:
Competitor Comparisons
Qiskit is an open-source SDK for working with quantum computers at the level of extended quantum circuits, operators, and primitives.
Pros of Qiskit
- More extensive hardware support, especially for IBM quantum devices
- Larger community and ecosystem, with more resources and tutorials available
- Advanced features for quantum circuit optimization and error mitigation
Cons of Qiskit
- Steeper learning curve for beginners
- Less flexibility in terms of backend integration compared to PennyLane
- More complex installation process with multiple components
Code Comparison
Qiskit:
from qiskit import QuantumCircuit, execute, Aer
qc = QuantumCircuit(2, 2)
qc.h(0)
qc.cx(0, 1)
qc.measure([0, 1], [0, 1])
backend = Aer.get_backend('qasm_simulator')
job = execute(qc, backend, shots=1000)
result = job.result()
PennyLane:
import pennylane as qml
dev = qml.device('default.qubit', wires=2)
@qml.qnode(dev)
def circuit():
qml.Hadamard(wires=0)
qml.CNOT(wires=[0, 1])
return qml.probs(wires=[0, 1])
result = circuit()
Both frameworks offer powerful tools for quantum computing, with Qiskit providing more extensive hardware support and advanced features, while PennyLane offers greater flexibility and ease of use for beginners. The choice between them depends on specific project requirements and user preferences.
A Python framework for creating, editing, and invoking Noisy Intermediate Scale Quantum (NISQ) circuits.
Pros of Cirq
- More comprehensive set of quantum gates and operations
- Better support for noise simulation and error mitigation
- Stronger integration with Google's quantum hardware
Cons of Cirq
- Steeper learning curve for beginners
- Less focus on hybrid quantum-classical algorithms
- More limited support for automatic differentiation
Code Comparison
Cirq example:
import cirq
q0, q1 = cirq.LineQubit.range(2)
circuit = cirq.Circuit(
cirq.H(q0),
cirq.CNOT(q0, q1),
cirq.measure(q0, q1, key='result')
)
PennyLane example:
import pennylane as qml
dev = qml.device('default.qubit', wires=2)
@qml.qnode(dev)
def circuit():
qml.Hadamard(wires=0)
qml.CNOT(wires=[0, 1])
return qml.expval(qml.PauliZ(0) @ qml.PauliZ(1))
Both libraries offer quantum circuit creation and simulation, but Cirq focuses more on low-level circuit manipulation, while PennyLane emphasizes variational quantum algorithms and gradient-based optimization.
A Python library for quantum programming using Quil.
Pros of pyquil
- Specialized for Rigetti quantum hardware, offering deep integration and optimization
- Provides low-level control over quantum operations and pulse-level programming
- Includes tools for noise characterization and mitigation specific to Rigetti devices
Cons of pyquil
- Limited to Rigetti hardware, less flexible for other quantum platforms
- Steeper learning curve due to lower-level abstractions
- Smaller ecosystem and community compared to PennyLane
Code Comparison
pyquil:
from pyquil import Program, get_qc
from pyquil.gates import H, CNOT
p = Program()
p += H(0)
p += CNOT(0, 1)
qc = get_qc('2q-qvm')
result = qc.run_and_measure(p, trials=1000)
PennyLane:
import pennylane as qml
dev = qml.device('default.qubit', wires=2)
@qml.qnode(dev)
def circuit():
qml.Hadamard(wires=0)
qml.CNOT(wires=[0, 1])
return qml.probs(wires=[0, 1])
result = circuit()
Both examples create a simple Bell state, but PennyLane's approach is more abstracted and device-agnostic, while pyquil's is more hardware-specific and lower-level.
Convert
designs to code with AI
Introducing Visual Copilot: A new AI model to turn Figma designs to high quality code using your components.
Try Visual CopilotREADME
PennyLane is a cross-platform Python library for quantum computing, quantum machine learning, and quantum chemistry.
The definitive open-source framework for quantum programming. Built by researchers, for research.
Key Features
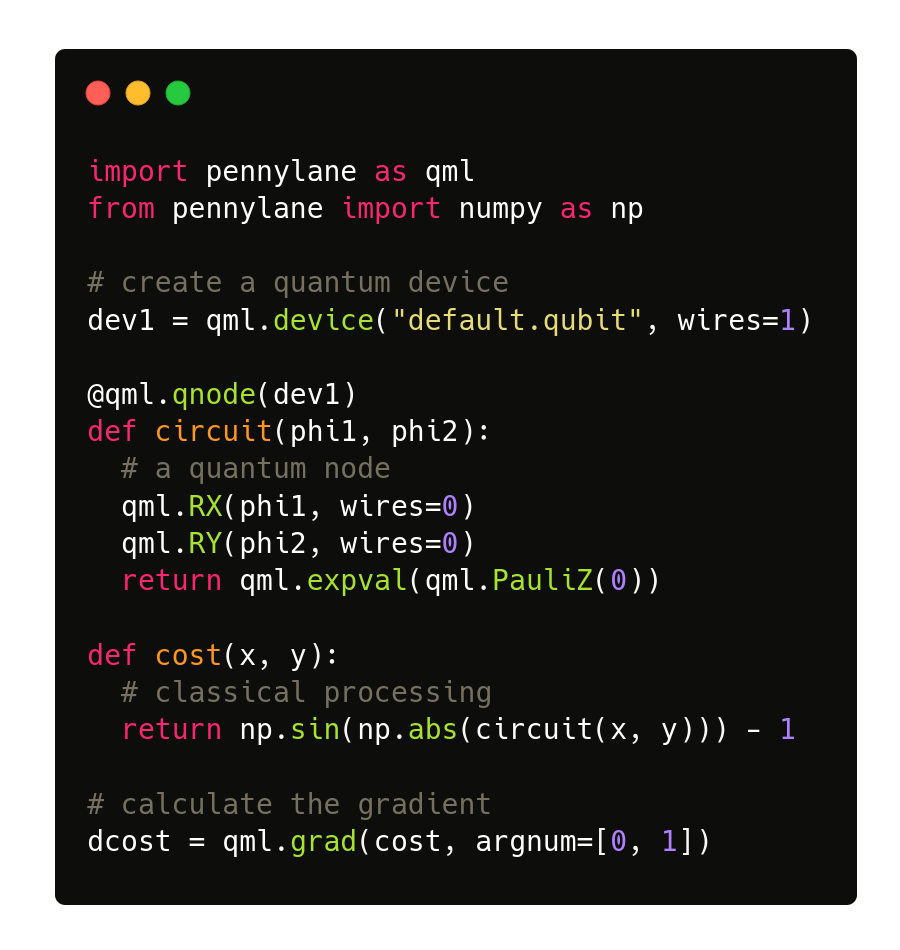
-
Program quantum computers. Build quantum circuits with a wide range of state preparations, gates, and measurements. Run on high-performance simulators or various hardware devices, with advanced features like mid-circuit measurements and error mitigation.
-
Master quantum algorithms. From NISQ to fault-tolerant quantum computing, unlock algorithms for research and application. Analyze performance, visualize circuits, and access tools for quantum chemistry and algorithm development.
-
Machine learning with quantum hardware and simulators. Integrate with PyTorch, TensorFlow, JAX, Keras, or NumPy to define and train hybrid models using quantum-aware optimizers and hardware-compatible gradients for advanced research tasks. Quantum machine learning quickstart.
-
Quantum datasets. Access high-quality, pre-simulated datasets to decrease time-to-research and accelerate algorithm development. Browse the datasets or contribute your own data.
-
Compilation and performance. Experimental support for just-in-time compilation. Compile your entire hybrid workflow, with support for advanced features such as adaptive circuits, real-time measurement feedback, and unbounded loops. See Catalyst for more details.
For more details and additional features, please see the PennyLane website.
Installation
PennyLane requires Python version 3.10 and above. Installation of PennyLane, as well as all dependencies, can be done using pip:
python -m pip install pennylane
Docker support
Docker images are found on the PennyLane Docker Hub page, where there is also a detailed description about PennyLane Docker support. See description here for more information.
Getting started
Get up and running quickly with PennyLane by following our quickstart guide, designed to introduce key features and help you start building quantum circuits right away.
Whether you're exploring quantum machine learning (QML), quantum computing, or quantum chemistry, PennyLane offers a wide range of tools and resources to support your research:

Key Resources:
- Research-oriented Demos
- Learn Quantum Programming with the Codebook and Coding Challenges
- Frequently Asked Questions
- Glossary
- Videos
You can also check out our documentation for quickstart guides to using PennyLane, and detailed developer guides on how to write your own PennyLane-compatible quantum device.
Demos
Take a deeper dive into quantum computing by exploring cutting-edge algorithms using PennyLane and quantum hardware. Explore PennyLane demos.

If you would like to contribute your own demo, see our demo submission guide.
Research Applications
PennyLane is at the forefront of research in quantum computing, quantum machine learning, and quantum chemistry. Explore how PennyLane is used for research in the following publications:
-
Quantum Computing: Fast quantum circuit cutting with randomized measurements
-
Quantum Machine Learning: Better than classical? The subtle art of benchmarking quantum machine learning models
-
Quantum Chemistry: Accelerating Quantum Computations of Chemistry Through Regularized Compressed Double Factorization
Impactful research drives PennyLane. Let us know what features you need for your research on GitHub or on our website.
Contributing to PennyLane
We welcome contributionsâsimply fork the PennyLane repository, and then make a pull request containing your contribution. All contributors to PennyLane will be listed as authors on the releases. All users who contribute significantly to the code (new plugins, new functionality, etc.) will be listed on the PennyLane arXiv paper.
We also encourage bug reports, suggestions for new features and enhancements, and even links to cool projects or applications built on PennyLane.
See our contributions page and our Development guide for more details.
Support
- Source Code: https://github.com/PennyLaneAI/pennylane
- Issue Tracker: https://github.com/PennyLaneAI/pennylane/issues
If you are having issues, please let us know by posting the issue on our GitHub issue tracker.
Join the PennyLane Discussion Forum to connect with the quantum community, get support, and engage directly with our team. Itâs the perfect place to share ideas, ask questions, and collaborate with fellow researchers and developers!
Note that we are committed to providing a friendly, safe, and welcoming environment for all. Please read and respect the Code of Conduct.
Authors
PennyLane is the work of many contributors.
If you are doing research using PennyLane, please cite our paper:
Ville Bergholm et al. PennyLane: Automatic differentiation of hybrid quantum-classical computations. 2018. arXiv:1811.04968
License
PennyLane is free and open source, released under the Apache License, Version 2.0.
Top Related Projects
Qiskit is an open-source SDK for working with quantum computers at the level of extended quantum circuits, operators, and primitives.
A Python framework for creating, editing, and invoking Noisy Intermediate Scale Quantum (NISQ) circuits.
A Python library for quantum programming using Quil.
Convert
designs to code with AI
Introducing Visual Copilot: A new AI model to turn Figma designs to high quality code using your components.
Try Visual Copilot