gateway
A blazing fast AI Gateway with integrated guardrails. Route to 200+ LLMs, 50+ AI Guardrails with 1 fast & friendly API.
Top Related Projects
The official Python library for the OpenAI API
Integrate cutting-edge LLM technology quickly and easily into your apps
🦜🔗 Build context-aware reasoning applications
🤗 Transformers: State-of-the-art Machine Learning for Pytorch, TensorFlow, and JAX.
Quick Overview
Portkey Gateway is an open-source AI gateway that provides a unified interface for multiple AI providers. It allows developers to easily switch between different AI models and providers, manage API keys, and handle rate limiting and retries. The project aims to simplify the integration of AI services into applications.
Pros
- Unified API for multiple AI providers (OpenAI, Anthropic, Azure, etc.)
- Easy switching between different AI models and providers
- Built-in rate limiting and retry mechanisms
- Customizable request/response handling
Cons
- Limited documentation for advanced features
- Relatively new project, may have undiscovered bugs
- Dependency on third-party AI providers
- Potential performance overhead due to the gateway layer
Code Examples
- Basic usage with OpenAI:
from portkey import Portkey
portkey = Portkey(api_key="your-api-key")
response = portkey.chat.completions.create(
model="gpt-3.5-turbo",
messages=[{"role": "user", "content": "Hello, how are you?"}]
)
print(response.choices[0].message.content)
- Switching between providers:
from portkey import Portkey
portkey = Portkey(api_key="your-api-key")
# Using OpenAI
openai_response = portkey.chat.completions.create(
model="gpt-3.5-turbo",
messages=[{"role": "user", "content": "Hello, OpenAI!"}]
)
# Using Anthropic
anthropic_response = portkey.chat.completions.create(
model="claude-2",
messages=[{"role": "user", "content": "Hello, Anthropic!"}]
)
- Custom request handling:
from portkey import Portkey
def custom_handler(request, response):
print(f"Request: {request}")
print(f"Response: {response}")
return response
portkey = Portkey(api_key="your-api-key", trace_id="custom-trace")
portkey.add_handler(custom_handler)
response = portkey.chat.completions.create(
model="gpt-3.5-turbo",
messages=[{"role": "user", "content": "Hello, with custom handling!"}]
)
Getting Started
-
Install Portkey:
pip install portkey-ai
-
Set up your API key:
from portkey import Portkey portkey = Portkey(api_key="your-api-key")
-
Make your first API call:
response = portkey.chat.completions.create( model="gpt-3.5-turbo", messages=[{"role": "user", "content": "Hello, Portkey!"}] ) print(response.choices[0].message.content)
Competitor Comparisons
The official Python library for the OpenAI API
Pros of openai-python
- Official SDK for OpenAI API, ensuring direct compatibility and up-to-date features
- Comprehensive documentation and extensive community support
- Seamless integration with OpenAI's services and models
Cons of openai-python
- Limited to OpenAI's services, lacking support for other AI providers
- No built-in routing or load balancing capabilities
- Requires separate implementation for features like caching and rate limiting
Code Comparison
openai-python:
import openai
openai.api_key = "your-api-key"
response = openai.Completion.create(engine="davinci", prompt="Hello, world!")
print(response.choices[0].text)
gateway:
from portkey import Portkey
portkey = Portkey(api_key="your-api-key")
response = portkey.completions.create(model="gpt-3.5-turbo", prompt="Hello, world!")
print(response.choices[0].text)
The code snippets demonstrate that while openai-python is specifically tailored for OpenAI's services, gateway provides a more flexible interface that can potentially support multiple AI providers. gateway also offers additional features like caching, routing, and observability out of the box, making it a more comprehensive solution for managing AI API interactions.
Integrate cutting-edge LLM technology quickly and easily into your apps
Pros of Semantic Kernel
- More comprehensive framework for building AI applications
- Stronger integration with Azure and Microsoft ecosystem
- Extensive documentation and community support
Cons of Semantic Kernel
- Steeper learning curve due to its complexity
- Primarily focused on Microsoft technologies
- May be overkill for simple API routing needs
Code Comparison
Semantic Kernel (C#):
var kernel = Kernel.Builder.Build();
var function = kernel.CreateSemanticFunction("Generate a story about {{$input}}");
var result = await kernel.RunAsync("a brave knight", function);
Gateway (JavaScript):
const gateway = new Gateway();
gateway.addRoute('/generate', async (req, res) => {
const story = await openai.generateStory(req.body.input);
res.json({ story });
});
Summary
Semantic Kernel is a more comprehensive framework for building AI applications, with strong Microsoft ecosystem integration. However, it has a steeper learning curve and may be excessive for simple tasks. Gateway, on the other hand, focuses on API routing and management, making it more suitable for straightforward AI service integration but potentially less powerful for complex AI application development.
🦜🔗 Build context-aware reasoning applications
Pros of LangChain
- More comprehensive framework for building LLM applications
- Larger community and ecosystem with extensive documentation
- Supports a wider range of LLMs and integrations
Cons of LangChain
- Steeper learning curve due to its extensive features
- Can be overkill for simple LLM projects
- Less focused on API management and routing
Code Comparison
LangChain example:
from langchain import OpenAI, LLMChain, PromptTemplate
llm = OpenAI(temperature=0.9)
prompt = PromptTemplate(
input_variables=["product"],
template="What is a good name for a company that makes {product}?",
)
chain = LLMChain(llm=llm, prompt=prompt)
print(chain.run("colorful socks"))
Gateway example:
from gateway import Gateway
gateway = Gateway()
response = gateway.chat.completions.create(
model="gpt-3.5-turbo",
messages=[{"role": "user", "content": "What is a good name for a company that makes colorful socks?"}]
)
print(response.choices[0].message.content)
The LangChain example showcases its abstraction layers and templating, while the Gateway example demonstrates its simpler, more direct approach to API calls.
🤗 Transformers: State-of-the-art Machine Learning for Pytorch, TensorFlow, and JAX.
Pros of transformers
- Extensive library of pre-trained models for various NLP tasks
- Well-documented with comprehensive examples and tutorials
- Large and active community support
Cons of transformers
- Can be resource-intensive, especially for large models
- Steeper learning curve for beginners in NLP
- May require additional setup for specific hardware acceleration
Code Comparison
transformers:
from transformers import pipeline
classifier = pipeline("sentiment-analysis")
result = classifier("I love this product!")[0]
print(f"Label: {result['label']}, Score: {result['score']:.4f}")
gateway:
from portkey import Portkey
portkey = Portkey(api_key="your_api_key")
response = portkey.chat.completions.create(
model="gpt-3.5-turbo",
messages=[{"role": "user", "content": "Analyze the sentiment: I love this product!"}]
)
print(response.choices[0].message.content)
The transformers library focuses on providing a wide range of pre-trained models for various NLP tasks, while gateway serves as an API gateway for multiple AI providers. transformers is more suitable for in-depth NLP work, while gateway simplifies access to different AI services through a unified interface.
Convert
designs to code with AI
Introducing Visual Copilot: A new AI model to turn Figma designs to high quality code using your components.
Try Visual CopilotREADME
AI Gateway
Route to 250+ LLMs with 1 fast & friendly API
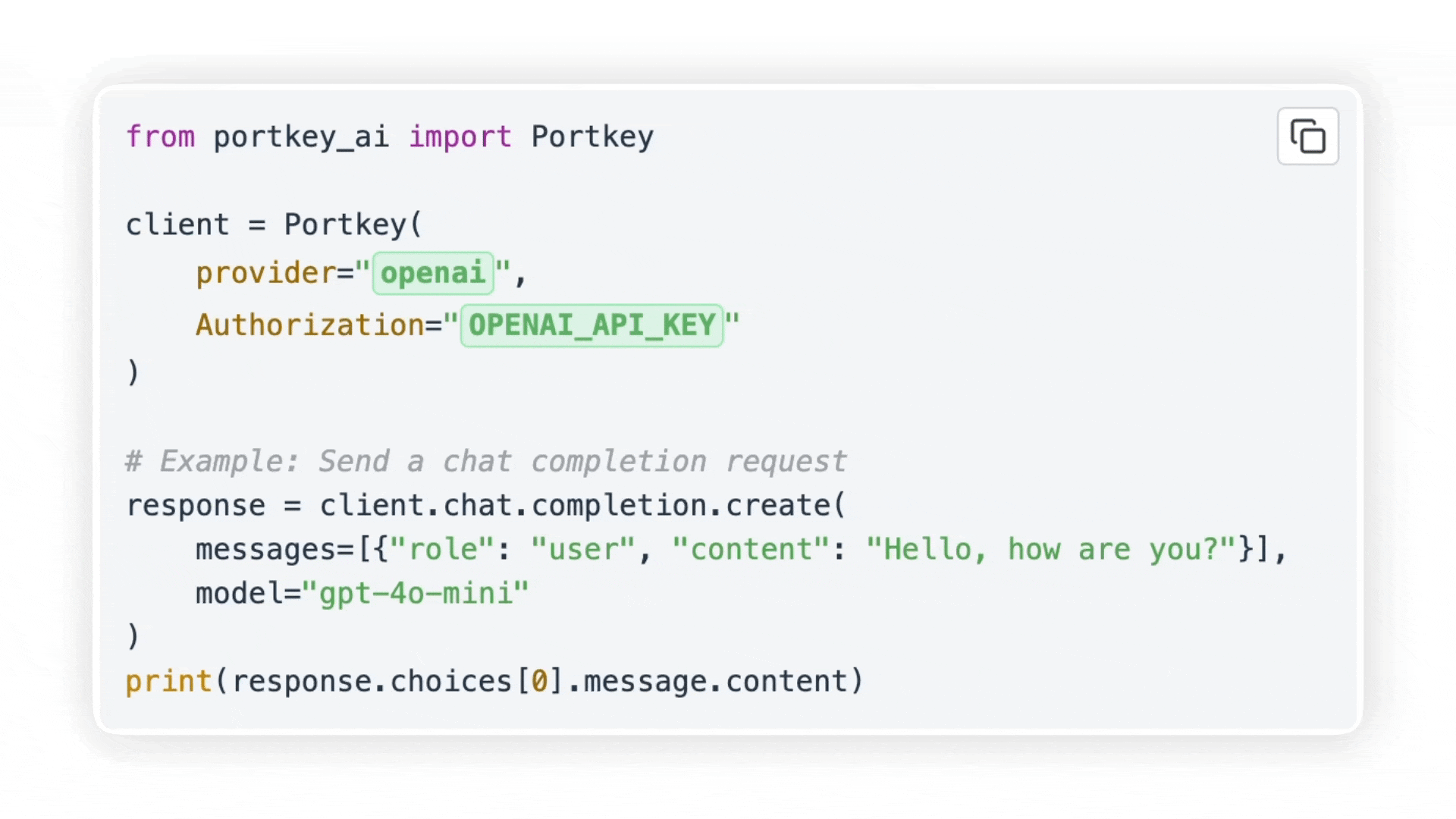
Docs | Enterprise | Hosted Gateway | Changelog | API Reference
The AI Gateway is designed for fast, reliable & secure routing to 1600+ language, vision, audio, and image models. It is a lightweight, open-source, and enterprise-ready solution that allows you to integrate with any language model in under 2 minutes.
- Blazing fast (<1ms latency) with a tiny footprint (122kb)
- Battle tested, with over 10B tokens processed everyday
- Enterprise-ready with enhanced security, scale, and custom deployments
What can you do with the AI Gateway?
- Integrate with any LLM in under 2 minutes - Quickstart
- Prevent downtimes through automatic retries and fallbacks
- Scale AI apps with load balancing and conditional routing
- Protect your AI deployments with guardrails
- Go beyond text with multi-modal capabilities
- Finally, explore agentic workflow integrations
[!TIP] Starring this repo helps more developers discover the AI Gateway ðð»
Quickstart (2 mins)
1. Setup your AI Gateway
# Run the gateway locally (needs Node.js and npm)
npx @portkey-ai/gateway
Deployment guides:The Gateway is running on
http://localhost:8787/v1
The Gateway Console is running on
http://localhost:8787/public/
2. Make your first request
# pip install -qU portkey-ai
from portkey_ai import Portkey
# OpenAI compatible client
client = Portkey(
provider="openai", # or 'anthropic', 'bedrock', 'groq', etc
Authorization="sk-***" # the provider API key
)
# Make a request through your AI Gateway
client.chat.completions.create(
messages=[{"role": "user", "content": "What's the weather like?"}],
model="gpt-4o-mini"
)
Supported Libraries:
JS
Python
REST
OpenAI SDKs
Langchain
LlamaIndex
Autogen
CrewAI
More..
On the Gateway Console (http://localhost:8787/public/
) you can see all of your local logs in one place.
3. Routing & Guardrails
Configs
in the LLM gateway allow you to create routing rules, add reliability and setup guardrails.
config = {
"retry": {"attempts": 5},
"output_guardrails": [{
"default.contains": {"operator": "none", "words": ["Apple"]},
"deny": True
}]
}
# Attach the config to the client
client = client.with_options(config=config)
client.chat.completions.create(
model="gpt-4o-mini",
messages=[{"role": "user", "content": "Reply randomly with Apple or Bat"}]
)
# This would always response with "Bat" as the guardrail denies all replies containing "Apple". The retry config would retry 5 times before giving up.
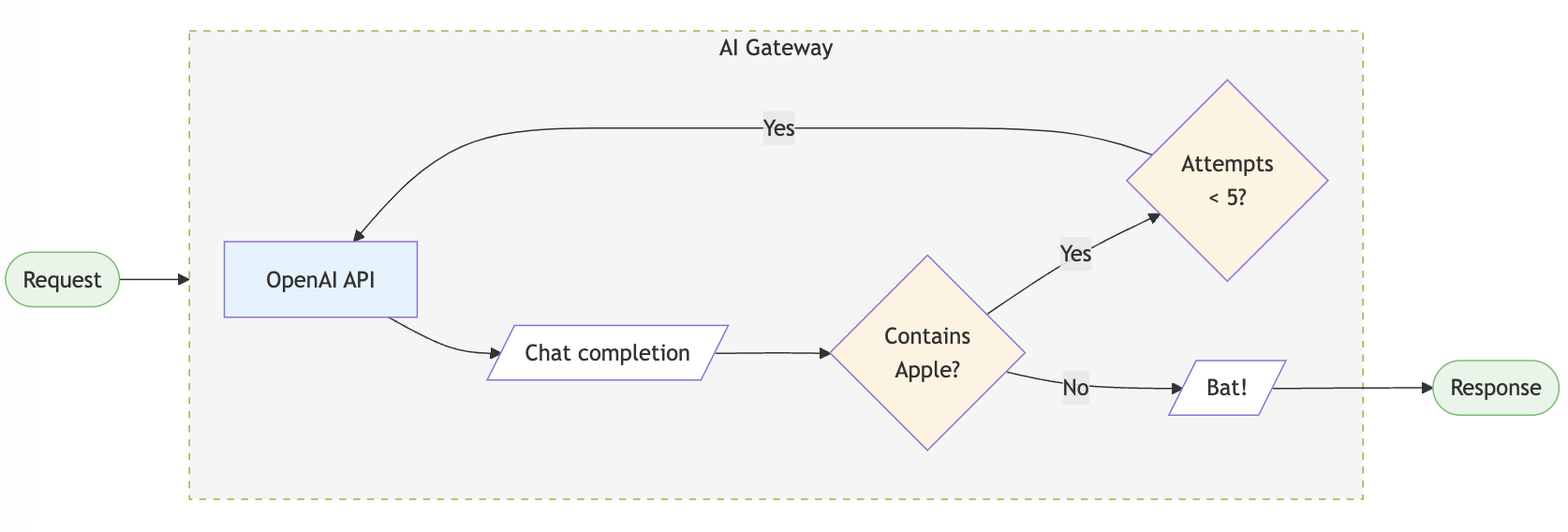
You can do a lot more stuff with configs in your AI gateway. Jump to examples â
Enterprise Version (Private deployments)
AWS
Azure
GCP
OpenShift
Kubernetes
The LLM Gateway's enterprise version offers advanced capabilities for org management, governance, security and more out of the box. View Feature Comparison â
The enterprise deployment architecture for supported platforms is available here - Enterprise Private Cloud Deployments
AI Engineering Hours
Join weekly community calls every Friday (8 AM PT) to kickstart your AI Gateway implementation! Happening every Friday
Minutes of Meetings published here.
LLMs in Prod'25
Insights from analyzing 2 trillion+ tokens, across 90+ regions and 650+ teams in production. What to expect from this report:
- Trends shaping AI adoption and LLM provider growth.
- Benchmarks to optimize speed, cost and reliability.
- Strategies to scale production-grade AI systems.
Core Features
Reliable Routing
- Fallbacks: Fallback to another provider or model on failed requests using the LLM gateway. You can specify the errors on which to trigger the fallback. Improves reliability of your application.
- Automatic Retries: Automatically retry failed requests up to 5 times. An exponential backoff strategy spaces out retry attempts to prevent network overload.
- Load Balancing: Distribute LLM requests across multiple API keys or AI providers with weights to ensure high availability and optimal performance.
- Request Timeouts: Manage unruly LLMs & latencies by setting up granular request timeouts, allowing automatic termination of requests that exceed a specified duration.
- Multi-modal LLM Gateway: Call vision, audio (text-to-speech & speech-to-text), and image generation models from multiple providers â all using the familiar OpenAI signature
- Realtime APIs: Call realtime APIs launched by OpenAI through the integrate websockets server.
Security & Accuracy
- Guardrails: Verify your LLM inputs and outputs to adhere to your specified checks. Choose from the 40+ pre-built guardrails to ensure compliance with security and accuracy standards. You can bring your own guardrails or choose from our many partners.
- Secure Key Management: Use your own keys or generate virtual keys on the fly.
- Role-based access control: Granular access control for your users, workspaces and API keys.
- Compliance & Data Privacy: The AI gateway is SOC2, HIPAA, GDPR, and CCPA compliant.
Cost Management
- Smart caching: Cache responses from LLMs to reduce costs and improve latency. Supports simple and semantic* caching.
- Usage analytics: Monitor and analyze your AI and LLM usage, including request volume, latency, costs and error rates.
- Provider optimization*: Automatically switch to the most cost-effective provider based on usage patterns and pricing models.
Collaboration & Workflows
- Agents Support: Seamlessly integrate with popular agent frameworks to build complex AI applications. The gateway seamlessly integrates with Autogen, CrewAI, LangChain, LlamaIndex, Phidata, Control Flow, and even Custom Agents.
- Prompt Template Management*: Create, manage and version your prompt templates collaboratively through a universal prompt playground.
Cookbooks
âï¸ Trending
- Use models from Nvidia NIM with AI Gateway
- Monitor CrewAI Agents with Portkey!
- Comparing Top 10 LMSYS Models with AI Gateway.
ð¨ Latest
- Create Synthetic Datasets using Nemotron
- Use the LLM Gateway with Vercel's AI SDK
- Monitor Llama Agents with Portkey's LLM Gateway
Supported Providers
Explore Gateway integrations with 45+ providers and 8+ agent frameworks.
Provider | Support | Stream | |
---|---|---|---|
![]() | OpenAI | â | â |
![]() | Azure OpenAI | â | â |
![]() | Anyscale | â | â |
![]() | Google Gemini | â | â |
![]() | Anthropic | â | â |
![]() | Cohere | â | â |
Together AI | â | â | |
Perplexity | â | â | |
Mistral | â | â | |
![]() | Nomic | â | â |
![]() | AI21 | â | â |
Stability AI | â | â | |
DeepInfra | â | â | |
![]() | Ollama | â | â |
Novita AI | â | â |
View the complete list of 200+ supported models here
Agents
Gateway seamlessly integrates with popular agent frameworks. Read the documentation here.
Framework | Call 200+ LLMs | Advanced Routing | Caching | Logging & Tracing* | Observability* | Prompt Management* |
---|---|---|---|---|---|---|
Autogen | â | â | â | â | â | â |
CrewAI | â | â | â | â | â | â |
LangChain | â | â | â | â | â | â |
Phidata | â | â | â | â | â | â |
Llama Index | â | â | â | â | â | â |
Control Flow | â | â | â | â | â | â |
Build Your Own Agents | â | â | â | â | â | â |
*Available on the hosted app. For detailed documentation click here.
Gateway Enterprise Version
Make your AI app more reliable and forward compatible, while ensuring complete data security and privacy.
â
Secure Key Management - for role-based access control and tracking
â
Simple & Semantic Caching - to serve repeat queries faster & save costs
â
Access Control & Inbound Rules - to control which IPs and Geos can connect to your deployments
â
PII Redaction - to automatically remove sensitive data from your requests to prevent indavertent exposure
â
SOC2, ISO, HIPAA, GDPR Compliances - for best security practices
â
Professional Support - along with feature prioritization
Schedule a call to discuss enterprise deployments
Contributing
The easiest way to contribute is to pick an issue with the good first issue
tag ðª. Read the contribution guidelines here.
Bug Report? File here | Feature Request? File here
Getting Started with the Community
Join our weekly AI Engineering Hours every Friday (8 AM PT) to:
- Meet other contributors and community members
- Learn advanced Gateway features and implementation patterns
- Share your experiences and get help
- Stay updated with the latest development priorities
Join the next session â | Meeting notes
Community
Join our growing community around the world, for help, ideas, and discussions on AI.
- View our official Blog
- Chat with us on Discord
- Follow us on Twitter
- Connect with us on LinkedIn
- Read the documentation in Japanese
- Visit us on YouTube
- Join our Dev community
Top Related Projects
The official Python library for the OpenAI API
Integrate cutting-edge LLM technology quickly and easily into your apps
🦜🔗 Build context-aware reasoning applications
🤗 Transformers: State-of-the-art Machine Learning for Pytorch, TensorFlow, and JAX.
Convert
designs to code with AI
Introducing Visual Copilot: A new AI model to turn Figma designs to high quality code using your components.
Try Visual Copilot