autogen
A programming framework for agentic AI 🤖 PyPi: autogen-agentchat Discord: https://aka.ms/autogen-discord Office Hour: https://aka.ms/autogen-officehour
Top Related Projects
Examples and guides for using the OpenAI API
Integrate cutting-edge LLM technology quickly and easily into your apps
🦜🔗 Build context-aware reasoning applications
🤗 Transformers: State-of-the-art Machine Learning for Pytorch, TensorFlow, and JAX.
Quick Overview
Microsoft AutoGen is an open-source framework for building Large Language Model (LLM) applications using multiple agents. It enables the creation of conversational AI systems where multiple AI agents can collaborate, solve problems, and perform tasks together. AutoGen simplifies the development of complex AI applications by providing a flexible and extensible architecture for multi-agent interactions.
Pros
- Enables the creation of sophisticated multi-agent AI systems
- Provides a flexible and extensible framework for LLM applications
- Supports various LLM backends, including OpenAI GPT models and local models
- Offers built-in tools for task planning, execution, and error handling
Cons
- Requires a good understanding of LLMs and multi-agent systems
- May have a steeper learning curve compared to simpler chatbot frameworks
- Documentation could be more comprehensive for advanced use cases
- Performance may vary depending on the chosen LLM backend
Code Examples
- Creating a simple conversation between two agents:
from autogen import AssistantAgent, UserProxyAgent, ConversationChain
assistant = AssistantAgent("assistant")
user_proxy = UserProxyAgent("user_proxy")
conversation = ConversationChain(agents=[user_proxy, assistant])
conversation.initiate("Tell me a joke about programming.")
- Using a function-calling agent to perform a specific task:
from autogen import AssistantAgent, FunctionCallingAgent
def calculate_sum(a, b):
return a + b
assistant = AssistantAgent("assistant")
function_agent = FunctionCallingAgent("function_agent", functions=[calculate_sum])
result = function_agent.run("Calculate the sum of 5 and 7")
print(result) # Output: 12
- Creating a group chat with multiple agents:
from autogen import AssistantAgent, UserProxyAgent, GroupChat, GroupChatManager
assistant1 = AssistantAgent("assistant1")
assistant2 = AssistantAgent("assistant2")
user_proxy = UserProxyAgent("user_proxy")
group_chat = GroupChat(agents=[user_proxy, assistant1, assistant2])
manager = GroupChatManager(group_chat=group_chat)
manager.initiate("Discuss the pros and cons of using Python for web development.")
Getting Started
To get started with Microsoft AutoGen, follow these steps:
- Install the library:
pip install pyautogen
- Set up your OpenAI API key (if using OpenAI models):
import os
os.environ["OPENAI_API_KEY"] = "your-api-key-here"
- Create a simple conversation:
from autogen import AssistantAgent, UserProxyAgent, ConversationChain
assistant = AssistantAgent("assistant")
user_proxy = UserProxyAgent("user_proxy")
conversation = ConversationChain(agents=[user_proxy, assistant])
conversation.initiate("Hello, how can you help me today?")
This will start a basic conversation between a user proxy and an AI assistant. You can expand on this example to create more complex multi-agent systems and interactions.
Competitor Comparisons
Examples and guides for using the OpenAI API
Pros of OpenAI Cookbook
- Extensive collection of practical examples and tutorials for using OpenAI's APIs
- Covers a wide range of use cases and applications, from basic to advanced
- Regularly updated with new examples and best practices
Cons of OpenAI Cookbook
- Focused solely on OpenAI's offerings, limiting its scope compared to AutoGen
- Less emphasis on multi-agent systems and complex interactions
- Primarily code snippets and examples rather than a full framework
Code Comparison
OpenAI Cookbook example (basic API call):
import openai
response = openai.Completion.create(
model="text-davinci-002",
prompt="Translate the following English text to French: '{}'",
max_tokens=60
)
AutoGen example (multi-agent conversation):
from autogen import AssistantAgent, UserProxyAgent, ConversableAgent
assistant = AssistantAgent("assistant")
user_proxy = UserProxyAgent("user_proxy")
user_proxy.initiate_chat(assistant, message="Translate 'Hello' to French")
The OpenAI Cookbook provides straightforward examples for API usage, while AutoGen focuses on creating and managing multi-agent systems for more complex interactions and tasks.
Integrate cutting-edge LLM technology quickly and easily into your apps
Pros of Semantic Kernel
- More focused on integrating AI capabilities into existing applications
- Provides a structured approach to building AI-powered skills and functions
- Offers better support for memory and context management in conversations
Cons of Semantic Kernel
- Less emphasis on multi-agent interactions and autonomous task completion
- May require more setup and configuration for complex AI-driven workflows
- Limited support for dynamic code generation and execution
Code Comparison
Semantic Kernel:
var kernel = Kernel.Builder.Build();
var skill = kernel.ImportSkill(new TextSkill());
var result = await kernel.RunAsync("Hello world!", skill["Uppercase"]);
AutoGen:
human_proxy = autogen.UserProxyAgent(name="Human")
assistant = autogen.AssistantAgent(name="AI")
task = "Write a hello world program"
human_proxy.initiate_chat(assistant, message=task)
Summary
Semantic Kernel excels in integrating AI into existing applications with structured skills and memory management. AutoGen focuses more on multi-agent interactions and autonomous task completion. The choice between them depends on the specific requirements of your project and the level of AI integration needed.
🦜🔗 Build context-aware reasoning applications
Pros of LangChain
- More extensive documentation and tutorials
- Larger community and ecosystem of integrations
- Flexible architecture for building complex AI applications
Cons of LangChain
- Steeper learning curve for beginners
- Can be overwhelming with numerous components and options
- Less focus on multi-agent systems compared to AutoGen
Code Comparison
LangChain example:
from langchain import OpenAI, LLMChain, PromptTemplate
llm = OpenAI(temperature=0.9)
prompt = PromptTemplate(
input_variables=["product"],
template="What is a good name for a company that makes {product}?",
)
chain = LLMChain(llm=llm, prompt=prompt)
print(chain.run("colorful socks"))
AutoGen example:
from autogen import AssistantAgent, UserProxyAgent, config_list_from_json
assistant = AssistantAgent("assistant", llm_config={"config_list": config_list_from_json("OAI_CONFIG_LIST")})
user_proxy = UserProxyAgent("user_proxy", code_execution_config={"work_dir": "coding"})
user_proxy.initiate_chat(assistant, message="Write a Python function to calculate the factorial of a number.")
Both LangChain and AutoGen offer powerful tools for building AI applications, but they have different strengths. LangChain excels in flexibility and ecosystem support, while AutoGen focuses on multi-agent systems and ease of use for specific scenarios.
🤗 Transformers: State-of-the-art Machine Learning for Pytorch, TensorFlow, and JAX.
Pros of Transformers
- Extensive library of pre-trained models for various NLP tasks
- Well-documented and widely adopted in the AI/ML community
- Supports multiple deep learning frameworks (PyTorch, TensorFlow)
Cons of Transformers
- Focused primarily on NLP tasks, less versatile for general AI applications
- Steeper learning curve for beginners compared to AutoGen's high-level API
- Requires more manual configuration for complex multi-model interactions
Code Comparison
Transformers:
from transformers import pipeline
classifier = pipeline("sentiment-analysis")
result = classifier("I love this product!")[0]
print(f"Label: {result['label']}, Score: {result['score']:.4f}")
AutoGen:
from autogen import AssistantAgent, UserProxyAgent
assistant = AssistantAgent("assistant")
user_proxy = UserProxyAgent("user_proxy")
user_proxy.initiate_chat(assistant, message="Analyze the sentiment: I love this product!")
Summary
Transformers excels in providing a comprehensive toolkit for NLP tasks with a wide range of pre-trained models. AutoGen, on the other hand, offers a more accessible approach to building conversational AI systems and multi-agent workflows. While Transformers provides granular control over model operations, AutoGen simplifies the process of creating interactive AI agents for various applications.
Convert
designs to code with AI
Introducing Visual Copilot: A new AI model to turn Figma designs to high quality code using your components.
Try Visual CopilotREADME
AutoGen
AutoGen is a framework for creating multi-agent AI applications that can act autonomously or work alongside humans.
Installation
AutoGen requires Python 3.10 or later.
# Install AgentChat and OpenAI client from Extensions
pip install -U "autogen-agentchat" "autogen-ext[openai]"
The current stable version is v0.4. If you are upgrading from AutoGen v0.2, please refer to the Migration Guide for detailed instructions on how to update your code and configurations.
# Install AutoGen Studio for no-code GUI
pip install -U "autogenstudio"
Quickstart
Hello World
Create an assistant agent using OpenAI's GPT-4o model. See other supported models.
import asyncio
from autogen_agentchat.agents import AssistantAgent
from autogen_ext.models.openai import OpenAIChatCompletionClient
async def main() -> None:
model_client = OpenAIChatCompletionClient(model="gpt-4o")
agent = AssistantAgent("assistant", model_client=model_client)
print(await agent.run(task="Say 'Hello World!'"))
await model_client.close()
asyncio.run(main())
Web Browsing Agent Team
Create a group chat team with a web surfer agent and a user proxy agent for web browsing tasks. You need to install playwright.
# pip install -U autogen-agentchat autogen-ext[openai,web-surfer]
# playwright install
import asyncio
from autogen_agentchat.agents import UserProxyAgent
from autogen_agentchat.conditions import TextMentionTermination
from autogen_agentchat.teams import RoundRobinGroupChat
from autogen_agentchat.ui import Console
from autogen_ext.models.openai import OpenAIChatCompletionClient
from autogen_ext.agents.web_surfer import MultimodalWebSurfer
async def main() -> None:
model_client = OpenAIChatCompletionClient(model="gpt-4o")
# The web surfer will open a Chromium browser window to perform web browsing tasks.
web_surfer = MultimodalWebSurfer("web_surfer", model_client, headless=False, animate_actions=True)
# The user proxy agent is used to get user input after each step of the web surfer.
# NOTE: you can skip input by pressing Enter.
user_proxy = UserProxyAgent("user_proxy")
# The termination condition is set to end the conversation when the user types 'exit'.
termination = TextMentionTermination("exit", sources=["user_proxy"])
# Web surfer and user proxy take turns in a round-robin fashion.
team = RoundRobinGroupChat([web_surfer, user_proxy], termination_condition=termination)
try:
# Start the team and wait for it to terminate.
await Console(team.run_stream(task="Find information about AutoGen and write a short summary."))
finally:
await web_surfer.close()
await model_client.close()
asyncio.run(main())
AutoGen Studio
Use AutoGen Studio to prototype and run multi-agent workflows without writing code.
# Run AutoGen Studio on http://localhost:8080
autogenstudio ui --port 8080 --appdir ./my-app
Why Use AutoGen?

The AutoGen ecosystem provides everything you need to create AI agents, especially multi-agent workflows -- framework, developer tools, and applications.
The framework uses a layered and extensible design. Layers have clearly divided responsibilities and build on top of layers below. This design enables you to use the framework at different levels of abstraction, from high-level APIs to low-level components.
- Core API implements message passing, event-driven agents, and local and distributed runtime for flexibility and power. It also support cross-language support for .NET and Python.
- AgentChat API implements a simpler but opinionated API for rapid prototyping. This API is built on top of the Core API and is closest to what users of v0.2 are familiar with and supports common multi-agent patterns such as two-agent chat or group chats.
- Extensions API enables first- and third-party extensions continuously expanding framework capabilities. It support specific implementation of LLM clients (e.g., OpenAI, AzureOpenAI), and capabilities such as code execution.
The ecosystem also supports two essential developer tools:
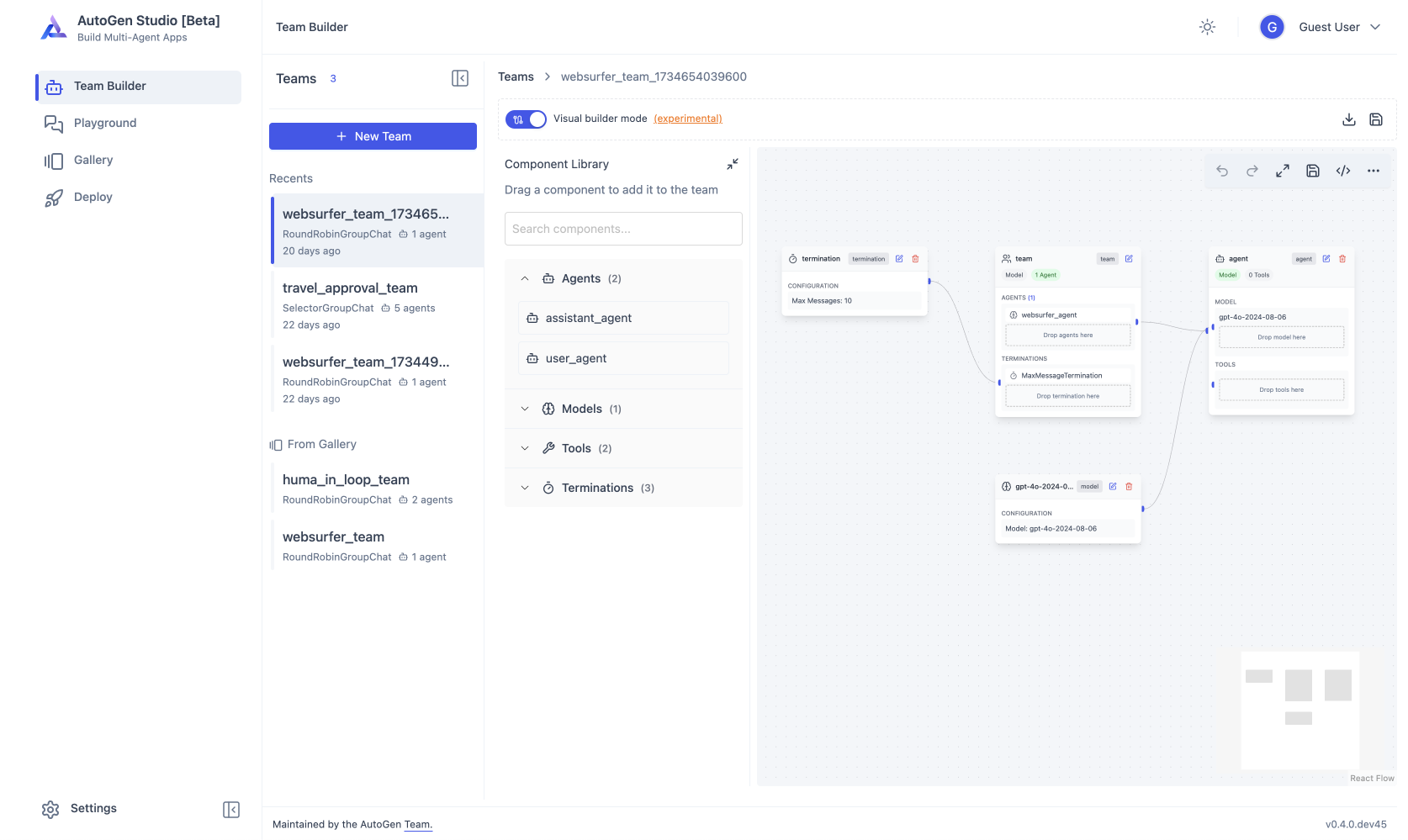
- AutoGen Studio provides a no-code GUI for building multi-agent applications.
- AutoGen Bench provides a benchmarking suite for evaluating agent performance.
You can use the AutoGen framework and developer tools to create applications for your domain. For example, Magentic-One is a state-of-the-art multi-agent team built using AgentChat API and Extensions API that can handle a variety of tasks that require web browsing, code execution, and file handling.
With AutoGen you get to join and contribute to a thriving ecosystem. We host weekly office hours and talks with maintainers and community. We also have a Discord server for real-time chat, GitHub Discussions for Q&A, and a blog for tutorials and updates.
Where to go next?
Interested in contributing? See CONTRIBUTING.md for guidelines on how to get started. We welcome contributions of all kinds, including bug fixes, new features, and documentation improvements. Join our community and help us make AutoGen better!
Have questions? Check out our Frequently Asked Questions (FAQ) for answers to common queries. If you don't find what you're looking for, feel free to ask in our GitHub Discussions or join our Discord server for real-time support. You can also read our blog for updates.
Legal Notices
Microsoft and any contributors grant you a license to the Microsoft documentation and other content in this repository under the Creative Commons Attribution 4.0 International Public License, see the LICENSE file, and grant you a license to any code in the repository under the MIT License, see the LICENSE-CODE file.
Microsoft, Windows, Microsoft Azure, and/or other Microsoft products and services referenced in the documentation may be either trademarks or registered trademarks of Microsoft in the United States and/or other countries. The licenses for this project do not grant you rights to use any Microsoft names, logos, or trademarks. Microsoft's general trademark guidelines can be found at http://go.microsoft.com/fwlink/?LinkID=254653.
Privacy information can be found at https://go.microsoft.com/fwlink/?LinkId=521839
Microsoft and any contributors reserve all other rights, whether under their respective copyrights, patents, or trademarks, whether by implication, estoppel, or otherwise.
Top Related Projects
Examples and guides for using the OpenAI API
Integrate cutting-edge LLM technology quickly and easily into your apps
🦜🔗 Build context-aware reasoning applications
🤗 Transformers: State-of-the-art Machine Learning for Pytorch, TensorFlow, and JAX.
Convert
designs to code with AI
Introducing Visual Copilot: A new AI model to turn Figma designs to high quality code using your components.
Try Visual Copilot