Top Related Projects
12 weeks, 26 lessons, 52 quizzes, classic Machine Learning for all
⛔️ DEPRECATED – See https://github.com/ageron/handson-ml3 instead.
The "Python Machine Learning (1st edition)" book code repository and info resource
TensorFlow Tutorial and Examples for Beginners (support TF v1 & v2)
Machine Learning From Scratch. Bare bones NumPy implementations of machine learning models and algorithms with a focus on accessibility. Aims to cover everything from linear regression to deep learning.
📺 Discover the latest machine learning / AI courses on YouTube.
Quick Overview
The apachecn/ailearning
GitHub repository is a comprehensive collection of machine learning and artificial intelligence resources, including tutorials, code examples, and reference materials. It serves as a valuable resource for both beginners and experienced practitioners in the field of AI and machine learning.
Pros
- Comprehensive Content: The repository covers a wide range of topics, from fundamental machine learning concepts to advanced techniques and applications.
- Multilingual Support: The materials are available in multiple languages, including English, Chinese, and others, making it accessible to a global audience.
- Active Community: The project has a vibrant community of contributors, ensuring regular updates and improvements to the content.
- Practical Examples: The repository includes numerous code examples and hands-on tutorials, allowing learners to apply the concepts they've learned.
Cons
- Uneven Quality: As the content is contributed by a large community, the quality and depth of the materials may vary across different sections.
- Lack of Structured Curriculum: The repository is organized as a collection of resources, rather than a structured curriculum, which may make it challenging for beginners to navigate.
- Potential Outdated Content: Given the rapid pace of advancements in AI and machine learning, some of the content may become outdated over time.
- Language Barriers: While the materials are available in multiple languages, learners who are not proficient in the available languages may face difficulties.
Code Examples
The apachecn/ailearning
repository contains a wide range of code examples and tutorials covering various machine learning and AI topics. Here are a few examples:
- Linear Regression:
import numpy as np
from sklearn.linear_model import LinearRegression
# Generate sample data
X = np.array([[1, 1], [1, 2], [2, 2], [2, 3]])
y = np.array([5, 8, 9, 11])
# Create and train the linear regression model
model = LinearRegression()
model.fit(X, y)
# Make a prediction
print(model.predict([[3, 5]]))
This code demonstrates the use of the LinearRegression
model from the scikit-learn library to perform linear regression on a simple dataset.
- K-Means Clustering:
import numpy as np
from sklearn.cluster import KMeans
import matplotlib.pyplot as plt
# Generate sample data
X = np.array([[1, 2], [1, 4], [1, 0], [4, 2], [4, 4], [4, 0]])
# Create and train the K-Means model
model = KMeans(n_clusters=2)
model.fit(X)
# Visualize the clustering results
plt.scatter(X[:, 0], X[:, 1], c=model.labels_, cmap='viridis')
plt.scatter(model.cluster_centers_[:, 0], model.cluster_centers_[:, 1], color='red')
plt.show()
This code demonstrates the use of the KMeans
model from the scikit-learn library to perform K-Means clustering on a simple 2D dataset and visualize the results.
- Convolutional Neural Network (CNN) for Image Classification:
import tensorflow as tf
from tensorflow.keras.models import Sequential
from tensorflow.keras.layers import Conv2D, MaxPooling2D, Flatten, Dense
# Load and preprocess the dataset
(X_train, y_train), (X_test, y_test) = tf.keras.datasets.mnist.load_data()
X_train = X_train.reshape(-1, 28, 28, 1) / 255.0
X_test = X_test.reshape(-1, 28, 28, 1) / 255.0
# Create the CNN model
model = Sequential([
Conv2D(32, (3, 3), activation='relu', input_shape=(28, 28, 1)),
MaxPooling2D((2, 2)),
Conv2D(64, (3, 3), activation='relu'),
MaxPooling2D((2, 2)),
Flatten(),
Dense(64, activation='relu'),
Dense
Competitor Comparisons
12 weeks, 26 lessons, 52 quizzes, classic Machine Learning for all
Pros of ML-For-Beginners
- More structured curriculum with clear learning paths
- Extensive documentation and explanations for each concept
- Multi-language support for code examples
Cons of ML-For-Beginners
- Less focus on advanced topics and cutting-edge techniques
- Fewer practical projects and real-world applications
Code Comparison
ML-For-Beginners:
from sklearn.model_selection import train_test_split
X_train, X_test, y_train, y_test = train_test_split(X, y, test_size=0.2, random_state=42)
ailearning:
from sklearn.model_selection import train_test_split
X_train, X_test, y_train, y_test = train_test_split(X, y, test_size=0.3, random_state=0)
Both repositories use similar code for splitting datasets, with minor differences in parameters.
Summary
ML-For-Beginners offers a more structured approach to learning machine learning, with clear documentation and multi-language support. However, it may lack depth in advanced topics. ailearning provides a broader range of topics and practical applications but may be less organized for beginners. Both repositories use similar code structures for common machine learning tasks.
⛔️ DEPRECATED – See https://github.com/ageron/handson-ml3 instead.
Pros of handson-ml
- More comprehensive coverage of machine learning topics
- Better organized with clear chapter structure
- Includes Jupyter notebooks for interactive learning
Cons of handson-ml
- Less focus on deep learning and neural networks
- Fewer practical examples for real-world applications
Code Comparison
handson-ml:
from sklearn.ensemble import RandomForestClassifier
forest_clf = RandomForestClassifier(n_estimators=100, random_state=42)
forest_clf.fit(X_train, y_train)
y_pred = forest_clf.predict(X_test)
ailearning:
import torch
import torch.nn as nn
class Net(nn.Module):
def __init__(self):
super(Net, self).__init__()
self.conv1 = nn.Conv2d(1, 10, kernel_size=5)
self.conv2 = nn.Conv2d(10, 20, kernel_size=5)
self.fc1 = nn.Linear(320, 50)
self.fc2 = nn.Linear(50, 10)
Summary
handson-ml provides a more structured approach to learning machine learning concepts, with a focus on scikit-learn and traditional ML algorithms. ailearning offers a broader range of topics, including deep learning and neural networks, using frameworks like PyTorch. While handson-ml excels in organization and clarity, ailearning provides more diverse and advanced examples for those interested in cutting-edge AI techniques.
The "Python Machine Learning (1st edition)" book code repository and info resource
Pros of python-machine-learning-book
- More focused on machine learning concepts and implementations
- Provides comprehensive code examples and explanations
- Regularly updated with new content and improvements
Cons of python-machine-learning-book
- Limited coverage of deep learning and neural networks
- Less diverse range of AI topics compared to ailearning
- Primarily in English, which may limit accessibility for non-English speakers
Code Comparison
python-machine-learning-book:
from sklearn.model_selection import train_test_split
X_train, X_test, y_train, y_test = train_test_split(
X, y, test_size=0.3, random_state=1, stratify=y)
ailearning:
import numpy as np
import pandas as pd
from sklearn.model_selection import train_test_split
X_train, X_test, y_train, y_test = train_test_split(X, y, test_size=0.3, random_state=42)
Both repositories provide code examples for machine learning tasks, but python-machine-learning-book tends to offer more detailed explanations and context for each code snippet. The ailearning repository covers a broader range of AI topics and includes content in multiple languages, making it more accessible to a diverse audience. However, python-machine-learning-book is more focused on machine learning specifically and provides a more structured learning path for this subject.
TensorFlow Tutorial and Examples for Beginners (support TF v1 & v2)
Pros of TensorFlow-Examples
- More focused on TensorFlow-specific examples and tutorials
- Cleaner, more organized repository structure
- Regularly updated with newer TensorFlow versions and features
Cons of TensorFlow-Examples
- Limited to TensorFlow framework only
- Less comprehensive coverage of general AI/ML concepts
- Fewer explanations and theoretical background
Code Comparison
TensorFlow-Examples:
import tensorflow as tf
# Create a constant tensor
hello = tf.constant('Hello, TensorFlow!')
# Start a TensorFlow session
sess = tf.Session()
# Run the op
print(sess.run(hello))
AILearning:
import numpy as np
import pandas as pd
from sklearn.model_selection import train_test_split
from sklearn.linear_model import LinearRegression
# Load and prepare data
data = pd.read_csv('data.csv')
X = data[['feature1', 'feature2']]
y = data['target']
# Split data and train model
X_train, X_test, y_train, y_test = train_test_split(X, y, test_size=0.2)
model = LinearRegression().fit(X_train, y_train)
The code comparison shows that TensorFlow-Examples focuses on TensorFlow-specific code, while AILearning covers a broader range of libraries and techniques in machine learning.
Machine Learning From Scratch. Bare bones NumPy implementations of machine learning models and algorithms with a focus on accessibility. Aims to cover everything from linear regression to deep learning.
Pros of ML-From-Scratch
- Focuses on implementing machine learning algorithms from scratch, providing a deeper understanding of the underlying mechanics
- Clear and concise Python implementations with minimal dependencies
- Includes a wide range of algorithms, from basic to advanced
Cons of ML-From-Scratch
- Less comprehensive in terms of overall AI/ML topics compared to AILearning
- Lacks extensive documentation and explanations for each algorithm
- May not cover the latest cutting-edge techniques in the field
Code Comparison
ML-From-Scratch (Linear Regression implementation):
class LinearRegression(Regression):
def fit(self, X, y):
X = np.insert(X, 0, 1, axis=1)
self.w = np.linalg.inv(X.T.dot(X)).dot(X.T).dot(y)
AILearning (Linear Regression implementation):
def fit_normal(X, y):
X = np.insert(X, 0, 1, axis=1)
w = np.linalg.inv(X.T @ X) @ X.T @ y
return w
Both repositories provide implementations of machine learning algorithms, but ML-From-Scratch focuses more on building algorithms from the ground up, while AILearning offers a broader range of AI and machine learning topics with more extensive documentation and resources.
📺 Discover the latest machine learning / AI courses on YouTube.
Pros of ML-YouTube-Courses
- Curated list of high-quality, free ML courses from YouTube
- Organized by topics and skill levels for easy navigation
- Regularly updated with new content and community contributions
Cons of ML-YouTube-Courses
- Limited to video content only, lacking hands-on exercises or projects
- May not cover all AI/ML topics as comprehensively as ailearning
- Dependent on external YouTube links, which may become unavailable
Code Comparison
ML-YouTube-Courses doesn't contain code samples, while ailearning includes practical examples. Here's a snippet from ailearning:
# Example from ailearning
import numpy as np
def sigmoid(x):
return 1 / (1 + np.exp(-x))
def sigmoid_derivative(x):
return x * (1 - x)
ML-YouTube-Courses focuses on organizing and presenting course information:
## Machine Learning
### Beginner
- [Machine Learning — Andrew Ng, Stanford University](https://www.youtube.com/playlist?list=PLLssT5z_DsK-h9vYZkQkYNWcItqhlRJLN)
Both repositories serve different purposes: ML-YouTube-Courses as a curated list of video resources, and ailearning as a comprehensive AI/ML learning platform with code examples and explanations.
Convert
designs to code with AI
Introducing Visual Copilot: A new AI model to turn Figma designs to high quality code using your components.
Try Visual CopilotREADME
AI learning
åè®®ï¼CC BY-NC-SA 4.0
ä¸ç§æ°ææ¯ä¸æ¦å¼å§æµè¡ï¼ä½ è¦ä¹åä¸åè·¯æºï¼è¦ä¹æä¸ºéºè·¯ç³ãââStewart Brand
- å¨çº¿é 读
- å¨çº¿é 读ï¼v1ï¼
- QuantLearning
- ApacheCN ä¸æç¿»è¯ç» 713436582
- ApacheCN å¦ä¹ èµæº
- 注: 广åä½åä½(ç©ç¾ä»·å»)ï¼è¯·èç³» apachecn@163.com
路线å¾
- å ¥é¨åªç: æ¥éª¤ 1 => 2 => 3ï¼ä½ å¯ä»¥å½å¤§çï¼
- ä¸çº§è¡¥å - èµæåº: https://github.com/apachecn/ai-roadmap
è¡¥å
- ç®æ³å·é¢: https://www.ixigua.com/pseries/6822642486343631363/
- é¢è¯æ±è: https://www.ixigua.com/pseries/6822563009391493636/
- æºå¨å¦ä¹ 宿: https://www.ixigua.com/pseries/6822816341615968772/
- NLPæå¦è§é¢: https://www.ixigua.com/pseries/6828241431295951373/
- AI常ç¨å½æ°è¯´æ: https://github.com/apachecn/AiLearning/tree/master/AI常ç¨å½æ°è¯´æ.md
1.æºå¨å¦ä¹ - åºç¡
æ¯æçæ¬
Version | Supported |
---|---|
3.6.x | :x: |
2.7.x | :white_check_mark: |
注æäºé¡¹:
- æºå¨å¦ä¹ 宿: ä» ä» åªæ¯å¦ä¹ ï¼è¯·ä½¿ç¨ python 2.7.x çæ¬ ï¼3.6.x åªæ¯ä¿®æ¹äºé¨åï¼
åºæ¬ä»ç»
- èµææ¥æº: Machine Learning in Action(æºå¨å¦ä¹ 宿-个人ç¬è®°)
- ç»ä¸æ°æ®å°å: https://github.com/apachecn/data
- ç¾åº¦äºæå å°å: https://github.com/apachecn/data/issues/3
- 书ç±ä¸è½½å°å: https://github.com/apachecn/data/tree/master/book
- æºå¨å¦ä¹ ä¸è½½å°å: https://github.com/apachecn/data/tree/master/æºå¨å¦ä¹
- 深度å¦ä¹ æ°æ®å°å: https://github.com/apachecn/data/tree/master/深度å¦ä¹
- æ¨èç³»ç»æ°æ®å°å: https://github.com/apachecn/data/tree/master/æ¨èç³»ç»
- è§é¢ç½ç«: ä¼é · ï¼bilibili / Acfun / ç½æäºè¯¾å ï¼å¯ç´æ¥å¨çº¿ææ¾ãï¼æä¸æ¹æç¸åºé¾æ¥ï¼
- -- æ¨è 红è²ç³å¤´: å°æ¹¾å¤§å¦æè½©ç°æºå¨å¦ä¹ ç¬è®°
- -- æ¨è æºå¨å¦ä¹ ç¬è®°: https://feisky.xyz/machine-learning
å¦ä¹ ææ¡£
ç½ç«è§é¢
å½ç¶æç¥éï¼ç¬¬ä¸å¥å°±ä¼è¢«åæ§½ï¼å 为ç§çåºèº«ç人ï¼ä¸å±çåäºä¸å£å¾æ²«ï¼è¯´å»Xï¼è¿è¯è®º Andrew Ng çè§é¢ãã
æè¿ç¥éè¿æä¸é¨å人ï¼ç Andrew Ng çè§é¢å°±æ¯ç䏿ï¼é£ç¥ç§çæ°å¦æ¨å¯¼ï¼é£è¿·ä¹å¾®ç¬çè±æççæå¦ï¼æä½å°å䏿¯è¿æ ·èµ°è¿æ¥çï¼ï¼ æçå¿å¯è½æ¯ä½ 们é½çï¼å 为æå¨ç½ä¸æ¶èè¿ä¸10é¨ãæºå¨å¦ä¹ ãç¸å ³è§é¢ï¼å¤å å½å æ¬å飿 ¼çæç¨: 7æ+å°è±¡ ççï¼æé½å¾é¾å»å¬æï¼ç´å°æä¸å¤©ï¼è¢«ä¸ä¸ªç¾åº¦çé«çº§ç®æ³åæå¸æ¨è说: ãæºå¨å¦ä¹ 宿ãè¿ä¸éï¼éä¿ææï¼ä½ å»è¯è¯ï¼ï¼
æè¯äºè¯ï¼è¿å¥½æçPythonåºç¡åè°è¯è½åè¿ä¸éï¼åºæ¬ä¸ä»£ç é½è°è¯è¿ä¸éï¼å¾å¤é«å¤§ä¸ç "ç论+æ¨å¯¼"ï¼å¨æç¼ä¸åæäºå 个 "å åä¹é¤+循ç¯"ï¼ææ³è¿ä¸å°±æ¯åæè¿æ ·çç¨åºåæ³è¦çå ¥é¨æç¨ä¹ï¼
å¾å¤ç¨åºå说æºå¨å¦ä¹ TM 太é¾å¦äºï¼æ¯çï¼ç TM é¾å¦ï¼ææ³æé¾çæ¯: 没æä¸æ¬åãæºå¨å¦ä¹ 宿ã飿 ·çä½è æ¿æä»¥ç¨åºå Coding è§åº¦å»ç»å¤§å®¶è®²è§£ï¼ï¼
æè¿å 天ï¼GitHub æ¶¨äº 300é¢ starï¼å 群ç200äººï¼ ç°å¨è¿å¨ä¸æçå¢å ++ï¼ææ³å¤§å®¶å¯è½é½æ¯æå身åå§ï¼
å¾å¤æ³å ¥é¨æ°æå°±æ¯è¢«å¿½æ çæ¶èæ¶èåæ¶èï¼ä½æ¯æåè¿æ¯ä»ä¹é½æ²¡æå¦å°ï¼ä¹å°±æ¯"èµæºæ¶èå®¶"ï¼ä¹è®¸æ°æè¦çå°±æ¯ MachineLearning(æºå¨å¦ä¹ ) å¦ä¹ 路线å¾ã没éï¼æå¯ä»¥ç»ä½ 们çä¸ä»½ï¼å 为æä»¬è¿éè¿è§é¢è®°å½ä¸æ¥æä»¬çå¦ä¹ è¿ç¨ãæ°´å¹³å½ç¶ä¹æéï¼ä¸è¿å¯¹äºæ°æå ¥é¨ï¼ç»å¯¹æ²¡é®é¢ï¼å¦æä½ è¿ä¸ä¼ï¼é£ç®æè¾ï¼ï¼
è§é¢æä¹çï¼
- ç论ç§çåºèº«-建议å»å¦ä¹ Andrew Ng çè§é¢ï¼Ng çè§é¢ç»å¯¹æ¯æå¨ï¼è¿ä¸ªæ¯åº¸ç½®çï¼
- ç¼ç è½å强 - 建议çæä»¬çãæºå¨å¦ä¹ 宿-æå¦çã
- ç¼ç è½åå¼± - 建议çæä»¬çãæºå¨å¦ä¹ 宿-讨论çãï¼ä¸è¿å¨ççè®ºçæ¶åï¼ç æå¦ç-ç论é¨åï¼è®¨è®ºççåºè¯å¤ªå¤ï¼ä¸è¿å¨è®²è§£ä»£ç çæ¶åæ¯ä¸è¡ä¸è¡è®²è§£çï¼æä»¥ï¼æ ¹æ®èªå·±çéæ±ï¼èªç±çç»åã
ãå è´¹ãæ°å¦æå¦è§é¢ - 坿±å¦é¢ å ¥é¨ç¯
- @äºæ¯æ¢ æ¨è: 坿±å¦é¢-ç½æå ¬å¼è¯¾
æ¦ç | ç»è®¡ | 线æ§ä»£æ° |
---|---|---|
坿±å¦é¢(æ¦ç) | 坿±å¦é¢(ç»è®¡å¦) | 坿±å¦é¢(线æ§ä»£æ°) |
æºå¨å¦ä¹ è§é¢ - ApacheCN æå¦ç
AcFun | Bç« |
![]() | ![]() |
ä¼é · | ç½æäºè¯¾å |
![]() | ![]() |
ãå è´¹ãæºå¨/深度å¦ä¹ è§é¢ - å´æ©è¾¾
æºå¨å¦ä¹ | 深度å¦ä¹ |
---|---|
å´æ©è¾¾æºå¨å¦ä¹ | ç¥ç»ç½ç»å深度å¦ä¹ |
2.深度å¦ä¹
æ¯æçæ¬
Version | Supported |
---|---|
3.6.x | :white_check_mark: |
2.7.x | :x: |
å ¥é¨åºç¡
- ååä¼ é: https://www.cnblogs.com/charlotte77/p/5629865.html
- CNNåç: http://www.cnblogs.com/charlotte77/p/7759802.html
- RNNåç: https://blog.csdn.net/qq_39422642/article/details/78676567
- LSTMåç: https://blog.csdn.net/weixin_42111770/article/details/80900575
Pytorch - æç¨
-- å¾ æ´æ°
TensorFlow 2.0 - æç¨
-- å¾ æ´æ°
ç®å½ç»æ:
- å®è£ æå
- Keras å¿«éå ¥é¨
- å®æé¡¹ç® 1 çµå½±æ æåç±»
- å®æé¡¹ç® 2 æ±½è½¦çæ²¹æç
- å®æé¡¹ç® 3 ä¼å è¿æå忬 æå
- å®æé¡¹ç® 4 å¤è¯è¯èªå¨çæ
ååï¼åè¯ï¼
è¯æ§æ 注
å½åå®ä½è¯å«
奿³åæ
WordNetå¯ä»¥è¢«ç使¯ä¸ä¸ªåä¹è¯è¯å ¸
è¯å¹²æåï¼stemmingï¼ä¸è¯å½¢è¿åï¼lemmatizationï¼
TensorFlow 2.0å¦ä¹ ç½å
3.èªç¶è¯è¨å¤ç
æ¯æçæ¬
Version | Supported |
---|---|
3.6.x | :white_check_mark: |
2.7.x | :x: |
å¦ä¹ è¿ç¨ä¸-å å¿å¤æçååï¼ï¼ï¼
èªä»å¦ä¹ NLP以åï¼æåç°å½å
ä¸å½å¤çå
¸ååºå«:
1. å¯¹èµæºçæåº¦æ¯å®å
¨ç¸åç:
1) å½å
: 就好å为äºåæ°ï¼ä¸¾åå·¥ä½è£
é¼çä¼è®®ï¼å°±æ¯æ²¡æå¹²è´§ï¼å
¨é¨é½æ¯è±¡å¾æ§çPPTä»ç»ï¼ä¸æ¯é对å¨åçåä½
2ï¼å½å¤: å°±å¥½åæ¯ä¸ºäºæ¨å¨nlpè¿æ¥ä¸æ ·ï¼å享è
åç§å¹²è´§èµæåå
·ä½çå®ç°ãï¼ç¹å«æ¯: pythonèªç¶è¯è¨å¤çï¼
2. 论æçå®ç°:
1) åç§é«å¤§ä¸ç论æå®ç°ï¼å´è¿æ¯æ²¡çå°ä¸ä¸ªåæ ·çGitHub项ç®ï¼ï¼å¯è½æçæç´¢è½åå·®äºç¹ï¼ä¸ç´æ²¡æ¾å°ï¼
2ï¼å½å¤å°±ä¸ä¸¾ä¾äºï¼æç䏿ï¼
3. 弿ºçæ¡æ¶
1ï¼å½å¤ç弿ºæ¡æ¶: tensorflow/pytorch ææ¡£+æç¨+è§é¢ï¼å®æ¹æä¾ï¼
2) å½å
ç弿ºæ¡æ¶: é¢é¢ï¼è¿ç举ä¾ä¸åºæ¥ï¼ä½æ¯çé¼å¹å¾ä¸æ¯å½å¤å·®ï¼ï¼MXNetè½ç¶æä¼å¤å½äººåä¸å¼åï¼ä½ä¸è½ç®æ¯å½å
弿ºæ¡æ¶ãåºäºMXNetç卿妿·±åº¦å¦ä¹ (http://zh.d2l.ai & https://discuss.gluon.ai/t/topic/753)䏿æç¨,å·²ç»ç±æ²ç¥(ææ²)以åé¿æ¯é¡¿Â·å¼ 讲æå½å¶ï¼å
¬å¼åå¸(ææ¡£+第ä¸å£æç¨+è§é¢ï¼ã)
æ¯ä¸æ¬¡æ·±å
¥é½è¦å»ç¿»å¢ï¼æ¯ä¸æ¬¡æ·±å
¥é½è¦Googleï¼æ¯ä¸æ¬¡ççå½å
ç说: å工大ã讯é£ãä¸ç§å¤§ãç¾åº¦ãé¿éå¤çé¼ï¼ä½æ¯èµæè¿æ¯å¾å½å¤å»æ¾ï¼
ææ¶åççæºæ¨çï¼ççæç¹ç§ä¸èµ·èªå·±å½å
çææ¯ç¯å¢ï¼
å½ç¶è°¢è°¢å½å
å¾å¤å客大佬ï¼ç¹å«æ¯ä¸äºå
¥é¨çDemoååºæ¬æ¦å¿µããæ·±å
¥çæ°´å¹³æéï¼æ²¡çæã
- ãå ¥é¨é¡»ç¥ãå¿ é¡»äºè§£: https://github.com/apachecn/AiLearning/tree/master/nlp
- ãå ¥é¨æç¨ãå¼ºçæ¨è: PyTorch èªç¶è¯è¨å¤ç: https://github.com/apachecn/NLP-with-PyTorch
- Python èªç¶è¯è¨å¤ç 第äºç: https://usyiyi.github.io/nlp-py-2e-zh
- æ¨èä¸ä¸ªliuhuanyong大佬æ´ççnlpå ¨é¢ç¥è¯ä½ç³»: https://liuhuanyong.github.io
- 弿º - è¯åéåºéå:
- https://www.cnblogs.com/Darwin2000/p/5786984.html
- https://ai.tencent.com/ailab/nlp/embedding.html
- https://blog.csdn.net/xiezj007/article/details/85073890
- https://github.com/Embedding/Chinese-Word-Vectors
- https://github.com/brightmart/nlp_chinese_corpus
- https://github.com/codemayq/chinese_chatbot_corpus
- https://github.com/candlewill/Dialog_Corpus
1.使ç¨åºæ¯ ï¼ç¾åº¦å ¬å¼è¯¾ï¼
第ä¸é¨å å ¥é¨ä»ç»
第äºé¨å æºå¨ç¿»è¯
- 2.) æºå¨ç¿»è¯
第ä¸é¨å ç¯ç« åæ
- 3.1.) ç¯ç« åæ-å 容æ¦è¿°
- 3.2.) ç¯ç« åæ-å 容æ ç¾
- 3.3.) ç¯ç« åæ-æ æåæ
- 3.4.) ç¯ç« åæ-èªå¨æè¦
第åé¨å UNIT-è¯è¨çè§£ä¸äº¤äºææ¯
åºç¨é¢å
䏿åè¯:
- æå»ºDAGå¾
- 卿è§åæ¥æ¾ï¼ç»¼åæ£ååï¼æ£åå æååè¾åºï¼æ±å¾DAGæå¤§æ¦çè·¯å¾
- 使ç¨äºSBMEè¯æè®ç»äºä¸å¥ HMM + Viterbi 模åï¼è§£å³æªç»å½è¯é®é¢
1.ææ¬åç±»ï¼Text Classificationï¼
ææ¬åç±»æ¯ææ è®°å¥åæææ¡£ï¼ä¾å¦çµåé®ä»¶åå¾é®ä»¶åç±»åæ æåæã
ä¸é¢æ¯ä¸äºå¾å¥½çåå¦è ææ¬åç±»æ°æ®éã
- è·¯é社Newswire主é¢åç±»ï¼è·¯é社-21578ï¼ã1987å¹´è·¯é社åºç°çä¸ç³»åæ°é»æä»¶ï¼æç±»å«ç¼å¶ç´¢å¼ãå¦è§RCV1ï¼RCV2åTRC2ã
- IMDBçµå½±è¯è®ºæ æåç±»ï¼æ¯å¦ç¦ï¼ãæ¥èªç½ç«imdb.comçä¸ç³»åçµå½±è¯è®ºåå ¶ç§¯æææ¶æçæ 绪ã
- æ°é»ç»çµå½±è¯è®ºæ æåç±»ï¼åº·å¥å°ï¼ãæ¥èªç½ç«imdb.comçä¸ç³»åçµå½±è¯è®ºåå ¶ç§¯æææ¶æçæ 绪ã
æå ³æ´å¤ä¿¡æ¯ï¼è¯·åé å¸å: åæ ç¾ææ¬åç±»çæ°æ®éã
æ æåæ
æ¯èµå°å: https://www.kaggle.com/c/word2vec-nlp-tutorial
- æ¹æ¡ä¸(0.86): WordCount + æ´ç´ Bayes
- æ¹æ¡äº(0.94): LDA + å类模åï¼knn/å³çæ /é»è¾åå½/svm/xgboost/éæºæ£®æï¼
- a) å³çæ ææä¸æ¯å¾å¥½ï¼è¿ç§è¿ç»ç¹å¾ä¸å¤ªéåç
- b) éè¿åæ°è°æ´ 200 个topicï¼ä¿¡æ¯éä¿åææè¾ä¼ï¼è®¡ç®ä¸»é¢ï¼
- æ¹æ¡ä¸(0.72): word2vec + CNN
- 说å®è¯: 没æä¸ä¸ªå¥½çæºå¨ï¼æ¯è°ä¸åºæ¥ä¸ä¸ªå¥½çç»æ (: é
éè¿AUC æ¥è¯ä¼°æ¨¡åçææ
2.è¯è¨æ¨¡åï¼Language Modelingï¼
è¯è¨å»ºæ¨¡æ¶åå¼åä¸ç§ç»è®¡æ¨¡åï¼ç¨äºé¢æµå¥åä¸çä¸ä¸ä¸ªåè¯æä¸ä¸ªåè¯ä¸çä¸ä¸ä¸ªåè¯ã宿¯è¯é³è¯å«åæºå¨ç¿»è¯çä»»å¡ä¸çå置任å¡ã
宿¯è¯é³è¯å«åæºå¨ç¿»è¯çä»»å¡ä¸çå置任å¡ã
ä¸é¢æ¯ä¸äºå¾å¥½çåå¦è è¯è¨å»ºæ¨¡æ°æ®éã
- å¤è ¾å ¡é¡¹ç®ï¼ä¸ç³»åå 费书ç±ï¼å¯ä»¥ç¨çº¯ææ¬æ£ç´¢åç§è¯è¨ã
- è¿ææ´å¤æ£å¼çè¯æåºå¾å°äºå¾å¥½çç ç©¶; ä¾å¦: 叿大å¦ç°ä»£ç¾å½è±è¯æ åè¯æåºã大éè±è¯åè¯æ ·æ¬ã è°·æ10亿åè¯æåºã
æ°è¯åç°
- 䏿åè¯æ°è¯åç°
- python3å©ç¨äºä¿¡æ¯åå·¦å³ä¿¡æ¯çµç䏿åè¯æ°è¯åç°
- https://github.com/zhanzecheng/Chinese_segment_augment
å¥åç¸ä¼¼åº¦è¯å«
- 项ç®å°å: https://www.kaggle.com/c/quora-question-pairs
- è§£å³æ¹æ¡: word2vec + Bi-GRU
ææ¬çº é
- bi-gram + levenshtein
3.å¾ååå¹ï¼Image Captioningï¼
mageå广¯ä¸ºç»å®å¾åçæææ¬æè¿°çä»»å¡ã
ä¸é¢æ¯ä¸äºå¾å¥½çåå¦è å¾åå广°æ®éã
- ä¸ä¸æä¸çå ¬å ±å¯¹è±¡ï¼COCOï¼ãå å«è¶ è¿12ä¸å¼ 带æè¿°çå¾åçéå
- Flickr 8Kãä»flickr.comè·åç8å个æè¿°å¾åçéåã
- Flickr 30Kãä»flickr.comè·åç3ä¸ä¸ªæè¿°å¾åçéåã æ¬²äºè§£æ´å¤ï¼è¯·çå¸å:
æ¢ç´¢å¾åå广°æ®éï¼2016å¹´
4.æºå¨ç¿»è¯ï¼Machine Translationï¼
æºå¨ç¿»è¯æ¯å°ææ¬ä»ä¸ç§è¯è¨ç¿»è¯æå¦ä¸ç§è¯è¨çä»»å¡ã
ä¸é¢æ¯ä¸äºå¾å¥½çåå¦è æºå¨ç¿»è¯æ°æ®éã
- å æ¿å¤§ç¬¬36å±è®®ä¼çåè°å½ä¼è®®åãæå¯¹çè±è¯åæ³è¯å¥åã
- 欧洲议ä¼è¯è®¼å¹³è¡è¯æåº1996-2011ãå¥å对ä¸å¥æ¬§æ´²è¯è¨ã æå¤§éæ åæ°æ®éç¨äºå¹´åº¦æºå¨ç¿»è¯ææ; çå°:
æºå¨ç¿»è¯
- Encoder + Decoder(Attention)
- åèæ¡ä¾: http://pytorch.apachecn.org/cn/tutorials/intermediate/seq2seq_translation_tutorial.html
5.é®çç³»ç»ï¼Question Answeringï¼
é®çæ¯ä¸é¡¹ä»»å¡ï¼å ¶ä¸æä¾äºä¸ä¸ªå¥åæææ¬æ ·æ¬ï¼ä»ä¸æåºé®é¢å¹¶ä¸å¿ é¡»åçé®é¢ã
ä¸é¢æ¯ä¸äºå¾å¥½çåå¦è é®é¢åçæ°æ®éã
- æ¯å¦ç¦é®é¢åçæ°æ®éï¼SQuADï¼ãåçæå ³ç»´åºç¾ç§æç« çé®é¢ã
- Deepmindé®é¢åçè¯æåºã仿¯æ¥é®æ¥åçæå ³æ°é»æç« çé®é¢ã
- äºé©¬éé®çæ°æ®ãåçæå ³äºé©¬é产åçé®é¢ã æå ³æ´å¤ä¿¡æ¯ï¼è¯·åé å¸å:
6.è¯é³è¯å«ï¼Speech Recognitionï¼
è¯é³è¯å«æ¯å°å£è¯çé³é¢è½¬æ¢ä¸ºäººç±»å¯è¯»ææ¬çä»»å¡ã
ä¸é¢æ¯ä¸äºå¾å¥½çåå¦è è¯é³è¯å«æ°æ®éã
- TIMITå£°å¦ - è¯é³è¿ç»è¯é³è¯æåºã䏿¯å è´¹çï¼ä½å å ¶å¹¿æ³ä½¿ç¨èä¸å¸ãå£è¯ç¾å½è±è¯åç¸å ³ç转å½ã
- VoxForgeãç¨äºæå»ºç¨äºè¯é³è¯å«ç弿ºæ°æ®åºç项ç®ã
- LibriSpeech ASRè¯æåºãä»LibriVoxæ¶éç大éè±è¯æå£°è¯»ç©ã
7.èªå¨ææï¼Document Summarizationï¼
ææ¡£æè¦æ¯å建è¾å¤§ææ¡£çç®çææä¹æè¿°çä»»å¡ã
ä¸é¢æ¯ä¸äºå¾å¥½çåå¦è ææ¡£æè¦æ°æ®éã
- æ³å¾æ¡ä¾æ¥åæ°æ®éãæ¶éäº4000份æ³å¾æ¡ä»¶åå ¶æè¦ã
- TIPSTERææ¬æè¦è¯ä¼°ä¼è®®è¯æåºãæ¶éäºè¿200份æä»¶åå ¶æè¦ã
- è±è¯æ°é»ææ¬çAQUAINTè¯æåºã䏿¯å è´¹çï¼èæ¯å¹¿æ³ä½¿ç¨çãæ°é»æç« çè¯æåºã 欲äºè§£æ´å¤ä¿¡æ¯:
ææ¡£çè§£ä¼è®®ï¼DUCï¼ä»»å¡ã å¨åªéå¯ä»¥æ¾å°ç¨äºææ¬æè¦çè¯å¥½æ°æ®éï¼
å½åå®ä½è¯å«
- Bi-LSTM CRF
- åèæ¡ä¾: http://pytorch.apachecn.org/cn/tutorials/beginner/nlp/advanced_tutorial.html
- CRFæ¨èææ¡£: https://www.jianshu.com/p/55755fc649b1
ææ¬æè¦
- æ½åå¼
- word2vec + textrank
- word2vecæ¨èææ¡£: https://www.zhihu.com/question/44832436/answer/266068967
- textrankæ¨èææ¡£: https://blog.csdn.net/BaiHuaXiu123/article/details/77847232
Graphå¾è®¡ç®ãæ ¢æ ¢æ´æ°ã
- æ°æ®é: https://github.com/apachecn/data/tree/master/graph
- å¦ä¹ èµæ: spark graphX宿.pdf ãæä»¶å¤ªå¤§ä¸æ¹ä¾¿æä¾ï¼èªå·±ç¾åº¦ã
ç¥è¯å¾è°±
- ç¥è¯å¾è°±ï¼æåªè®¤ SimmerChan: ãç¥è¯å¾è°±-ç»AIè£ ä¸ªå¤§èã
- 说å®è¯ï¼ææ¯çè¿å主èå¥åçå客é¿å¤§çï¼åçççæ¯æ·±å ¥æµ åºãæå¾åæ¬¢ï¼æä»¥å°±å享ç»å¤§å®¶ï¼å¸æä½ 们ä¹å欢ã
è¿ä¸æ¥é 读
妿æ¨å¸ææ´æ·±å ¥ï¼æ¬èæä¾äºå ¶ä»æ°æ®éå表ã
- ç»´åºç¾ç§ç ç©¶ä¸ä½¿ç¨çææ¬æ°æ®é
- æ°æ®é: 计ç®è¯è¨å¦å®¶åèªç¶è¯è¨å¤çç 究人å使ç¨çä¸»è¦ææ¬è¯æåºæ¯ä»ä¹ï¼
- æ¯å¦ç¦ç»è®¡èªç¶è¯è¨å¤çè¯æåº
- æåæ¯é¡ºåºæåçNLPæ°æ®éå表
- è¯¥æºæNLTK
- å¨DL4J䏿弿·±åº¦å¦ä¹ æ°æ®
- NLPæ°æ®é
- å½å 弿¾æ°æ®é: https://bosonnlp.com/dev/resource
åè
è´è°¢
æè¿æ ææ¶å°ç¾¤åæ¨éç龿¥ï¼åç°å¾å°å¤§ä½¬é«åº¦ç认å¯ï¼å¹¶å¨çå¿çæ¨å¹¿ã卿¤æè°¢:
èµå©æä»¬
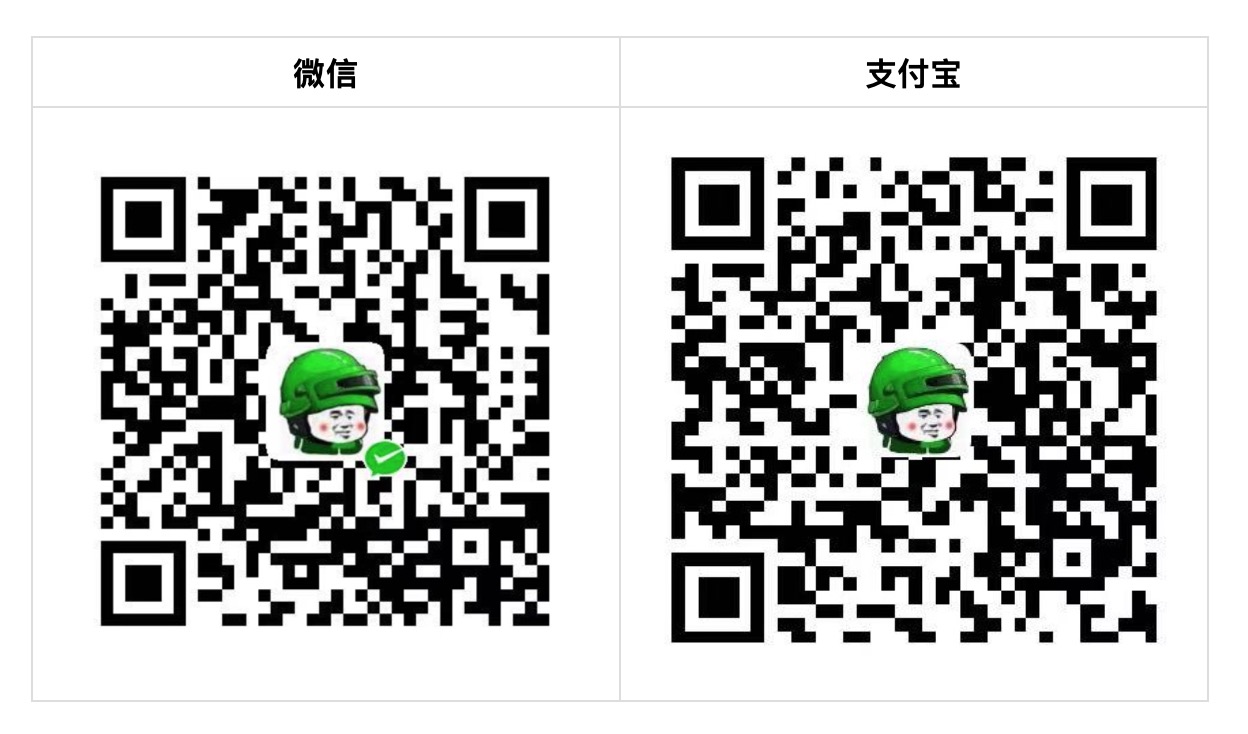
Top Related Projects
12 weeks, 26 lessons, 52 quizzes, classic Machine Learning for all
⛔️ DEPRECATED – See https://github.com/ageron/handson-ml3 instead.
The "Python Machine Learning (1st edition)" book code repository and info resource
TensorFlow Tutorial and Examples for Beginners (support TF v1 & v2)
Machine Learning From Scratch. Bare bones NumPy implementations of machine learning models and algorithms with a focus on accessibility. Aims to cover everything from linear regression to deep learning.
📺 Discover the latest machine learning / AI courses on YouTube.
Convert
designs to code with AI
Introducing Visual Copilot: A new AI model to turn Figma designs to high quality code using your components.
Try Visual Copilot