dopamine
Dopamine is a research framework for fast prototyping of reinforcement learning algorithms.
Top Related Projects
OpenAI Baselines: high-quality implementations of reinforcement learning algorithms
A fork of OpenAI Baselines, implementations of reinforcement learning algorithms
TF-Agents: A reliable, scalable and easy to use TensorFlow library for Contextual Bandits and Reinforcement Learning.
A library of reinforcement learning components and agents
A modular, primitive-first, python-first PyTorch library for Reinforcement Learning.
Quick Overview
Dopamine is an open-source research framework for fast prototyping of reinforcement learning algorithms developed by Google. It aims to make RL research more accessible and reproducible by providing a flexible, scalable, and well-tested framework for implementing and evaluating RL agents.
Pros
- Easy to use and extend, with a modular design that allows for quick experimentation
- Implements several popular RL algorithms out of the box (e.g., DQN, Rainbow, C51)
- Integrates well with TensorFlow and Gin-config for flexible configuration
- Provides tools for visualization and analysis of agent performance
Cons
- Limited to discrete action spaces, which may not be suitable for all RL problems
- Primarily focused on value-based methods, with less support for policy gradient algorithms
- May have a steeper learning curve for those unfamiliar with TensorFlow or Gin-config
- Documentation could be more comprehensive for advanced usage scenarios
Code Examples
- Creating a DQN agent:
import dopamine.agents.dqn.dqn_agent as dqn_agent
import dopamine.discrete_domains.gym_lib as gym_lib
environment = gym_lib.create_gym_environment('Pong')
agent = dqn_agent.DQNAgent(
num_actions=environment.action_space.n,
observation_shape=environment.observation_space.shape,
stack_size=4)
- Running a training iteration:
initial_observation = environment.reset()
action = agent.begin_episode(initial_observation)
for _ in range(max_steps_per_episode):
observation, reward, done, _ = environment.step(action)
action = agent.step(reward, observation)
if done:
break
agent.end_episode(reward)
- Evaluating agent performance:
from dopamine.metrics import statistics_instance
statistics = statistics_instance.StatisticsInstance('eval')
for _ in range(num_eval_episodes):
statistics.episode()
# Run evaluation episode
statistics.add_episode_reward(episode_reward)
average_reward = statistics.get_average_reward()
Getting Started
To get started with Dopamine:
- Install Dopamine:
pip install dopamine-rl
- Create a simple DQN agent and run it on a Gym environment:
import dopamine.discrete_domains.run_experiment as run_experiment
import dopamine.agents.dqn.dqn_agent as dqn_agent
import gin
gin.parse_config_file('dopamine/agents/dqn/configs/dqn.gin')
runner = run_experiment.Runner(
base_dir='/tmp/dopamine_runs',
create_agent_fn=dqn_agent.DQNAgent)
runner.run_experiment()
This will train a DQN agent on the default Atari environment (Pong) using the configurations specified in the gin file.
Competitor Comparisons
OpenAI Baselines: high-quality implementations of reinforcement learning algorithms
Pros of Baselines
- Wider range of algorithms implemented, including PPO, TRPO, and DDPG
- More extensive documentation and examples for various environments
- Active community support and regular updates
Cons of Baselines
- Steeper learning curve for beginners
- Less focus on visualization tools compared to Dopamine
- Some implementations may be less optimized for performance
Code Comparison
Baselines (PPO implementation):
def learn(network, env, total_timesteps, **network_kwargs):
policy = build_policy(env, network, **network_kwargs)
# PPO-specific parameters
nminibatches = 4
noptepochs = 4
model = PPO2(policy=policy, env=env, nminibatches=nminibatches, noptepochs=noptepochs)
model.learn(total_timesteps=total_timesteps)
Dopamine (DQN implementation):
def create_agent(sess, environment, summary_writer=None):
return dqn_agent.DQNAgent(
sess,
num_actions=environment.action_space.n,
observation_shape=environment.observation_space.shape,
summary_writer=summary_writer)
runner = Runner(base_dir, create_agent, game_name)
runner.run()
A fork of OpenAI Baselines, implementations of reinforcement learning algorithms
Pros of Stable-Baselines
- Wider range of algorithms implemented, including PPO, SAC, and DDPG
- More extensive documentation and tutorials for beginners
- Active community and frequent updates
Cons of Stable-Baselines
- Less focus on research-oriented features compared to Dopamine
- May have slightly higher computational overhead due to its comprehensive nature
Code Comparison
Dopamine (loading an agent):
agent = dopamine.agents.dqn.dqn_agent.DQNAgent(
num_actions=environment.action_space.n,
observation_shape=environment.observation_space.shape,
observation_dtype=environment.observation_space.dtype,
stack_size=config.stack_size,
network=atari_lib.nature_dqn_network)
Stable-Baselines (loading an agent):
from stable_baselines3 import DQN
model = DQN("MlpPolicy", "CartPole-v1", verbose=1)
model.learn(total_timesteps=10000)
Both libraries offer easy-to-use interfaces for reinforcement learning, but Stable-Baselines provides a more streamlined API for quick experimentation, while Dopamine offers more flexibility for custom implementations and research-oriented projects.
TF-Agents: A reliable, scalable and easy to use TensorFlow library for Contextual Bandits and Reinforcement Learning.
Pros of TensorFlow Agents
- More comprehensive and feature-rich, offering a wider range of RL algorithms
- Better integration with the TensorFlow ecosystem
- More active development and community support
Cons of TensorFlow Agents
- Steeper learning curve due to its complexity
- Potentially slower execution compared to Dopamine's focused approach
Code Comparison
Dopamine (simple DQN implementation):
agent = dqn_agent.DQNAgent(
num_actions=num_actions,
observation_shape=observation_shape,
observation_dtype=tf.float32,
stack_size=stack_size,
network=atari_lib.NatureDQNNetwork)
TensorFlow Agents (DQN implementation):
agent = dqn_agent.DqnAgent(
time_step_spec,
action_spec,
q_network=q_net,
optimizer=optimizer,
td_errors_loss_fn=common.element_wise_squared_loss,
train_step_counter=train_step_counter)
Both repositories focus on reinforcement learning, but TensorFlow Agents offers a more comprehensive toolkit with broader algorithm support. Dopamine, on the other hand, provides a simpler, more focused approach to RL research. TensorFlow Agents integrates better with the TensorFlow ecosystem, while Dopamine may be easier to get started with for beginners. The code comparison shows that TensorFlow Agents requires more setup but offers more flexibility in configuration.
A library of reinforcement learning components and agents
Pros of Acme
- More comprehensive and flexible framework for RL research
- Supports a wider range of algorithms and environments
- Better suited for distributed and large-scale experiments
Cons of Acme
- Steeper learning curve due to increased complexity
- Potentially overkill for simpler RL tasks
- Less focus on visualization tools compared to Dopamine
Code Comparison
Dopamine (agent creation):
agent = rainbow_agent.RainbowAgent(
num_actions=environment.action_space.n,
observation_shape=environment.observation_space.shape,
observation_dtype=environment.observation_space.dtype)
Acme (agent creation):
agent = sac.SACAgent(
environment_spec=environment_spec,
policy_network=policy_network,
critic_network=critic_network,
target_entropy=target_entropy)
Both repositories provide frameworks for reinforcement learning research, but Acme offers a more extensive and flexible approach. Dopamine focuses on simplicity and reproducibility, making it easier for beginners to get started. Acme, on the other hand, provides a broader range of tools and algorithms, making it more suitable for advanced research and large-scale experiments. The code comparison shows that Acme requires more setup but offers greater customization, while Dopamine provides a more straightforward approach to agent creation.
Pros of rlax
- More flexible and modular design, allowing for easier customization of RL algorithms
- Better integration with JAX, enabling efficient GPU/TPU acceleration
- Broader range of RL algorithms and components available
Cons of rlax
- Steeper learning curve due to its more low-level nature
- Less comprehensive documentation and tutorials compared to Dopamine
- Requires more boilerplate code to set up complete RL experiments
Code Comparison
rlax example:
import jax
import rlax
def loss_fn(params, target, prediction):
return rlax.l2_loss(prediction, target)
grad_fn = jax.grad(loss_fn)
Dopamine example:
import dopamine.jax.agents.dqn.dqn_agent as dqn_agent
agent = dqn_agent.JaxDQNAgent(
num_actions=4,
observation_shape=(84, 84, 4),
stack_size=4
)
rlax offers more granular control over RL components, while Dopamine provides higher-level abstractions for complete agents. rlax's integration with JAX allows for easy gradient computation, whereas Dopamine focuses on providing ready-to-use agent implementations.
A modular, primitive-first, python-first PyTorch library for Reinforcement Learning.
Pros of RL
- Built on PyTorch, offering more flexibility and easier integration with other deep learning projects
- Supports a wider range of RL algorithms, including policy gradient methods and model-based RL
- More active community and frequent updates
Cons of RL
- Less focus on reproducibility compared to Dopamine
- May have a steeper learning curve for beginners due to its broader scope
Code Comparison
RL (PyTorch):
import torch
from torch import nn
from torch.distributions import Categorical
class Policy(nn.Module):
def __init__(self):
super(Policy, self).__init__()
self.affine1 = nn.Linear(4, 128)
self.action_head = nn.Linear(128, 2)
Dopamine (TensorFlow):
import tensorflow as tf
def create_agent(sess, environment, summary_writer=None):
return dqn_agent.DQNAgent(
sess,
num_actions=environment.action_space.n,
observation_shape=environment.observation_space.shape,
summary_writer=summary_writer)
The code snippets showcase the different approaches: RL uses PyTorch's object-oriented style, while Dopamine relies on TensorFlow's functional approach. RL's example demonstrates defining a policy network, whereas Dopamine's shows agent creation.
Convert
designs to code with AI
Introducing Visual Copilot: A new AI model to turn Figma designs to high quality code using your components.
Try Visual CopilotREADME
Dopamine
Getting Started | Docs | Baseline Results | Changelist
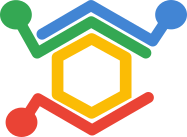
Dopamine is a research framework for fast prototyping of reinforcement learning algorithms. It aims to fill the need for a small, easily grokked codebase in which users can freely experiment with wild ideas (speculative research).
Our design principles are:
- Easy experimentation: Make it easy for new users to run benchmark experiments.
- Flexible development: Make it easy for new users to try out research ideas.
- Compact and reliable: Provide implementations for a few, battle-tested algorithms.
- Reproducible: Facilitate reproducibility in results. In particular, our setup follows the recommendations given by Machado et al. (2018).
Dopamine supports the following agents, implemented with jax:
- DQN (Mnih et al., 2015)
- C51 (Bellemare et al., 2017)
- Rainbow (Hessel et al., 2018)
- IQN (Dabney et al., 2018)
- SAC (Haarnoja et al., 2018)
For more information on the available agents, see the docs.
Many of these agents also have a tensorflow (legacy) implementation, though newly added agents are likely to be jax-only.
This is not an official Google product.
Getting Started
We provide docker containers for using Dopamine. Instructions can be found here.
Alternatively, Dopamine can be installed from source (preferred) or installed with pip. For either of these methods, continue reading at prerequisites.
Prerequisites
Dopamine supports Atari environments and Mujoco environments. Install the environments you intend to use before you install Dopamine:
Atari
- Install the atari roms following the instructions from atari-py.
pip install ale-py
(we recommend using a virtual environment):unzip $ROM_DIR/ROMS.zip -d $ROM_DIR && ale-import-roms $ROM_DIR/ROMS
(replace $ROM_DIR with the directory you extracted the ROMs to).
Mujoco
- Install Mujoco and get a license here.
- Run
pip install mujoco-py
(we recommend using a virtual environment).
Installing from Source
The most common way to use Dopamine is to install it from source and modify the source code directly:
git clone https://github.com/google/dopamine
After cloning, install dependencies:
pip install -r dopamine/requirements.txt
Dopamine supports tensorflow (legacy) and jax (actively maintained) agents. View the Tensorflow documentation for more information on installing tensorflow.
Note: We recommend using a virtual environment when working with Dopamine.
Installing with Pip
Note: We strongly recommend installing from source for most users.
Installing with pip is simple, but Dopamine is designed to be modified directly. We recommend installing from source for writing your own experiments.
pip install dopamine-rl
Running tests
You can test whether the installation was successful by running the following from the dopamine root directory.
export PYTHONPATH=$PYTHONPATH:$PWD
python -m tests.dopamine.atari_init_test
Next Steps
View the docs for more information on training agents.
We supply baselines for each Dopamine agent.
We also provide a set of Colaboratory notebooks which demonstrate how to use Dopamine.
References
Mnih et al., Human-level Control through Deep Reinforcement Learning. Nature, 2015.
Giving credit
If you use Dopamine in your work, we ask that you cite our white paper. Here is an example BibTeX entry:
@article{castro18dopamine,
author = {Pablo Samuel Castro and
Subhodeep Moitra and
Carles Gelada and
Saurabh Kumar and
Marc G. Bellemare},
title = {Dopamine: {A} {R}esearch {F}ramework for {D}eep {R}einforcement {L}earning},
year = {2018},
url = {http://arxiv.org/abs/1812.06110},
archivePrefix = {arXiv}
}
Top Related Projects
OpenAI Baselines: high-quality implementations of reinforcement learning algorithms
A fork of OpenAI Baselines, implementations of reinforcement learning algorithms
TF-Agents: A reliable, scalable and easy to use TensorFlow library for Contextual Bandits and Reinforcement Learning.
A library of reinforcement learning components and agents
A modular, primitive-first, python-first PyTorch library for Reinforcement Learning.
Convert
designs to code with AI
Introducing Visual Copilot: A new AI model to turn Figma designs to high quality code using your components.
Try Visual Copilot