Top Related Projects
Unsupervised text tokenizer for Neural Network-based text generation.
💥 Fast State-of-the-Art Tokenizers optimized for Research and Production
💫 Industrial-strength Natural Language Processing (NLP) in Python
NLTK Source
Topic Modelling for Humans
An open-source NLP research library, built on PyTorch.
Quick Overview
The tensorflow/text
repository is a collection of text processing and text generation models and utilities built on top of TensorFlow. It provides a set of high-level APIs for common natural language processing (NLP) tasks, such as text classification, named entity recognition, and text generation.
Pros
- Comprehensive NLP Toolkit: The repository offers a wide range of NLP tools and models, making it a one-stop-shop for many common text processing tasks.
- Integration with TensorFlow: As a part of the TensorFlow ecosystem,
tensorflow/text
seamlessly integrates with other TensorFlow libraries, allowing for end-to-end deep learning pipelines. - Actively Maintained: The project is actively maintained by the TensorFlow team, ensuring regular updates and bug fixes.
- Extensible and Customizable: The modular design of the library allows for easy customization and extension of the provided models and utilities.
Cons
- Steep Learning Curve: The library's extensive feature set and integration with the broader TensorFlow ecosystem can make it challenging for beginners to get started.
- Limited Documentation: While the project has good documentation, it may not be as comprehensive as some users would prefer, especially for more advanced use cases.
- Performance Overhead: The tight integration with TensorFlow can introduce some performance overhead compared to more lightweight text processing libraries.
- Dependency on TensorFlow: The project is tightly coupled with the TensorFlow library, which may be a drawback for users who prefer to work with other deep learning frameworks.
Code Examples
Here are a few code examples demonstrating the usage of tensorflow/text
:
Text Classification
import tensorflow as tf
import tensorflow_text as tf_text
# Load a pre-trained text classification model
model = tf.keras.models.load_model('path/to/model')
# Preprocess the input text
text = "This movie was amazing!"
input_text = tf_text.case_fold_utf8([text])
# Make a prediction
prediction = model.predict(input_text)
print(f"Predicted class: {prediction[0]}")
Named Entity Recognition
import tensorflow as tf
import tensorflow_text as tf_text
# Load a pre-trained NER model
model = tf.keras.models.load_model('path/to/ner_model')
# Preprocess the input text
text = "John Doe lives in New York City."
input_text = tf_text.case_fold_utf8([text])
# Make a prediction
prediction = model.predict(input_text)
print(f"Named entities: {prediction[0]}")
Text Generation
import tensorflow as tf
import tensorflow_text as tf_text
# Load a pre-trained text generation model
model = tf.keras.models.load_model('path/to/text_generation_model')
# Generate new text
seed_text = "Once upon a time, there was a"
generated_text = model.generate(seed_text, max_length=100)
print(f"Generated text: {generated_text[0]}")
Getting Started
To get started with tensorflow/text
, you can follow these steps:
-
Install the required dependencies:
pip install tensorflow tensorflow-text
-
Import the necessary modules:
import tensorflow as tf import tensorflow_text as tf_text
-
Load a pre-trained model or create a new model using the provided utilities:
# Load a pre-trained text classification model model = tf.keras.models.load_model('path/to/model') # Create a new text generation model model = tf.keras.Sequential([ tf.keras.layers.Embedding(input_dim=vocab_size, output_dim=embedding_dim), tf.keras.layers.LSTM(units=rnn_units), tf.keras.layers.Dense(vocab_size, activation='softmax') ])
-
Preprocess your input data using the provided text processing utilities:
# Preprocess the input text text = "This is a sample text." input_text = tf_text.case_fold_
Competitor Comparisons
Unsupervised text tokenizer for Neural Network-based text generation.
Pros of SentencePiece
- Language-agnostic tokenization, supporting a wide range of languages without pre-tokenization
- Smaller model size and faster tokenization speed
- Direct integration with popular NLP libraries like TensorFlow and PyTorch
Cons of SentencePiece
- Limited built-in text preprocessing capabilities compared to TensorFlow Text
- Fewer advanced features for complex text manipulation tasks
- Less seamless integration with the broader TensorFlow ecosystem
Code Comparison
SentencePiece:
import sentencepiece as spm
sp = spm.SentencePieceProcessor()
sp.load('model.model')
encoded = sp.encode('Hello, world!', out_type=int)
TensorFlow Text:
import tensorflow_text as text
tokenizer = text.WhitespaceTokenizer()
tokens = tokenizer.tokenize(['Hello, world!'])
Both libraries offer efficient tokenization, but SentencePiece focuses on subword tokenization, while TensorFlow Text provides a broader range of text processing tools. SentencePiece is more suitable for multilingual scenarios and when model size is a concern, whereas TensorFlow Text excels in complex text manipulation tasks within the TensorFlow ecosystem.
💥 Fast State-of-the-Art Tokenizers optimized for Research and Production
Pros of tokenizers
- Faster tokenization due to Rust implementation
- More flexible and customizable tokenization options
- Supports a wider range of pre-trained tokenizers
Cons of tokenizers
- Less integrated with TensorFlow ecosystem
- May require additional setup for use with TensorFlow models
- Smaller community compared to TensorFlow Text
Code Comparison
tokenizers:
from tokenizers import Tokenizer
from tokenizers.models import WordPiece
tokenizer = Tokenizer(WordPiece(unk_token="[UNK]"))
tokenizer.train(files=["path/to/files/*.txt"], vocab_size=30000)
output = tokenizer.encode("Hello, world!")
text:
import tensorflow_text as text
tokenizer = text.WhitespaceTokenizer()
tokens = tokenizer.tokenize(["Hello, world!"])
The tokenizers library offers more flexibility in tokenizer creation and training, while text provides simpler integration with TensorFlow but with fewer customization options. tokenizers excels in performance and adaptability, making it suitable for various NLP tasks. text, being part of the TensorFlow ecosystem, offers seamless integration with TensorFlow models and operations but may be less versatile for complex tokenization requirements.
💫 Industrial-strength Natural Language Processing (NLP) in Python
Pros of spaCy
- More comprehensive out-of-the-box NLP pipeline with pre-trained models
- Easier to use for non-deep learning tasks and general NLP workflows
- Faster processing speed for many common NLP tasks
Cons of spaCy
- Less flexibility for custom deep learning models
- Smaller community and ecosystem compared to TensorFlow
- Limited support for non-English languages compared to TensorFlow Text
Code Comparison
spaCy:
import spacy
nlp = spacy.load("en_core_web_sm")
doc = nlp("Apple is looking at buying U.K. startup for $1 billion")
for ent in doc.ents:
print(ent.text, ent.label_)
TensorFlow Text:
import tensorflow_text as text
tokenizer = text.WhitespaceTokenizer()
tokens = tokenizer.tokenize(['hello world', 'how are you'])
print(tokens.to_list())
The spaCy example demonstrates its ease of use for named entity recognition, while the TensorFlow Text example shows basic tokenization. spaCy provides a more complete NLP pipeline out-of-the-box, whereas TensorFlow Text offers lower-level text processing operations that can be integrated into TensorFlow models.
NLTK Source
Pros of NLTK
- Comprehensive library with a wide range of NLP tools and resources
- Excellent documentation and educational materials for learning NLP concepts
- Large community support and extensive corpus of pre-processed text data
Cons of NLTK
- Slower performance compared to TensorFlow Text, especially for large-scale tasks
- Less integration with modern deep learning frameworks
- Limited support for advanced neural network-based NLP models
Code Comparison
NLTK:
import nltk
from nltk.tokenize import word_tokenize
from nltk.tag import pos_tag
text = "NLTK is a leading platform for building Python programs to work with human language data."
tokens = word_tokenize(text)
pos_tags = pos_tag(tokens)
TensorFlow Text:
import tensorflow as tf
import tensorflow_text as text
tokenizer = text.WhitespaceTokenizer()
tokens = tokenizer.tokenize(["TensorFlow Text provides fast, efficient text processing tools."])
Both libraries offer tokenization capabilities, but TensorFlow Text is designed for better integration with TensorFlow's ecosystem and provides optimized performance for large-scale NLP tasks. NLTK offers a broader range of traditional NLP tools and is more suitable for educational purposes and smaller-scale projects.
Topic Modelling for Humans
Pros of Gensim
- More comprehensive and mature library for topic modeling and document similarity
- Easier to use for beginners, with better documentation and tutorials
- Supports a wider range of algorithms and models for text processing
Cons of Gensim
- Less integration with deep learning frameworks
- Slower performance for large-scale text processing tasks
- Limited support for advanced NLP tasks like named entity recognition or sentiment analysis
Code Comparison
Gensim example (topic modeling):
from gensim import corpora, models
dictionary = corpora.Dictionary(texts)
corpus = [dictionary.doc2bow(text) for text in texts]
lda_model = models.LdaMulticore(corpus=corpus, num_topics=10)
TensorFlow Text example (text classification):
import tensorflow as tf
import tensorflow_text as text
model = tf.keras.Sequential([
text.TextVectorization(max_tokens=10000),
tf.keras.layers.Embedding(10000, 16),
tf.keras.layers.GlobalAveragePooling1D(),
tf.keras.layers.Dense(1)
])
Both libraries offer powerful text processing capabilities, but Gensim excels in topic modeling and document similarity tasks, while TensorFlow Text is better suited for deep learning-based NLP applications.
An open-source NLP research library, built on PyTorch.
Pros of AllenNLP
- More comprehensive NLP toolkit with higher-level abstractions
- Easier to use for researchers and practitioners in NLP
- Better documentation and tutorials for getting started
Cons of AllenNLP
- Less flexible for low-level customization compared to TensorFlow Text
- Smaller community and ecosystem than TensorFlow
- Potentially slower performance for some tasks
Code Comparison
AllenNLP example:
from allennlp.predictors import Predictor
predictor = Predictor.from_path("https://storage.googleapis.com/allennlp-public-models/bert-base-srl-2020.03.24.tar.gz")
result = predictor.predict(sentence="Did Uriah honestly think he could beat the game in under three hours?")
TensorFlow Text example:
import tensorflow_text as text
tokenizer = text.WhitespaceTokenizer()
tokens = tokenizer.tokenize(['hello world', 'how are you'])
print(tokens.to_list())
The AllenNLP example shows a high-level API for semantic role labeling, while the TensorFlow Text example demonstrates low-level tokenization. This illustrates AllenNLP's focus on complete NLP tasks versus TensorFlow Text's emphasis on fundamental text processing operations.
Convert
designs to code with AI
Introducing Visual Copilot: A new AI model to turn Figma designs to high quality code using your components.
Try Visual CopilotREADME
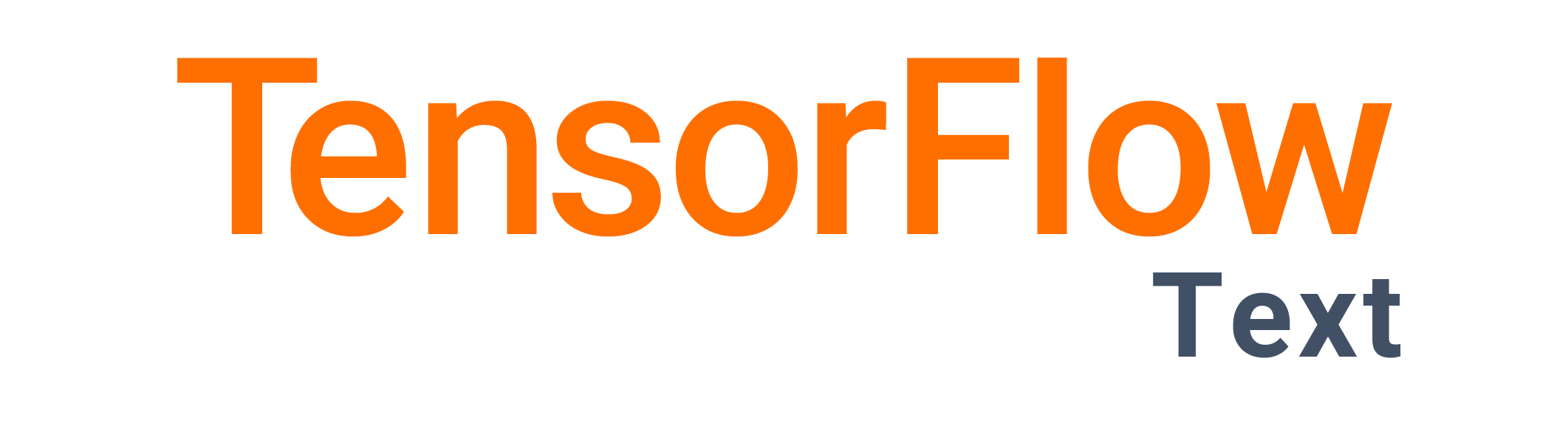
TensorFlow Text - Text processing in Tensorflow
IMPORTANT: When installing TF Text with pip install
, please note the
version of TensorFlow you are running, as you should specify the corresponding
minor version of TF Text (eg. for tensorflow==2.3.x use tensorflow_text==2.3.x).
INDEX
Introduction
TensorFlow Text provides a collection of text related classes and ops ready to use with TensorFlow 2.0. The library can perform the preprocessing regularly required by text-based models, and includes other features useful for sequence modeling not provided by core TensorFlow.
The benefit of using these ops in your text preprocessing is that they are done in the TensorFlow graph. You do not need to worry about tokenization in training being different than the tokenization at inference, or managing preprocessing scripts.
Documentation
Please visit http://tensorflow.org/text for all documentation. This site includes API docs, guides for working with TensorFlow Text, as well as tutorials for building specific models.
Unicode
Most ops expect that the strings are in UTF-8. If you're using a different encoding, you can use the core tensorflow transcode op to transcode into UTF-8. You can also use the same op to coerce your string to structurally valid UTF-8 if your input could be invalid.
docs = tf.constant([u'Everything not saved will be lost.'.encode('UTF-16-BE'),
u'Sadâ¹'.encode('UTF-16-BE')])
utf8_docs = tf.strings.unicode_transcode(docs, input_encoding='UTF-16-BE',
output_encoding='UTF-8')
Normalization
When dealing with different sources of text, it's important that the same words are recognized to be identical. A common technique for case-insensitive matching in Unicode is case folding (similar to lower-casing). (Note that case folding internally applies NFKC normalization.)
We also provide Unicode normalization ops for transforming strings into a canonical representation of characters, with Normalization Form KC being the default (NFKC).
print(text.case_fold_utf8(['Everything not saved will be lost.']))
print(text.normalize_utf8(['Ãffin']))
print(text.normalize_utf8(['Ãffin'], 'nfkd'))
tf.Tensor(['everything not saved will be lost.'], shape=(1,), dtype=string)
tf.Tensor(['\xc3\x84ffin'], shape=(1,), dtype=string)
tf.Tensor(['A\xcc\x88ffin'], shape=(1,), dtype=string)
Tokenization
Tokenization is the process of breaking up a string into tokens. Commonly, these tokens are words, numbers, and/or punctuation.
The main interfaces are Tokenizer
and TokenizerWithOffsets
which each have a
single method tokenize
and tokenizeWithOffsets
respectively. There are
multiple implementing tokenizers available now. Each of these implement
TokenizerWithOffsets
(which extends Tokenizer
) which includes an option for
getting byte offsets into the original string. This allows the caller to know
the bytes in the original string the token was created from.
All of the tokenizers return RaggedTensors with the inner-most dimension of tokens mapping to the original individual strings. As a result, the resulting shape's rank is increased by one. Please review the ragged tensor guide if you are unfamiliar with them. https://www.tensorflow.org/guide/ragged_tensor
WhitespaceTokenizer
This is a basic tokenizer that splits UTF-8 strings on ICU defined whitespace characters (eg. space, tab, new line).
tokenizer = text.WhitespaceTokenizer()
tokens = tokenizer.tokenize(['everything not saved will be lost.', u'Sadâ¹'.encode('UTF-8')])
print(tokens.to_list())
[['everything', 'not', 'saved', 'will', 'be', 'lost.'], ['Sad\xe2\x98\xb9']]
UnicodeScriptTokenizer
This tokenizer splits UTF-8 strings based on Unicode script boundaries. The script codes used correspond to International Components for Unicode (ICU) UScriptCode values. See: http://icu-project.org/apiref/icu4c/uscript_8h.html
In practice, this is similar to the WhitespaceTokenizer
with the most apparent
difference being that it will split punctuation (USCRIPT_COMMON) from language
texts (eg. USCRIPT_LATIN, USCRIPT_CYRILLIC, etc) while also separating language
texts from each other.
tokenizer = text.UnicodeScriptTokenizer()
tokens = tokenizer.tokenize(['everything not saved will be lost.',
u'Sadâ¹'.encode('UTF-8')])
print(tokens.to_list())
[['everything', 'not', 'saved', 'will', 'be', 'lost', '.'],
['Sad', '\xe2\x98\xb9']]
Unicode split
When tokenizing languages without whitespace to segment words, it is common to just split by character, which can be accomplished using the unicode_split op found in core.
tokens = tf.strings.unicode_split([u"ä»
ä»å¹´å".encode('UTF-8')], 'UTF-8')
print(tokens.to_list())
[['\xe4\xbb\x85', '\xe4\xbb\x8a', '\xe5\xb9\xb4', '\xe5\x89\x8d']]
Offsets
When tokenizing strings, it is often desired to know where in the original
string the token originated from. For this reason, each tokenizer which
implements TokenizerWithOffsets
has a tokenize_with_offsets method that will
return the byte offsets along with the tokens. The start_offsets lists the bytes
in the original string each token starts at (inclusive), and the end_offsets
lists the bytes where each token ends at (exclusive, i.e., first byte after
the token).
tokenizer = text.UnicodeScriptTokenizer()
(tokens, start_offsets, end_offsets) = tokenizer.tokenize_with_offsets(
['everything not saved will be lost.', u'Sadâ¹'.encode('UTF-8')])
print(tokens.to_list())
print(start_offsets.to_list())
print(end_offsets.to_list())
[['everything', 'not', 'saved', 'will', 'be', 'lost', '.'],
['Sad', '\xe2\x98\xb9']]
[[0, 11, 15, 21, 26, 29, 33], [0, 3]]
[[10, 14, 20, 25, 28, 33, 34], [3, 6]]
TF.Data Example
Tokenizers work as expected with the tf.data API. A simple example is provided below.
docs = tf.data.Dataset.from_tensor_slices([['Never tell me the odds.'],
["It's a trap!"]])
tokenizer = text.WhitespaceTokenizer()
tokenized_docs = docs.map(lambda x: tokenizer.tokenize(x))
iterator = tokenized_docs.make_one_shot_iterator()
print(iterator.get_next().to_list())
print(iterator.get_next().to_list())
[['Never', 'tell', 'me', 'the', 'odds.']]
[["It's", 'a', 'trap!']]
Keras API
When you use different tokenizers and ops to preprocess your data, the resulting outputs are Ragged Tensors. The Keras API makes it easy now to train a model using Ragged Tensors without having to worry about padding or masking the data, by either using the ToDense layer which handles all of these for you or relying on Keras built-in layers support for natively working on ragged data.
model = tf.keras.Sequential([
tf.keras.layers.InputLayer(input_shape=(None,), dtype='int32', ragged=True)
text.keras.layers.ToDense(pad_value=0, mask=True),
tf.keras.layers.Embedding(100, 16),
tf.keras.layers.LSTM(32),
tf.keras.layers.Dense(32, activation='relu'),
tf.keras.layers.Dense(1, activation='sigmoid')
])
Other Text Ops
TF.Text packages other useful preprocessing ops. We will review a couple below.
Wordshape
A common feature used in some natural language understanding models is to see if the text string has a certain property. For example, a sentence breaking model might contain features which check for word capitalization or if a punctuation character is at the end of a string.
Wordshape defines a variety of useful regular expression based helper functions for matching various relevant patterns in your input text. Here are a few examples.
tokenizer = text.WhitespaceTokenizer()
tokens = tokenizer.tokenize(['Everything not saved will be lost.',
u'Sadâ¹'.encode('UTF-8')])
# Is capitalized?
f1 = text.wordshape(tokens, text.WordShape.HAS_TITLE_CASE)
# Are all letters uppercased?
f2 = text.wordshape(tokens, text.WordShape.IS_UPPERCASE)
# Does the token contain punctuation?
f3 = text.wordshape(tokens, text.WordShape.HAS_SOME_PUNCT_OR_SYMBOL)
# Is the token a number?
f4 = text.wordshape(tokens, text.WordShape.IS_NUMERIC_VALUE)
print(f1.to_list())
print(f2.to_list())
print(f3.to_list())
print(f4.to_list())
[[True, False, False, False, False, False], [True]]
[[False, False, False, False, False, False], [False]]
[[False, False, False, False, False, True], [True]]
[[False, False, False, False, False, False], [False]]
N-grams & Sliding Window
N-grams are sequential words given a sliding window size of n. When combining
the tokens, there are three reduction mechanisms supported. For text, you would
want to use Reduction.STRING_JOIN
which appends the strings to each other.
The default separator character is a space, but this can be changed with the
string_separater argument.
The other two reduction methods are most often used with numerical values, and
these are Reduction.SUM
and Reduction.MEAN
.
tokenizer = text.WhitespaceTokenizer()
tokens = tokenizer.tokenize(['Everything not saved will be lost.',
u'Sadâ¹'.encode('UTF-8')])
# Ngrams, in this case bi-gram (n = 2)
bigrams = text.ngrams(tokens, 2, reduction_type=text.Reduction.STRING_JOIN)
print(bigrams.to_list())
[['Everything not', 'not saved', 'saved will', 'will be', 'be lost.'], []]
Installation
Install using PIP
When installing TF Text with pip install
, please note the version
of TensorFlow you are running, as you should specify the corresponding version
of TF Text. For example, if you're using TF 2.0, install the 2.0 version of TF
Text, and if you're using TF 1.15, install the 1.15 version of TF Text.
pip install -U tensorflow-text==<version>
A note about different operating system packages
After version 2.10, we will only be providing pip packages for Linux x86_64 and Intel-based Macs. TensorFlow Text has always leveraged the release infrastructure of the core TensorFlow package to more easily maintain compatible releases with minimal maintenance, allowing the team to focus on TF Text itself and contributions to other parts of the TensorFlow ecosystem.
For other systems like Windows, Aarch64, and Apple Macs, TensorFlow relies on build collaborators, and so we will not be providing packages for them. However, we will continue to accept PRs to make building for these OSs easy for users, and will try to point to community efforts related to them.
Build from source steps:
Note that TF Text needs to be built in the same environment as TensorFlow. Thus, if you manually build TF Text, it is highly recommended that you also build TensorFlow.
If building on MacOS, you must have coreutils installed. It is probably easiest to do with Homebrew.
- build and install TensorFlow.
- Clone the TF Text repo:
git clone https://github.com/tensorflow/text.git cd text
- Run the build script to create a pip package:
After this step, there should be a./oss_scripts/run_build.sh
*.whl
file in current directory. File name similar totensorflow_text-2.5.0rc0-cp38-cp38-linux_x86_64.whl
. - Install the package to environment:
pip install ./tensorflow_text-*-*-*-os_platform.whl
Build or test using TensorFlow's SIG docker image:
-
Pull image from Tensorflow SIG docker builds.
-
Run a container based with the pulled image and create a bash session. This can be done by running
docker run -it {image_name} bash
.
{image_name}
can be any name with{tf_verison}-python{python_version}
format. An example for python 3.10 and TF version 2.10 :-2.10-python3.10
. -
Clone the TF-Text Github repository inside container:
git clone https://github.com/tensorflow/text.git
.
Once cloned, change to the working directory usingcd text/
. -
Run the configuration script(s):
./oss_scripts/configure.sh
and./oss_scripts/prepare_tf_dep.sh
.
This will update bazel and TF dependencies to installed tensorflow in the container. -
To run the tests, use the bazel command:
bazel test --test_output=errors tensorflow_text:all
. This will run all the tests declared in theBUILD
file.
To run a specific test, modify the above command replacing:all
with the test name (for example:fast_bert_normalizer
). -
Build the pip package/wheel:
bazel build --config=release_cpu_linux oss_scripts/pip_package:build_pip_package
./bazel-bin/oss_scripts/pip_package/build_pip_package /{wheel_dir}
Once the build is complete, you should see the wheel available under
{wheel_dir}
directory.
Top Related Projects
Unsupervised text tokenizer for Neural Network-based text generation.
💥 Fast State-of-the-Art Tokenizers optimized for Research and Production
💫 Industrial-strength Natural Language Processing (NLP) in Python
NLTK Source
Topic Modelling for Humans
An open-source NLP research library, built on PyTorch.
Convert
designs to code with AI
Introducing Visual Copilot: A new AI model to turn Figma designs to high quality code using your components.
Try Visual Copilot