PaddleDetection
Object Detection toolkit based on PaddlePaddle. It supports object detection, instance segmentation, multiple object tracking and real-time multi-person keypoint detection.
Top Related Projects
Models and examples built with TensorFlow
Detectron2 is a platform for object detection, segmentation and other visual recognition tasks.
OpenMMLab Detection Toolbox and Benchmark
YOLOv5 🚀 in PyTorch > ONNX > CoreML > TFLite
Mask R-CNN for object detection and instance segmentation on Keras and TensorFlow
YOLOv4 / Scaled-YOLOv4 / YOLO - Neural Networks for Object Detection (Windows and Linux version of Darknet )
Quick Overview
PaddleDetection is an end-to-end object detection toolkit based on PaddlePaddle, an open-source deep learning platform. It provides a rich set of object detection models, including YOLO, Faster R-CNN, and SSD, along with various data augmentation methods and training strategies. The project aims to make it easy for developers to train and deploy object detection models for various applications.
Pros
- Comprehensive collection of state-of-the-art object detection models
- Extensive data augmentation and training strategies for improved performance
- Easy-to-use APIs and tools for model training, evaluation, and deployment
- Good documentation and examples for various use cases
Cons
- Primarily focused on PaddlePaddle ecosystem, which may limit integration with other deep learning frameworks
- Steeper learning curve for users not familiar with PaddlePaddle
- Some advanced features may require in-depth knowledge of object detection algorithms
Code Examples
- Installing PaddleDetection:
pip install paddledetection
- Training a YOLOv3 model:
from ppdet.engine import Trainer
from ppdet.core.workspace import load_config
cfg = load_config('configs/yolov3/yolov3_darknet53_270e_coco.yml')
trainer = Trainer(cfg)
trainer.train()
- Performing inference with a trained model:
from ppdet.core.workspace import load_config
from ppdet.engine import Trainer
cfg = load_config('configs/yolov3/yolov3_darknet53_270e_coco.yml')
trainer = Trainer(cfg)
trainer.load_weights('output/yolov3_darknet53_270e_coco/model_final')
trainer.predict(['path/to/your/image.jpg'])
Getting Started
- Install PaddlePaddle and PaddleDetection:
pip install paddlepaddle-gpu
pip install paddledetection
- Clone the repository:
git clone https://github.com/PaddlePaddle/PaddleDetection.git
cd PaddleDetection
- Train a model:
python tools/train.py -c configs/yolov3/yolov3_darknet53_270e_coco.yml
- Evaluate the model:
python tools/eval.py -c configs/yolov3/yolov3_darknet53_270e_coco.yml -o weights=output/yolov3_darknet53_270e_coco/model_final
- Perform inference:
python tools/infer.py -c configs/yolov3/yolov3_darknet53_270e_coco.yml -o weights=output/yolov3_darknet53_270e_coco/model_final --infer_img=demo/000000014439.jpg
Competitor Comparisons
Models and examples built with TensorFlow
Pros of TensorFlow Models
- Broader scope, covering various ML tasks beyond object detection
- Larger community and more extensive documentation
- Better integration with TensorFlow ecosystem and tools
Cons of TensorFlow Models
- Can be more complex to use due to its extensive feature set
- May have slower development cycles for specific tasks like object detection
- Potentially higher resource requirements for some models
Code Comparison
PaddleDetection:
from ppdet.core.workspace import create
from ppdet.engine import Trainer
model = create('YOLOv3')
trainer = Trainer(model=model, use_gpu=True)
trainer.train()
TensorFlow Models:
import tensorflow as tf
from object_detection import model_lib_v2
model_fn = model_lib_v2.get_model_fn(
num_classes=90, pipeline_config_path='path/to/config')
estimator = tf.estimator.Estimator(model_fn=model_fn)
estimator.train(input_fn=train_input_fn, max_steps=num_train_steps)
Both repositories offer robust object detection capabilities, but PaddleDetection focuses specifically on this task, while TensorFlow Models covers a broader range of machine learning applications. PaddleDetection may be easier to use for beginners in object detection, while TensorFlow Models provides more flexibility for advanced users and diverse ML projects.
Detectron2 is a platform for object detection, segmentation and other visual recognition tasks.
Pros of Detectron2
- More extensive documentation and tutorials
- Larger community and ecosystem of extensions
- Better integration with PyTorch ecosystem
Cons of Detectron2
- Steeper learning curve for beginners
- Less focus on mobile and edge deployment
- Fewer pre-trained models for specialized tasks
Code Comparison
PaddleDetection:
from ppdet.core.workspace import create
from ppdet.engine import Trainer
model = create('YOLOv3')
trainer = Trainer(model=model, use_gpu=True)
trainer.train()
Detectron2:
from detectron2.config import get_cfg
from detectron2.engine import DefaultTrainer
cfg = get_cfg()
cfg.merge_from_file("config.yaml")
trainer = DefaultTrainer(cfg)
trainer.resume_or_load(resume=False)
trainer.train()
Both frameworks offer similar high-level APIs for model creation and training. PaddleDetection uses a custom workspace system, while Detectron2 relies on a configuration-based approach. Detectron2's code is more tightly integrated with PyTorch conventions, while PaddleDetection uses PaddlePaddle-specific constructs.
OpenMMLab Detection Toolbox and Benchmark
Pros of mmdetection
- More extensive model zoo with a wider variety of pre-trained models
- Better documentation and community support
- More flexible and modular architecture for easier customization
Cons of mmdetection
- Steeper learning curve for beginners
- Slightly more complex configuration system
- May have higher computational requirements for some models
Code Comparison
mmdetection:
from mmdet.apis import init_detector, inference_detector
config_file = 'configs/faster_rcnn/faster_rcnn_r50_fpn_1x_coco.py'
checkpoint_file = 'checkpoints/faster_rcnn_r50_fpn_1x_coco_20200130-047c8118.pth'
model = init_detector(config_file, checkpoint_file, device='cuda:0')
result = inference_detector(model, 'test.jpg')
PaddleDetection:
from ppdet.engine import Detector
from ppdet.utils.visualizer import visualize_results
model = Detector('faster_rcnn_r50_fpn_1x_coco')
result = model.predict('test.jpg')
visualize_results(result, 'test.jpg', output_dir='output')
Both repositories offer powerful object detection frameworks, but mmdetection provides more flexibility and a larger model zoo, while PaddleDetection offers a simpler API for quick deployment.
YOLOv5 🚀 in PyTorch > ONNX > CoreML > TFLite
Pros of YOLOv5
- Simpler architecture and easier to understand for beginners
- Faster training and inference times
- More extensive documentation and community support
Cons of YOLOv5
- Limited flexibility for customization compared to PaddleDetection
- Fewer pre-trained models and datasets available
- Less support for advanced features like multi-object tracking
Code Comparison
YOLOv5:
from yolov5 import YOLOv5
model = YOLOv5('yolov5s.pt')
results = model('image.jpg')
results.show()
PaddleDetection:
from ppdet.engine import Trainer
from ppdet.core.workspace import load_config
cfg = load_config('configs/yolov3/yolov3_darknet53_270e_coco.yml')
trainer = Trainer(cfg)
trainer.train()
YOLOv5 offers a more straightforward API for quick implementation, while PaddleDetection provides a more comprehensive framework with greater customization options. YOLOv5's code is more concise and easier to use out-of-the-box, whereas PaddleDetection requires more setup but offers more flexibility for advanced users.
Mask R-CNN for object detection and instance segmentation on Keras and TensorFlow
Pros of Mask_RCNN
- Simpler implementation, easier to understand and modify
- Well-documented with detailed explanations and examples
- Specifically designed for instance segmentation tasks
Cons of Mask_RCNN
- Less frequently updated compared to PaddleDetection
- Limited to Mask R-CNN architecture, while PaddleDetection offers multiple models
- Smaller community and fewer contributions
Code Comparison
Mask_RCNN:
import mrcnn.model as modellib
model = modellib.MaskRCNN(mode="inference", config=config, model_dir=MODEL_DIR)
model.load_weights(COCO_MODEL_PATH, by_name=True)
results = model.detect([image], verbose=1)
PaddleDetection:
from ppdet.engine import Trainer
from ppdet.core.workspace import load_config, merge_config
cfg = load_config("configs/mask_rcnn/mask_rcnn_r50_fpn_1x_coco.yml")
trainer = Trainer(cfg, mode='eval')
trainer.load_weights(cfg.weights)
trainer.predict([image])
Both repositories provide implementations for object detection and instance segmentation. Mask_RCNN focuses specifically on the Mask R-CNN architecture, while PaddleDetection offers a wider range of models and features. PaddleDetection is more actively maintained and has a larger community, but Mask_RCNN may be easier to understand and modify for those specifically interested in Mask R-CNN implementation.
YOLOv4 / Scaled-YOLOv4 / YOLO - Neural Networks for Object Detection (Windows and Linux version of Darknet )
Pros of darknet
- Lightweight and fast, optimized for real-time object detection
- Supports both CPU and GPU computation
- Extensive documentation and community support
Cons of darknet
- Limited to YOLO-based architectures
- Less flexibility in model customization
- Steeper learning curve for beginners
Code Comparison
darknet:
layer make_convolutional_layer(int batch, int h, int w, int c, int n, int groups, int size, int stride, int padding, ACTIVATION activation, int batch_normalize, int binary, int xnor, int adam)
{
layer l = {0};
l.type = CONVOLUTIONAL;
// ... (additional initialization)
}
PaddleDetection:
class ConvBNLayer(nn.Layer):
def __init__(self,
ch_in,
ch_out,
filter_size=3,
stride=1,
groups=1,
padding=0,
act=None):
super(ConvBNLayer, self).__init__()
# ... (additional initialization)
PaddleDetection offers a more Pythonic and object-oriented approach, while darknet uses a C-style structure initialization. PaddleDetection provides greater flexibility in model architecture design, whereas darknet focuses on efficiency and simplicity for YOLO-based models.
Convert
designs to code with AI
Introducing Visual Copilot: A new AI model to turn Figma designs to high quality code using your components.
Try Visual CopilotREADME
ç®ä½ä¸æ | English
ðç®å½
- ðç®å½
- ðç®ä»
- ð£ææ°è¿å±
- â¡ï¸å¿«éå¼å§
- ð¥ä½ä»£ç å ¨æµç¨å¼å
- ð«å¼æºç¤¾åº
- â¨ä¸»è¦ç¹æ§
- ð±å®è£
- ð¥æç¨
- ðFAQ
- ð§©æ¨¡åç»ä»¶
- ð±æ¨¡ååº
- âï¸æ¨¡åæ§è½å¯¹æ¯
- ðï¸äº§ä¸ç¹è²æ¨¡å|产ä¸å·¥å
·
- ðPP-YOLOE é«ç²¾åº¦ç®æ æ£æµæ¨¡å
- ðPP-YOLOE-R 髿§è½æè½¬æ¡æ£æµæ¨¡å
- ðPP-YOLOE-SOD é«ç²¾åº¦å°ç®æ æ£æµæ¨¡å
- ð«PP-PicoDet è¶ è½»é宿¶ç®æ æ£æµæ¨¡å
- ð¡PP-Tracking 宿¶å¤ç®æ è·è¸ªç³»ç»
- â·ï¸PP-TinyPose 人ä½éª¨éª¼å ³é®ç¹è¯å«
- ðð»PP-Human 宿¶è¡äººåæå·¥å ·
- ðï¸PP-Vehicle 宿¶è½¦è¾åæå·¥å ·
- ð¡äº§ä¸å®è·µèä¾
- ðä¼ä¸åºç¨æ¡ä¾
- ð许å¯è¯ä¹¦
- ðå¼ç¨
ðç®ä»
PaddleDetectionæ¯ä¸ä¸ªåºäºPaddlePaddleçç®æ æ£æµç«¯å°ç«¯å¼åå¥ä»¶ï¼å¨æä¾ä¸°å¯ç模åç»ä»¶åæµè¯åºåçåæ¶ï¼æ³¨é端å°ç«¯ç产ä¸è½å°åºç¨ï¼éè¿æé 产ä¸çº§ç¹è²æ¨¡å|å·¥å ·ã建设产ä¸åºç¨èä¾çææ®µï¼å¸®å©å¼åè å®ç°æ°æ®åå¤ã模åéåãæ¨¡åè®ç»ã模åé¨ç½²çå ¨æµç¨æéï¼å¿«éè¿è¡è½å°åºç¨ã
ä¸»è¦æ¨¡åææç¤ºä¾å¦ä¸ï¼ç¹å»æ é¢å¯å¿«é跳转ï¼ï¼
åæ¶ï¼PaddleDetectionæä¾äºæ¨¡åçå¨çº¿ä½éªåè½ï¼ç¨æ·å¯ä»¥éæ©èªå·±çæ°æ®è¿è¡å¨çº¿æ¨çã
ð£ææ°è¿å±
- ð¥2024.10.1 æ·»å ç®æ æ£æµãå®ä¾åå²é¢åä¸ç«å¼å
¨æµç¨å¼åè½å:
- 飿¡¨ä½ä»£ç å¼åå·¥å
·PaddleXï¼ä¾æäºPaddleDetectionçå
è¿ææ¯ï¼æ¯æäºç®æ æ£æµé¢åçä¸ç«å¼å
¨æµç¨å¼åè½åï¼
- ð¨ æ¨¡å丰å¯ä¸é®è°ç¨ï¼å°éç¨ç®æ æ£æµãå°ç®æ æ£æµåå®ä¾å岿¶åç55ä¸ªæ¨¡åæ´å为3æ¡æ¨¡å产线ï¼éè¿æç®ç**Python APIä¸é®è°ç¨ï¼å¿«éä½éªæ¨¡åææãæ¤å¤ï¼åä¸å¥APIï¼ä¹æ¯æå¾ååç±»ãå¾ååå²ãææ¬å¾åæºè½åæãéç¨OCRãæ¶åºé¢æµçå ±è®¡200+模åï¼å½¢æ20+ååè½æ¨¡åï¼æ¹ä¾¿å¼åè è¿è¡æ¨¡åç»å使ç¨**ã
- ð æé«æçéä½é¨æ§ï¼æä¾åºäºç»ä¸å½ä»¤åå¾å½¢çé¢ä¸¤ç§æ¹å¼ï¼å®ç°æ¨¡åç®æ´é«æç使ç¨ãç»åä¸å®å¶ãæ¯æé«æ§è½é¨ç½²ãæå¡åé¨ç½²å端侧é¨ç½²çå¤ç§é¨ç½²æ¹å¼ãæ¤å¤ï¼å¯¹äºåç§ä¸»æµç¡¬ä»¶å¦è±ä¼è¾¾GPUãæä»è¯ãæè ¾ã坿¦çºªåæµ·å çï¼è¿è¡æ¨¡åå¼åæ¶ï¼é½å¯ä»¥**æ ç¼åæ¢**ã
- æ·»å å®ä¾åå²SOTA模åMask-RT-DETR
- 飿¡¨ä½ä»£ç å¼åå·¥å
·PaddleXï¼ä¾æäºPaddleDetectionçå
è¿ææ¯ï¼æ¯æäºç®æ æ£æµé¢åçä¸ç«å¼å
¨æµç¨å¼åè½åï¼
ð¥è¶ è¶YOLOv8ï¼é£æ¡¨æ¨åºç²¾åº¦æé«ç宿¶æ£æµå¨RT-DETRï¼
RT-DETR解读æç« ä¼ éé¨
ï¼ä»£ç ä¼ éé¨
ï¼RT-DETR
â¡ï¸å¿«éå¼å§
ð¥ä½ä»£ç å ¨æµç¨å¼å
ð«å¼æºç¤¾åº
- ð ï¸ç¤¾åºè´¡ç®ï¼ PaddleDetectioné常欢è¿ä½ å å ¥å°é£æ¡¨ç¤¾åºç弿ºå»ºè®¾ä¸ï¼åä¸è´¡ç®æ¹å¼å¯ä»¥åè弿ºé¡¹ç®å¼åæåã
- ð社åºè¿ææ´»å¨
â¨ä¸»è¦ç¹æ§
ð§©æ¨¡åå设计
PaddleDetectionå°æ£æµæ¨¡åè§£è¦æä¸åçæ¨¡åç»ä»¶ï¼éè¿èªå®ä¹æ¨¡åç»ä»¶ç»åï¼ç¨æ·å¯ä»¥ä¾¿æ·é«æå°å®ææ£æµæ¨¡åçæå»ºãä¼ éé¨
ï¼ð§©æ¨¡åç»ä»¶ã
ð±ä¸°å¯ç模ååº
PaddleDetectionæ¯æå¤§éçææ°ä¸»æµçç®æ³åºå以åé¢è®ç»æ¨¡åï¼æ¶µç2D/3Dç®æ æ£æµãå®ä¾åå²ãäººè¸æ£æµãå
³é®ç¹æ£æµãå¤ç®æ è·è¸ªãåçç£å¦ä¹ çæ¹åãä¼ éé¨
ï¼ð±æ¨¡ååºãâï¸æ¨¡åæ§è½å¯¹æ¯ã
ðï¸äº§ä¸ç¹è²æ¨¡å|产ä¸å·¥å ·
PaddleDetectionæé 产ä¸çº§ç¹è²æ¨¡å以ååæå·¥å
·ï¼PP-YOLOE+ãPP-PicoDetãPP-TinyPoseãPP-HumanV2ãPP-Vehicleçï¼é对éç¨ãé«é¢åç±»åºç¨åºæ¯æä¾æ·±åº¦ä¼åè§£å³æ¹æ¡ä»¥åé«åº¦éæçåæå·¥å
·ï¼éä½å¼åè
çè¯éãéæ©ææ¬ï¼é对ä¸å¡åºæ¯å¿«éåºç¨è½å°ãä¼ éé¨
ï¼ðï¸äº§ä¸ç¹è²æ¨¡å|产ä¸å·¥å
·ã
ð¡ð产ä¸çº§é¨ç½²å®è·µ
PaddleDetectionæ´çå·¥ä¸ãåä¸ãæä¸ã交éãå»çãéèãè½æºçµåçAIåºç¨èä¾ï¼æéæ°æ®æ 注-模åè®ç»-模åè°ä¼-颿µé¨ç½²å
¨æµç¨ï¼æç»éä½ç®æ æ£æµææ¯äº§ä¸è½å°é¨æ§ãä¼ éé¨
ï¼ð¡äº§ä¸å®è·µèä¾ãðä¼ä¸åºç¨æ¡ä¾ã
ð±å®è£
åèå®è£ 说æè¿è¡å®è£ ã
ð¥æç¨
深度å¦ä¹ å ¥é¨æç¨
å¿«éå¼å§
æ°æ®åå¤
é ç½®æä»¶è¯´æ
模åå¼å
- æ°å¢æ£æµæ¨¡å
- äºæ¬¡å¼å
é¨ç½²æ¨ç
- 模åå¯¼åºæç¨
- 模åå缩
- Paddle Inferenceé¨ç½²
- Paddle Liteé¨ç½²
- Paddle Servingé¨ç½²
- ONNX模å导åº
- æ¨çbenchmark
ðFAQ
ð§©æ¨¡åç»ä»¶
Backbones | Necks | Loss | Common | Data Augmentation |
|
ð±æ¨¡ååº
2D Detection | Multi Object Tracking | KeyPoint Detection | Others |
|
âï¸æ¨¡åæ§è½å¯¹æ¯
ð¥ï¸æå¡å¨ç«¯æ¨¡åæ§è½å¯¹æ¯
忍¡åç»æå骨干ç½ç»ç代表模åå¨COCOæ°æ®éä¸ç²¾åº¦mAPååå¡Tesla V100ä¸é¢æµé度(FPS)对æ¯å¾ã
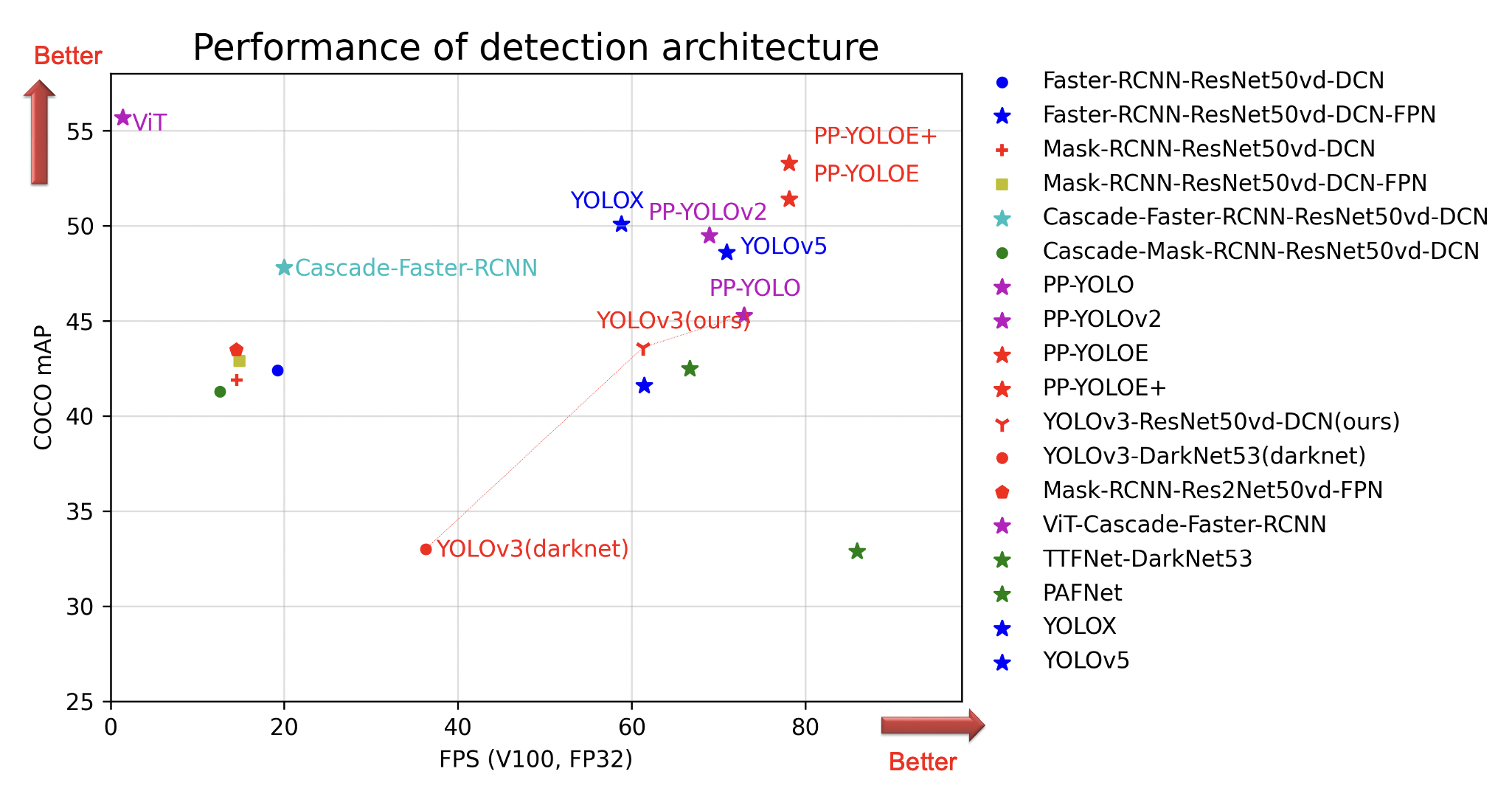
æµè¯è¯´æ(ç¹å»å±å¼)
- ViT为ViT-Cascade-Faster-RCNN模åï¼COCOæ°æ®émAPé«è¾¾55.7%
- Cascade-Faster-RCNN为Cascade-Faster-RCNN-ResNet50vd-DCNï¼PaddleDetectionå°å ¶ä¼åå°COCOæ°æ®mAP为47.8%æ¶æ¨çé度为20FPS
- PP-YOLOEæ¯å¯¹PP-YOLO v2模åçè¿ä¸æ¥ä¼åï¼Lçæ¬å¨COCOæ°æ®émAP为51.6%ï¼Tesla V100颿µé度78.1FPS
- PP-YOLOE+æ¯å¯¹PPOLOE模åçè¿ä¸æ¥ä¼åï¼Lçæ¬å¨COCOæ°æ®émAP为53.3%ï¼Tesla V100颿µé度78.1FPS
- YOLOXåYOLOv5å为åºäºPaddleDetectionå¤ç°ç®æ³ï¼YOLOv5代ç å¨PaddleYOLOä¸ï¼åç §PaddleYOLO_MODEL
- å¾ä¸æ¨¡ååå¯å¨ð±æ¨¡ååºä¸è·å
âï¸ç§»å¨ç«¯æ¨¡åæ§è½å¯¹æ¯
åç§»å¨ç«¯æ¨¡åå¨COCOæ°æ®éä¸ç²¾åº¦mAPåé«ééªé¾865å¤çå¨ä¸é¢æµé度(FPS)对æ¯å¾ã
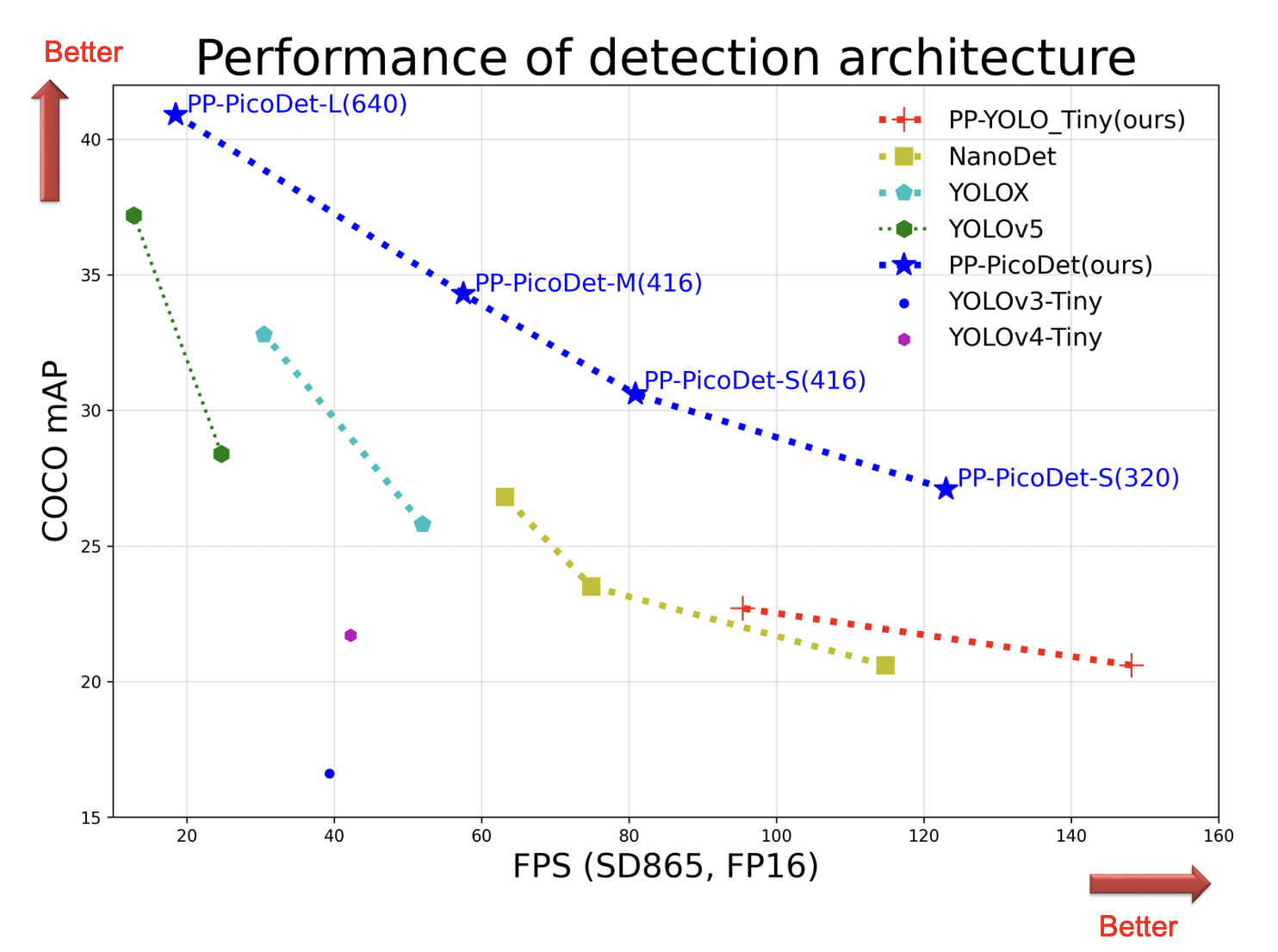
æµè¯è¯´æ(ç¹å»å±å¼)
- æµè¯æ°æ®å使ç¨é«ééªé¾865(4xA77+4xA55)å¤çå¨ï¼batch size为1, å¼å¯4çº¿ç¨æµè¯ï¼æµè¯ä½¿ç¨NCNN颿µåºï¼æµè¯èæ¬è§MobileDetBenchmark
- PP-PicoDetåPP-YOLO-Tiny为PaddleDetectionèªç 模åï¼å¯å¨ð±æ¨¡ååºä¸è·åï¼å ¶ä½æ¨¡åPaddleDetectionææªæä¾
ðï¸äº§ä¸ç¹è²æ¨¡å|产ä¸å·¥å ·
产ä¸ç¹è²æ¨¡åï½äº§ä¸å·¥å ·æ¯PaddleDetectioné对产ä¸é«é¢åºç¨åºæ¯æé çå ¼é¡¾ç²¾åº¦åéåº¦çæ¨¡å以åå·¥å ·ç®±ï¼æ³¨é仿°æ®å¤ç-模åè®ç»-模åè°ä¼-模åé¨ç½²ç端å°ç«¯æéï¼ä¸æä¾äºå®é ç产ç¯å¢ä¸çå®è·µèä¾ä»£ç ï¼å¸®å©æ¥æç±»ä¼¼éæ±çå¼åè 髿çå®æäº§åå¼åè½å°åºç¨ã
è¯¥ç³»åæ¨¡åï½å·¥å ·åå·²PPåç¼å½åï¼å ·ä½ä»ç»ãé¢è®ç»æ¨¡å以å产ä¸å®è·µèä¾ä»£ç å¦ä¸ã
ðPP-YOLOE é«ç²¾åº¦ç®æ æ£æµæ¨¡å
ç®ä»(ç¹å»å±å¼)
PP-YOLOEæ¯åºäºPP-YOLOv2çåè¶çåé¶æ®µAnchor-free模åï¼è¶ è¶äºå¤ç§æµè¡çYOLO模åãPP-YOLOEé¿å äºä½¿ç¨è¯¸å¦Deformable Convolutionæè Matrix NMSä¹ç±»çç¹æ®ç®åï¼ä»¥ä½¿å ¶è½è½»æ¾å°é¨ç½²å¨å¤ç§å¤æ ·ç硬件ä¸ãå ¶ä½¿ç¨å¤§è§æ¨¡æ°æ®éobj365é¢è®ç»æ¨¡åè¿è¡é¢è®ç»ï¼å¯ä»¥å¨ä¸ååºæ¯æ°æ®éä¸å¿«éè°ä¼æ¶æã
ä¼ éé¨
ï¼PP-YOLOE说æã
ä¼ éé¨
ï¼arXiv论æã
é¢è®ç»æ¨¡å(ç¹å»å±å¼)
模ååç§° | COCO精度ï¼mAPï¼ | V100 TensorRT FP16é度(FPS) | æ¨èé¨ç½²ç¡¬ä»¶ | é ç½®æä»¶ | 模åä¸è½½ |
---|---|---|---|---|---|
PP-YOLOE+_l | 53.3 | 149.2 | æå¡å¨ | 龿¥ | ä¸è½½å°å |
ä¼ éé¨
ï¼å
¨é¨é¢è®ç»æ¨¡åã
产ä¸åºç¨ä»£ç 示ä¾(ç¹å»å±å¼)
è¡ä¸ | ç±»å« | äº®ç¹ | ææ¡£è¯´æ | 模åä¸è½½ |
---|---|---|---|---|
åä¸ | åä½ç©æ£æµ | ç¨äºè¡èæ ½å¹ä¸åºäºå¾åççæµåç°åºæºå¨äººææ¯ï¼æä¾äºæ¥èª5ç§ä¸åè¡èåç§çå®å°å®ä¾ | PP-YOLOE+ 䏿¸¸ä»»å¡ | ä¸è½½é¾æ¥ |
éç¨ | ä½å åºæ¯æ£æµ | ä½å æ°æ®é使ç¨ExDarkï¼å æ¬ä»æä½å ç¯å¢å°æ®å ç¯å¢ç10ç§ä¸åå ç §æ¡ä»¶ä¸çå¾çã | PP-YOLOE+ 䏿¸¸ä»»å¡ | ä¸è½½é¾æ¥ |
å·¥ä¸ | PCBçµè·¯æ¿ççµæ£æµ | 工䏿°æ®é使ç¨PKU-Market-PCBï¼è¯¥æ°æ®éç¨äºå°å·çµè·¯æ¿ï¼PCBï¼çççµæ£æµï¼æä¾äº6ç§å¸¸è§çPCBç¼ºé· | PP-YOLOE+ 䏿¸¸ä»»å¡ | ä¸è½½é¾æ¥ |
ðPP-YOLOE-R 髿§è½æè½¬æ¡æ£æµæ¨¡å
ç®ä»(ç¹å»å±å¼)
PP-YOLOE-Ræ¯ä¸ä¸ªé«æçåé¶æ®µAnchor-freeæè½¬æ¡æ£æµæ¨¡åï¼åºäºPP-YOLOE+å¼å ¥äºä¸ç³»åæ¹è¿çç¥æ¥æåæ£æµç²¾åº¦ãæ ¹æ®ä¸åç硬件对精度åé度çè¦æ±ï¼PP-YOLOE-Rå å«s/m/l/xåä¸ªå°ºå¯¸çæ¨¡åãå¨DOTA 1.0æ°æ®éä¸ï¼PP-YOLOE-R-låPP-YOLOE-R-xå¨å尺度è®ç»åæµè¯çæ åµä¸åå«è¾¾å°äº78.14mAPå78.28 mAPï¼è¿å¨å尺度è¯ä¼°ä¸è¶ è¶äºå 乿æçæè½¬æ¡æ£æµæ¨¡åãéè¿å¤å°ºåº¦è®ç»åæµè¯ï¼PP-YOLOE-R-låPP-YOLOE-R-xçæ£æµç²¾åº¦è¿ä¸æ¥æåè³80.02mAPå80.73 mAPï¼è¶ è¶äºææçAnchor-freeæ¹æ³å¹¶ä¸åæå è¿çAnchor-basedç䏤鶿®µæ¨¡å精度å ä¹ç¸å½ãå¨ä¿æé«ç²¾åº¦çåæ¶ï¼PP-YOLOE-Ré¿å 使ç¨ç¹æ®çç®åï¼ä¾å¦Deformable ConvolutionæRotated RoI Alignï¼ä½¿å ¶è½è½»æ¾å°é¨ç½²å¨å¤ç§å¤æ ·ç硬件ä¸ã
ä¼ éé¨
ï¼PP-YOLOE-R说æã
ä¼ éé¨
ï¼arXiv论æã
é¢è®ç»æ¨¡å(ç¹å»å±å¼)
模å | Backbone | mAP | V100 TRT FP16 (FPS) | RTX 2080 Ti TRT FP16 (FPS) | Params (M) | FLOPs (G) | å¦ä¹ ççç¥ | è§åº¦è¡¨ç¤º | æ°æ®å¢å¹¿ | GPUæ°ç® | æ¯GPUå¾çæ°ç® | 模åä¸è½½ | é ç½®æä»¶ |
---|---|---|---|---|---|---|---|---|---|---|---|---|---|
PP-YOLOE-R-l | CRN-l | 80.02 | 69.7 | 48.3 | 53.29 | 281.65 | 3x | oc | MS+RR | 4 | 2 | model | config |
ä¼ éé¨
ï¼å
¨é¨é¢è®ç»æ¨¡åã
产ä¸åºç¨ä»£ç 示ä¾(ç¹å»å±å¼)
è¡ä¸ | ç±»å« | äº®ç¹ | ææ¡£è¯´æ | 模åä¸è½½ |
---|---|---|---|---|
éç¨ | æè½¬æ¡æ£æµ | ææææä½ 䏿PP-YOLOE-Ræè½¬æ¡æ£æµï¼10åéå°èæ±æ°æ®é精度è®ç»è³95mAP | åºäºPP-YOLOE-Rçæè½¬æ¡æ£æµ | ä¸è½½é¾æ¥ |
ðPP-YOLOE-SOD é«ç²¾åº¦å°ç®æ æ£æµæ¨¡å
ç®ä»(ç¹å»å±å¼)
PP-YOLOE-SOD(Small Object Detection)æ¯PaddleDetectionå¢éé对å°ç®æ æ£æµæåºçæ£æµæ¹æ¡ï¼å¨VisDrone-DETæ°æ®éä¸å模å精度达å°38.5mAPï¼è¾¾å°äºSOTAæ§è½ãå ¶åå«åºäºå徿¼å¾æµç¨ä¼åçå°ç®æ æ£æµæ¹æ¡ä»¥ååºäºå徿¨¡åç®æ³ä¼åçå°ç®æ æ£æµæ¹æ¡ãåæ¶æä¾äºæ°æ®éèªå¨åæèæ¬ï¼åªéè¾å ¥æ°æ®éæ æ³¨æä»¶ï¼ä¾¿å¯å¾å°æ°æ®éç»è®¡ç»æï¼è¾ å©å¤ææ°æ®éæ¯å¦æ¯å°ç®æ æ°æ®é以忝å¦éè¦éç¨åå¾çç¥ï¼åæ¶ç»åºç½ç»è¶ åæ°åèå¼ã
ä¼ éé¨
ï¼PP-YOLOE-SOD å°ç®æ æ£æµæ¨¡åã
é¢è®ç»æ¨¡å(ç¹å»å±å¼)
- VisDroneæ°æ®éé¢è®ç»æ¨¡å模å | COCOAPI mAPval 0.5:0.95 | COCOAPI mAPval 0.5 | COCOAPI mAPtest_dev 0.5:0.95 | COCOAPI mAPtest_dev 0.5 | MatlabAPI mAPtest_dev 0.5:0.95 | MatlabAPI mAPtest_dev 0.5 | ä¸è½½ | é ç½®æä»¶ |
---|---|---|---|---|---|---|---|---|
PP-YOLOE+_SOD-l | 31.9 | 52.1 | 25.6 | 43.5 | 30.25 | 51.18 | ä¸è½½é¾æ¥ | é ç½®æä»¶ |
ä¼ éé¨
ï¼å
¨é¨é¢è®ç»æ¨¡åã
产ä¸åºç¨ä»£ç 示ä¾(ç¹å»å±å¼)
è¡ä¸ | ç±»å« | äº®ç¹ | ææ¡£è¯´æ | 模åä¸è½½ |
---|---|---|---|---|
éç¨ | å°ç®æ æ£æµ | åºäºPP-YOLOE-SODçæ äººæºèªæå¾åæ£æµæ¡ä¾å ¨æµç¨å®æã | åºäºPP-YOLOE-SODçæ äººæºèªæå¾åæ£æµ | ä¸è½½é¾æ¥ |
ð«PP-PicoDet è¶ è½»é宿¶ç®æ æ£æµæ¨¡å
ç®ä»(ç¹å»å±å¼)
å ¨æ°çè½»éçº§ç³»åæ¨¡åPP-PicoDetï¼å¨ç§»å¨ç«¯å ·æåè¶çæ§è½ï¼æä¸ºå ¨æ°SOTAè½»é级模åã
ä¼ éé¨
ï¼PP-PicoDet说æã
ä¼ éé¨
ï¼arXiv论æã
é¢è®ç»æ¨¡å(ç¹å»å±å¼)
模ååç§° | COCO精度ï¼mAPï¼ | éªé¾865 å线ç¨é度(FPS) | æ¨èé¨ç½²ç¡¬ä»¶ | é ç½®æä»¶ | 模åä¸è½½ |
---|---|---|---|---|---|
PicoDet-L | 36.1 | 39.7 | ç§»å¨ç«¯ãåµå ¥å¼ | 龿¥ | ä¸è½½å°å |
ä¼ éé¨
ï¼å
¨é¨é¢è®ç»æ¨¡åã
产ä¸åºç¨ä»£ç 示ä¾(ç¹å»å±å¼)
è¡ä¸ | ç±»å« | äº®ç¹ | ææ¡£è¯´æ | 模åä¸è½½ |
---|---|---|---|---|
æºæ §åå¸ | éè·¯å徿£æµ | éè¿å¨å¸æ¿ç¯å«è½¦è¾ä¸å®è£ æå头对路é¢å徿£æµå¹¶åæï¼å®ç°å¯¹è·¯é¢éæçåå¾è¿è¡çæ§ï¼è®°å½å¹¶éç¥ç¯å«äººåæ¸ çï¼å¤§å¤§æåäºç¯å«äººæã | åºäºPP-PicoDetçè·¯é¢å徿£æµ | ä¸è½½é¾æ¥ |
ð¡PP-Tracking 宿¶å¤ç®æ è·è¸ªç³»ç»
ç®ä»(ç¹å»å±å¼)
PaddleDetectionå¢éæä¾äºå®æ¶å¤ç®æ è·è¸ªç³»ç»PP-Trackingï¼æ¯åºäºPaddlePaddle深度å¦ä¹ æ¡æ¶çä¸çé¦ä¸ªå¼æºç宿¶å¤ç®æ è·è¸ªç³»ç»ï¼å ·ææ¨¡å丰å¯ãåºç¨å¹¿æ³åé¨ç½²é«æä¸å¤§ä¼å¿ã PP-Trackingæ¯æåé头è·è¸ª(MOT)åè·¨é头è·è¸ª(MTMCT)ä¸¤ç§æ¨¡å¼ï¼é对å®é ä¸å¡çé¾ç¹åçç¹ï¼æä¾äºè¡äººè·è¸ªã车è¾è·è¸ªãå¤ç±»å«è·è¸ªãå°ç®æ è·è¸ªãæµéç»è®¡ä»¥åè·¨é头è·è¸ªçåç§å¤ç®æ è·è¸ªåè½ååºç¨ï¼é¨ç½²æ¹å¼æ¯æAPIè°ç¨åGUIå¯è§åçé¢ï¼é¨ç½²è¯è¨æ¯æPythonåC++ï¼é¨ç½²å¹³å°ç¯å¢æ¯æLinuxãNVIDIA Jetsonçã
ä¼ éé¨
ï¼PP-Tracking说æã
é¢è®ç»æ¨¡å(ç¹å»å±å¼)
模ååç§° | 模åç®ä» | 精度 | é度(FPS) | æ¨èé¨ç½²ç¡¬ä»¶ | é ç½®æä»¶ | 模åä¸è½½ |
---|---|---|---|---|---|---|
ByteTrack | SDEå¤ç®æ è·è¸ªç®æ³ ä» å 嫿£æµæ¨¡å | MOT-17 test: 78.4 | - | æå¡å¨ãç§»å¨ç«¯ãåµå ¥å¼ | 龿¥ | ä¸è½½å°å |
FairMOT | JDEå¤ç®æ è·è¸ªç®æ³ å¤ä»»å¡èåå¦ä¹ æ¹æ³ | MOT-16 test: 75.0 | - | æå¡å¨ãç§»å¨ç«¯ãåµå ¥å¼ | 龿¥ | ä¸è½½å°å |
OC-SORT | SDEå¤ç®æ è·è¸ªç®æ³ ä» å 嫿£æµæ¨¡å | MOT-17 half val: 75.5 | - | æå¡å¨ãç§»å¨ç«¯ãåµå ¥å¼ | 龿¥ | ä¸è½½å°å |
产ä¸åºç¨ä»£ç 示ä¾(ç¹å»å±å¼)
è¡ä¸ | ç±»å« | äº®ç¹ | ææ¡£è¯´æ | 模åä¸è½½ |
---|---|---|---|---|
éç¨ | å¤ç®æ è·è¸ª | å¿«é䏿åé头ãå¤é头è·è¸ª | PP-Tracking乿ææç©è½¬å¤ç®æ è·è¸ª | ä¸è½½é¾æ¥ |
â·ï¸PP-TinyPose 人ä½éª¨éª¼å ³é®ç¹è¯å«
ç®ä»(ç¹å»å±å¼)
PaddleDetection ä¸çå ³é®ç¹æ£æµé¨åç´§è·æå è¿çç®æ³ï¼å æ¬ Top-Down å Bottom-Up ä¸¤ç§æ¹æ³ï¼å¯ä»¥æ»¡è¶³ç¨æ·çä¸åéæ±ãåæ¶ï¼PaddleDetection æä¾é对移å¨ç«¯è®¾å¤ä¼åçèªç 宿¶å ³é®ç¹æ£æµæ¨¡å PP-TinyPoseã
ä¼ éé¨
ï¼PP-TinyPose说æã
é¢è®ç»æ¨¡å(ç¹å»å±å¼)
模ååç§° | 模åç®ä» | COCO精度ï¼APï¼ | é度(FPS) | æ¨èé¨ç½²ç¡¬ä»¶ | é ç½®æä»¶ | 模åä¸è½½ |
---|---|---|---|---|---|---|
PP-TinyPose | è½»é级å
³é®ç¹ç®æ³ è¾å ¥å°ºå¯¸256x192 | 68.8 | éªé¾865 å线ç¨: 158.7 FPS | ç§»å¨ç«¯ãåµå ¥å¼ | 龿¥ | ä¸è½½å°å |
ä¼ éé¨
ï¼å
¨é¨é¢è®ç»æ¨¡åã
产ä¸åºç¨ä»£ç 示ä¾(ç¹å»å±å¼)
è¡ä¸ | ç±»å« | äº®ç¹ | ææ¡£è¯´æ | 模åä¸è½½ |
---|---|---|---|---|
è¿å¨ | å¥èº« | æä¾ä»æ¨¡åéåãæ°æ®åå¤ã模åè®ç»ä¼åï¼å°åå¤çé»è¾å模åé¨ç½²çå ¨æµç¨å¯å¤ç¨æ¹æ¡ï¼ææè§£å³äºå¤æå¥èº«å¨ä½ç髿è¯å«ï¼æé AIèæå¥èº«æç»ï¼ | åºäºPP-TinyPoseå¢å¼ºççæºè½å¥èº«å¨ä½è¯å« | ä¸è½½é¾æ¥ |
ðð»PP-Human 宿¶è¡äººåæå·¥å ·
ç®ä»(ç¹å»å±å¼)
PaddleDetectionæ·±å ¥æ¢ç´¢æ ¸å¿è¡ä¸çé«é¢åºæ¯ï¼æä¾äºè¡äººå¼ç®±å³ç¨åæå·¥å ·ï¼æ¯æå¾ç/åé头è§é¢/å¤é头è§é¢/å¨çº¿è§é¢æµå¤ç§è¾å ¥æ¹å¼ï¼å¹¿æ³åºç¨äºæºæ §äº¤éãæºæ §åå¸ãå·¥ä¸å·¡æ£çé¢åãæ¯ææå¡å¨ç«¯é¨ç½²åTensorRTå éï¼T4æå¡å¨ä¸å¯è¾¾å°å®æ¶ã PP-Humanæ¯æå大产ä¸çº§åè½ï¼äºå¤§å¼å¸¸è¡ä¸ºè¯å«ã26ç§äººä½å±æ§åæã宿¶äººæµè®¡æ°ãè·¨é头ï¼ReIDï¼è·è¸ªã
ä¼ éé¨
ï¼PP-Humanè¡äººåæå·¥å
·ä½¿ç¨æåã
é¢è®ç»æ¨¡å(ç¹å»å±å¼)
ä»»å¡ | T4 TensorRT FP16: é度ï¼FPSï¼ | æ¨èé¨ç½²ç¡¬ä»¶ | 模åä¸è½½ | 模åä½ç§¯ |
---|---|---|---|---|
è¡äººæ£æµï¼é«ç²¾åº¦ï¼ | 39.8 | æå¡å¨ | ç®æ æ£æµ | 182M |
è¡äººè·è¸ªï¼é«ç²¾åº¦ï¼ | 31.4 | æå¡å¨ | å¤ç®æ è·è¸ª | 182M |
屿§è¯å«ï¼é«ç²¾åº¦ï¼ | å人 117.6 | æå¡å¨ | ç®æ æ£æµ 屿§è¯å« | ç®æ æ£æµï¼182M 屿§è¯å«ï¼86M |
æåè¯å« | å人 100 | æå¡å¨ | å¤ç®æ è·è¸ª å ³é®ç¹æ£æµ åºäºå ³é®ç¹è¡ä¸ºè¯å« | å¤ç®æ è·è¸ªï¼182M å ³é®ç¹æ£æµï¼101M åºäºå ³é®ç¹è¡ä¸ºè¯å«ï¼21.8M |
é¯å ¥è¯å« | 31.4 | æå¡å¨ | å¤ç®æ è·è¸ª | 182M |
ææ¶è¯å« | 50.8 | æå¡å¨ | è§é¢åç±» | 90M |
æ½çè¯å« | 340.1 | æå¡å¨ | ç®æ æ£æµ åºäºäººä½idçç®æ æ£æµ | ç®æ æ£æµï¼182M åºäºäººä½idçç®æ æ£æµï¼27M |
æçµè¯è¯å« | 166.7 | æå¡å¨ | ç®æ æ£æµ åºäºäººä½idçå¾ååç±» | ç®æ æ£æµï¼182M åºäºäººä½idçå¾ååç±»ï¼45M |
ä¼ éé¨
ï¼å®æ´é¢è®ç»æ¨¡åã
产ä¸åºç¨ä»£ç 示ä¾(ç¹å»å±å¼)
è¡ä¸ | ç±»å« | äº®ç¹ | ææ¡£è¯´æ | 模åä¸è½½ |
---|---|---|---|---|
æºè½å®é² | æåæ£æµ | 飿¡¨è¡äººåæPP-Human䏿ä¾çæåè¯å«ç®æ³ï¼éç¨äºå ³é®ç¹+æ¶ç©ºå¾å·ç§¯ç½ç»çææ¯ï¼å¯¹æåå§¿å¿æ éå¶ãèæ¯ç¯å¢æ è¦æ±ã | åºäºPP-Human v2çæåæ£æµ | ä¸è½½é¾æ¥ |
æºè½å®é² | ææ¶è¯å« | æ¬é¡¹ç®åºäºPaddleVideoè§é¢å¼åå¥ä»¶è®ç»ææ¶è¯å«æ¨¡åï¼ç¶åå°è®ç»å¥½ç模åéæå°PaddleDetectionçPP-Humanä¸ï¼å©åè¡äººè¡ä¸ºåæã | åºäºPP-Humançææ¶è¯å« | ä¸è½½é¾æ¥ |
æºè½å®é² | æåæ£æµ | åºäºPP-Human宿æ¥å®¢åææ´ä½æµç¨ã使ç¨PP-Human宿æ¥å®¢åæä¸é常常è§çåºæ¯ï¼ 1. æ¥å®¢å±æ§è¯å«(åéåè·¨å¢å¯è§åï¼ï¼2. æ¥å®¢è¡ä¸ºè¯å«ï¼æåè¯å«ï¼ã | åºäºPP-Humançæ¥å®¢åææ¡ä¾æç¨ | ä¸è½½é¾æ¥ |
ðï¸PP-Vehicle 宿¶è½¦è¾åæå·¥å ·
ç®ä»(ç¹å»å±å¼)
PaddleDetectionæ·±å ¥æ¢ç´¢æ ¸å¿è¡ä¸çé«é¢åºæ¯ï¼æä¾äºè½¦è¾å¼ç®±å³ç¨åæå·¥å ·ï¼æ¯æå¾ç/åé头è§é¢/å¤é头è§é¢/å¨çº¿è§é¢æµå¤ç§è¾å ¥æ¹å¼ï¼å¹¿æ³åºç¨äºæºæ §äº¤éãæºæ §åå¸ãå·¥ä¸å·¡æ£çé¢åãæ¯ææå¡å¨ç«¯é¨ç½²åTensorRTå éï¼T4æå¡å¨ä¸å¯è¾¾å°å®æ¶ã PP-Vehicle忬å大交éåºæ¯æ ¸å¿åè½ï¼è½¦çè¯å«ã屿§è¯å«ã车æµéç»è®¡ãè¿ç« æ£æµã
ä¼ éé¨
ï¼PP-Vehicle车è¾åæå·¥å
·æåã
é¢è®ç»æ¨¡å(ç¹å»å±å¼)
ä»»å¡ | T4 TensorRT FP16: é度(FPS) | æ¨èé¨ç½²ç¡¬ä»¶ | æ¨¡åæ¹æ¡ | 模åä½ç§¯ |
---|---|---|---|---|
è½¦è¾æ£æµï¼é«ç²¾åº¦ï¼ | 38.9 | æå¡å¨ | ç®æ æ£æµ | 182M |
车è¾è·è¸ªï¼é«ç²¾åº¦ï¼ | 25 | æå¡å¨ | å¤ç®æ è·è¸ª | 182M |
车çè¯å« | 213.7 | æå¡å¨ | è½¦çæ£æµ è½¦çè¯å« | è½¦çæ£æµï¼3.9M 车çå符è¯å«ï¼ 12M |
车è¾å±æ§ | 136.8 | æå¡å¨ | 屿§è¯å« | 7.2M |
ä¼ éé¨
ï¼å®æ´é¢è®ç»æ¨¡åã
产ä¸åºç¨ä»£ç 示ä¾(ç¹å»å±å¼)
è¡ä¸ | ç±»å« | äº®ç¹ | ææ¡£è¯´æ | 模åä¸è½½ |
---|---|---|---|---|
æºæ §äº¤é | 交éçæ§è½¦è¾åæ | æ¬é¡¹ç®åºäºPP-Vehicleæ¼ç¤ºæºæ §äº¤é䏿åéç车æµéçæ§ã车è¾è¿åæ£æµä»¥å车è¾ç»æåï¼è½¦çã车åãé¢è²ï¼åæä¸å¤§åºæ¯ã | åºäºPP-Vehicleç交éçæ§åæç³»ç» | ä¸è½½é¾æ¥ |
ð¡äº§ä¸å®è·µèä¾
产ä¸å®è·µè便¯PaddleDetectioné对é«é¢ç®æ æ£æµåºç¨åºæ¯ï¼æä¾ç端å°ç«¯å¼å示ä¾ï¼å¸®å©å¼åè æéæ°æ®æ 注-模åè®ç»-模åè°ä¼-颿µé¨ç½²å ¨æµç¨ã é对æ¯ä¸ªè便们é½éè¿AI-Studioæä¾äºé¡¹ç®ä»£ç 以å说æï¼ç¨æ·å¯ä»¥åæ¥è¿è¡ä½éªã
ä¼ éé¨
ï¼äº§ä¸å®è·µèä¾å®æ´å表
- åºäºPP-YOLOE-Rçæè½¬æ¡æ£æµ
- åºäºPP-YOLOE-SODçæ äººæºèªæå¾åæ£æµ
- åºäºPP-Vehicleç交éçæ§åæç³»ç»
- åºäºPP-Human v2çæåæ£æµ
- åºäºPP-TinyPoseå¢å¼ºççæºè½å¥èº«å¨ä½è¯å«
- åºäºPP-Humançææ¶è¯å«
- åºäºFaster-RCNNçç·ç 表é¢ççµæ£æµ
- åºäºPaddleDetectionçPCBççµæ£æµ
- åºäºFairMOTå®ç°äººæµéç»è®¡
- åºäºYOLOv3å®ç°è·åæ£æµ
- åºäºPP-PicoDetv2 çè·¯é¢å徿£æµ
- åºäºäººä½å ³é®ç¹æ£æµçåè§æ£æµ
- åºäºPP-Humançæ¥å®¢åææ¡ä¾æç¨
- æç»æ´æ°ä¸...
ðä¼ä¸åºç¨æ¡ä¾
ä¼ä¸åºç¨æ¡ä¾æ¯ä¼ä¸å¨å®ç产ç¯å¢ä¸è½å°åºç¨PaddleDetectionçæ¹æ¡æè·¯ï¼ç¸æ¯äº§ä¸å®è·µèä¾å ¶æ´å¤å¼ºè°æ´ä½æ¹æ¡è®¾è®¡æè·¯ï¼å¯ä¾å¼åè å¨é¡¹ç®æ¹æ¡è®¾è®¡ä¸ååèã
ä¼ éé¨
ï¼ä¼ä¸åºç¨æ¡ä¾å®æ´å表
- ä¸å½åæ¹çµç½ââåçµç«æºæ §å·¡æ£
- å½éçµæ°ââ轨éå¨çº¿æºè½å·¡æ£ç³»ç»
- 京ä¸ç©æµââååºè½¦è¾è¡ä¸ºè¯å«
- ä¸å ´å æâååºä¼ ç»ä»ªè¡¨ç»è®¡çæµ
- å®å¾·æ¶ä»£âå¨åçµæ± é«ç²¾åº¦è´¨éæ£æµ
- ä¸å½ç§å¦é¢ç©ºå¤©ä¿¡æ¯åæ°ç ç©¶é¢ââé«å°å¤«çåºé¥æçæµ
- å¾¡èªæºè½ââåºäºè¾¹ç¼çæ äººæºæºè½å·¡æ£
- æ®å®æ 人æºââé«ç²¾åº¦æ£®æå·¡æ£
- é¢é¦æºè½ââçº¢å¤æ ææµæ¸©çæ§
- å京å°éââå£ç½©æ£æµ
- 鳿ºè¾¾ââå·¥å人åè¿è§è¡ä¸ºæ£æµ
- åå¤å¤©ä¿¡ââè¾ç ¤ç®å¸¦æºå¨äººæºè½å·¡æ£
- 伿©ç©èç½ââ社åºä½æ·åç±»æ¯æå¹¿åç²¾åææ¾
- è³èæ §è§ââ室å 3Dç¹äºåºæ¯ç©ä½åå²ä¸æ£æµ
- æç»æ´æ°ä¸...
ð许å¯è¯ä¹¦
æ¬é¡¹ç®çåå¸åApache 2.0 license许å¯è®¤è¯ã
ðå¼ç¨
@misc{ppdet2019,
title={PaddleDetection, Object detection and instance segmentation toolkit based on PaddlePaddle.},
author={PaddlePaddle Authors},
howpublished = {\url{https://github.com/PaddlePaddle/PaddleDetection}},
year={2019}
}
Top Related Projects
Models and examples built with TensorFlow
Detectron2 is a platform for object detection, segmentation and other visual recognition tasks.
OpenMMLab Detection Toolbox and Benchmark
YOLOv5 🚀 in PyTorch > ONNX > CoreML > TFLite
Mask R-CNN for object detection and instance segmentation on Keras and TensorFlow
YOLOv4 / Scaled-YOLOv4 / YOLO - Neural Networks for Object Detection (Windows and Linux version of Darknet )
Convert
designs to code with AI
Introducing Visual Copilot: A new AI model to turn Figma designs to high quality code using your components.
Try Visual Copilot