Top Related Projects
12 weeks, 26 lessons, 52 quizzes, classic Machine Learning for all
A series of Jupyter notebooks that walk you through the fundamentals of Machine Learning and Deep Learning in Python using Scikit-Learn, Keras and TensorFlow 2.
VIP cheatsheets for Stanford's CS 229 Machine Learning
The "Python Machine Learning (3rd edition)" book code repository
"Probabilistic Machine Learning" - a book series by Kevin Murphy
Interactive deep learning book with multi-framework code, math, and discussions. Adopted at 500 universities from 70 countries including Stanford, MIT, Harvard, and Cambridge.
Quick Overview
The pumpkin-book repository is a community-driven project that provides detailed derivations and explanations for the formulas in the "Machine Learning" textbook by Zhou Zhihua. It aims to help readers better understand the theoretical foundations of machine learning algorithms and techniques.
Pros
- Offers in-depth explanations of complex machine learning concepts
- Collaborative effort with contributions from multiple community members
- Regularly updated with new content and improvements
- Free and open-source resource for machine learning enthusiasts and students
Cons
- Content is primarily in Chinese, which may limit accessibility for non-Chinese speakers
- Focuses on a specific textbook, potentially limiting its applicability to other learning resources
- May require a strong mathematical background to fully understand some derivations
- Lacks interactive elements or code implementations of the discussed algorithms
Note: As this is not a code library but rather a collection of explanations and derivations, the code example and getting started sections have been omitted as per the instructions.
Competitor Comparisons
12 weeks, 26 lessons, 52 quizzes, classic Machine Learning for all
Pros of ML-For-Beginners
- Comprehensive curriculum covering various ML topics
- Hands-on approach with practical exercises and projects
- Available in multiple languages, making it accessible to a wider audience
Cons of ML-For-Beginners
- May be too basic for advanced learners
- Focuses more on breadth than depth in some topics
- Limited coverage of deep learning concepts
Code Comparison
ML-For-Beginners:
from sklearn.model_selection import train_test_split
X_train, X_test, y_train, y_test = train_test_split(X, y, test_size=0.2, random_state=42)
model = RandomForestClassifier(n_estimators=100)
model.fit(X_train, y_train)
Pumpkin-book:
import numpy as np
def sigmoid(x):
return 1 / (1 + np.exp(-x))
def logistic_loss(w, X, y):
return -np.sum(y * np.log(sigmoid(X.dot(w))) + (1 - y) * np.log(1 - sigmoid(X.dot(w))))
The ML-For-Beginners repository provides a more practical, hands-on approach with code examples using popular libraries like scikit-learn. In contrast, the Pumpkin-book repository focuses on implementing algorithms from scratch, providing a deeper understanding of the underlying mathematics and principles.
A series of Jupyter notebooks that walk you through the fundamentals of Machine Learning and Deep Learning in Python using Scikit-Learn, Keras and TensorFlow 2.
Pros of handson-ml2
- More comprehensive coverage of machine learning topics, including deep learning
- Includes Jupyter notebooks with interactive code examples
- Regularly updated with new content and improvements
Cons of handson-ml2
- Primarily in English, which may be a barrier for non-English speakers
- Focuses more on practical implementation rather than theoretical foundations
Code Comparison
handson-ml2:
from sklearn.ensemble import RandomForestClassifier
rf_clf = RandomForestClassifier(n_estimators=100, random_state=42)
rf_clf.fit(X_train, y_train)
y_pred = rf_clf.predict(X_test)
pumpkin-book:
import numpy as np
def sigmoid(x):
return 1 / (1 + np.exp(-x))
def logistic_loss(w, X, y):
return -np.mean(y * np.log(sigmoid(X.dot(w))) + (1 - y) * np.log(1 - sigmoid(X.dot(w))))
The handson-ml2 repository provides practical examples using popular libraries like scikit-learn, while pumpkin-book focuses on implementing algorithms from scratch, emphasizing theoretical understanding.
VIP cheatsheets for Stanford's CS 229 Machine Learning
Pros of stanford-cs-229-machine-learning
- Offers concise cheatsheets for quick reference
- Covers a wide range of machine learning topics
- Available in multiple languages, making it accessible to a global audience
Cons of stanford-cs-229-machine-learning
- Lacks detailed explanations and proofs
- May not be suitable for beginners without prior machine learning knowledge
- Limited code examples and practical implementations
Code Comparison
stanford-cs-229-machine-learning doesn't provide extensive code examples, focusing more on theoretical concepts and formulas. In contrast, pumpkin-book offers some code snippets to illustrate concepts:
pumpkin-book example (Python):
import numpy as np
def sigmoid(x):
return 1 / (1 + np.exp(-x))
def logistic_regression(X, y, learning_rate, num_iterations):
m, n = X.shape
theta = np.zeros(n)
for _ in range(num_iterations):
h = sigmoid(np.dot(X, theta))
gradient = np.dot(X.T, (h - y)) / m
theta -= learning_rate * gradient
return theta
This code snippet demonstrates the implementation of logistic regression, which is not present in the stanford-cs-229-machine-learning repository.
The "Python Machine Learning (3rd edition)" book code repository
Pros of python-machine-learning-book-3rd-edition
- Comprehensive coverage of machine learning concepts with practical Python implementations
- Regularly updated with new content and code examples
- Extensive documentation and explanations for each code snippet
Cons of python-machine-learning-book-3rd-edition
- Primarily focused on Python, which may not be suitable for users of other programming languages
- More complex and advanced topics might be challenging for beginners
Code Comparison
python-machine-learning-book-3rd-edition:
from sklearn.model_selection import train_test_split
from sklearn.preprocessing import StandardScaler
from sklearn.linear_model import LogisticRegression
from sklearn.metrics import accuracy_score
X_train, X_test, y_train, y_test = train_test_split(X, y, test_size=0.3, random_state=1, stratify=y)
pumpkin-book:
import numpy as np
def sigmoid(x):
return 1 / (1 + np.exp(-x))
def logistic_loss(X, y, w, b):
m = X.shape[0]
z = np.dot(X, w) + b
loss = -np.sum(y * np.log(sigmoid(z)) + (1 - y) * np.log(1 - sigmoid(z))) / m
return loss
The code snippets demonstrate the difference in approach between the two repositories. python-machine-learning-book-3rd-edition uses scikit-learn for machine learning tasks, while pumpkin-book implements algorithms from scratch using NumPy.
"Probabilistic Machine Learning" - a book series by Kevin Murphy
Pros of pml-book
- More comprehensive coverage of probabilistic machine learning topics
- Includes Jupyter notebooks with code examples and interactive visualizations
- Regularly updated with new content and improvements
Cons of pml-book
- Larger repository size, which may take longer to clone and navigate
- More complex structure, potentially making it harder for beginners to follow
- Primarily focused on Python, limiting language diversity
Code Comparison
pml-book:
import numpy as np
import matplotlib.pyplot as plt
def plot_gaussian(mu, sigma):
x = np.linspace(mu - 3*sigma, mu + 3*sigma, 100)
y = np.exp(-(x - mu)**2 / (2 * sigma**2)) / (sigma * np.sqrt(2 * np.pi))
plt.plot(x, y)
pumpkin-book:
import numpy as np
def gaussian_pdf(x, mu, sigma):
return 1 / (sigma * np.sqrt(2 * np.pi)) * np.exp(-(x - mu)**2 / (2 * sigma**2))
The pml-book example includes visualization, while the pumpkin-book focuses on the core mathematical function. pml-book tends to provide more complete, application-ready code snippets, whereas pumpkin-book offers concise implementations of fundamental concepts.
Interactive deep learning book with multi-framework code, math, and discussions. Adopted at 500 universities from 70 countries including Stanford, MIT, Harvard, and Cambridge.
Pros of d2l-en
- Comprehensive coverage of deep learning topics with interactive code examples
- Multi-framework support (PyTorch, TensorFlow, and MXNet)
- Available in multiple languages and formats (web, PDF, print book)
Cons of d2l-en
- Steeper learning curve for beginners due to its depth and breadth
- Larger repository size, which may impact download and setup time
Code Comparison
d2l-en example (PyTorch):
import torch
from torch import nn
net = nn.Sequential(nn.Linear(4, 8), nn.ReLU(), nn.Linear(8, 1))
X = torch.rand(size=(2, 4))
net(X)
pumpkin-book example (NumPy):
import numpy as np
def sigmoid(x):
return 1 / (1 + np.exp(-x))
X = np.random.randn(2, 4)
W = np.random.randn(4, 1)
b = np.random.randn(1)
y = sigmoid(np.dot(X, W) + b)
The d2l-en example demonstrates the use of PyTorch's high-level neural network modules, while the pumpkin-book example shows a more basic implementation using NumPy. This reflects the different focus areas of the two repositories, with d2l-en providing a more practical, framework-oriented approach and pumpkin-book offering a more theoretical, foundational perspective.
Convert
designs to code with AI
Introducing Visual Copilot: A new AI model to turn Figma designs to high quality code using your components.
Try Visual CopilotREADME
âå¨å¿åèå¸çãæºå¨å¦ä¹ ãï¼è¥¿çä¹¦ï¼æ¯æºå¨å¦ä¹ é¢åçç»å ¸å ¥é¨ææä¹ä¸ï¼å¨èå¸ä¸ºäºä½¿å°½å¯è½å¤ç读è éè¿è¥¿ç书对æºå¨å¦ä¹ ææäºè§£, æä»¥å¨ä¹¦ä¸å¯¹é¨åå ¬å¼çæ¨å¯¼ç»è没æè¯¦è¿°ï¼ä½æ¯è¿å¯¹é£äºæ³æ·±ç©¶å ¬å¼æ¨å¯¼ç»èçè¯»è æ¥è¯´å¯è½âä¸å¤ªå好âï¼æ¬ä¹¦æ¨å¨å¯¹è¥¿ç书鿝è¾é¾çè§£çå ¬å¼å 以解æï¼ä»¥å对é¨åå ¬å¼è¡¥å å ·ä½çæ¨å¯¼ç»èãâ
读å°è¿éï¼å¤§å®¶å¯è½ä¼çé®ä¸ºå¥åé¢è¿æ®µè¯å äºå¼å·ï¼å 为è¿åªæ¯æä»¬æåçéæ³ï¼åæ¥æä»¬äºè§£å°ï¼å¨èå¸ä¹æä»¥çå»è¿äºæ¨å¯¼ç»èççå®åå æ¯ï¼ä»æ¬å°è®¤ä¸ºâçå·¥ç§æ°å¦åºç¡æå®ç¹ç大äºä¸å¦çåºè¯¥å¯¹è¥¿ç书ä¸çæ¨å¯¼ç»èæ å°é¾å§ï¼è¦ç¹å¨ä¹¦é齿äºï¼ç¥å»çç»èåºè½èè¡¥æåç»ä¹ âãæä»¥......æ¬åç书åªè½ç®æ¯æçæ°å¦æ¸£æ¸£å¨èªå¦çæ¶åè®°ä¸æ¥çç¬è®°ï¼å¸æè½å¤å¸®å©å¤§å®¶é½æä¸ºä¸ååæ ¼çâçå·¥ç§æ°å¦åºç¡æå®ç¹ç大äºä¸å¦çâã
使ç¨è¯´æ
- åçä¹¦çææå 容齿¯ä»¥è¥¿ç书çå 容为åç½®ç¥è¯è¿è¡è¡¨è¿°çï¼æä»¥åç书çæä½³ä½¿ç¨æ¹æ³æ¯ä»¥è¥¿ç书为主线ï¼éå°èªå·±æ¨å¯¼ä¸åºæ¥æè ç䏿çå ¬å¼æ¶åæ¥æ¥é åç书ï¼
- 对äºå妿ºå¨å¦ä¹ çå°ç½ï¼è¥¿ç书第1ç« å第2ç« çå ¬å¼**强çä¸å»ºè®®æ·±ç©¶**ï¼ç®åè¿ä¸ä¸å³å¯ï¼çä½ å¦å¾æç¹é£çæ¶åå忥å齿¥å¾åï¼
- æ¯ä¸ªå ¬å¼çè§£æåæ¨å¯¼æä»¬é½åäºä»¥æ¬ç§æ°å¦åºç¡çè§è§è¿è¡è®²è§£ï¼æä»¥è¶ çº²çæ°å¦ç¥è¯æä»¬é常é½ä¼ä»¥éå½ååèæç®çå½¢å¼ç»åºï¼æå ´è¶£çåå¦å¯ä»¥ç»§ç»æ²¿çæä»¬ç»çèµæè¿è¡æ·±å ¥å¦ä¹ ï¼
- è¥åç书鿲¡æä½ æ³è¦æ¥é çå ¬å¼ï¼æè ä½ åç°åç书åªä¸ªå°æ¹æé误ï¼è¯·æ¯«ä¸ç¹è±«å°å»æä»¬GitHubçIssuesï¼ å°åï¼https://github.com/datawhalechina/pumpkin-book/issues ï¼è¿è¡åé¦ï¼å¨å¯¹åºçåæäº¤ä½ å¸æè¡¥å çå ¬å¼ç¼å·æè å误信æ¯ï¼æä»¬é常ä¼å¨24å°æ¶ä»¥å ç»æ¨åå¤ï¼è¶ è¿24å°æ¶æªåå¤çè¯å¯ä»¥å¾®ä¿¡èç³»æä»¬ï¼å¾®ä¿¡å·ï¼at-Sm1lesï¼ï¼
é å¥èµæº
è§é¢æç¨ï¼https://www.bilibili.com/video/BV1Mh411e7VU
ç»éå¦ä¹ ï¼https://www.datawhale.cn/learn/summary/2
å¨çº¿é 读ï¼https://www.datawhale.cn/learn/summary/2
PDFçæ¬ä¸è½½ï¼https://github.com/datawhalechina/pumpkin-book/releases
纸质ç
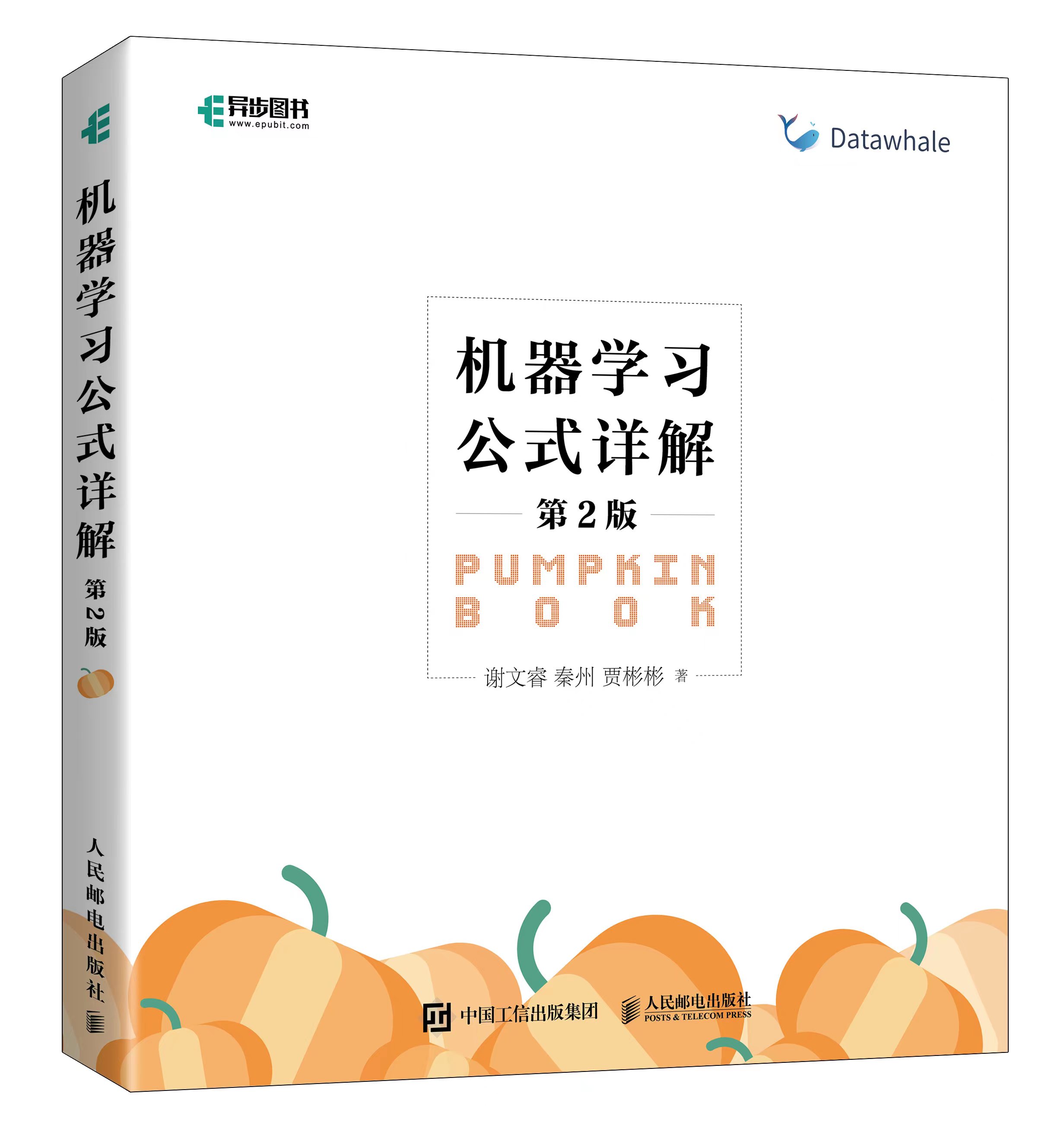
è´ä¹°é¾æ¥ï¼äº¬ä¸ | å½å½ | 天ç«
å误表ï¼https://datawhalechina.github.io/pumpkin-book/#/errata
纸质çå弿ºççåºå«
弿ºçæ¬æ¯æä»¬å¯éåºç社çå ¨ä¹¦å稿ï¼ç»ç±äººæ°é®çµåºç社çç¼è¾èå¸ä»¬å¯¹å稿è¿è¡äºåå¤ä¿®ç¼®æç»è¯çäºçº¸è´¨ä¹¦ç±ï¼å¨æ¤å人æ°é®çµåºç社çç¼è¾èå¸ç认ç严谨表示衷å¿çæè°¢ï¼ï¼éï¼æ ¡å¯¹æ ·ç¨¿ï¼
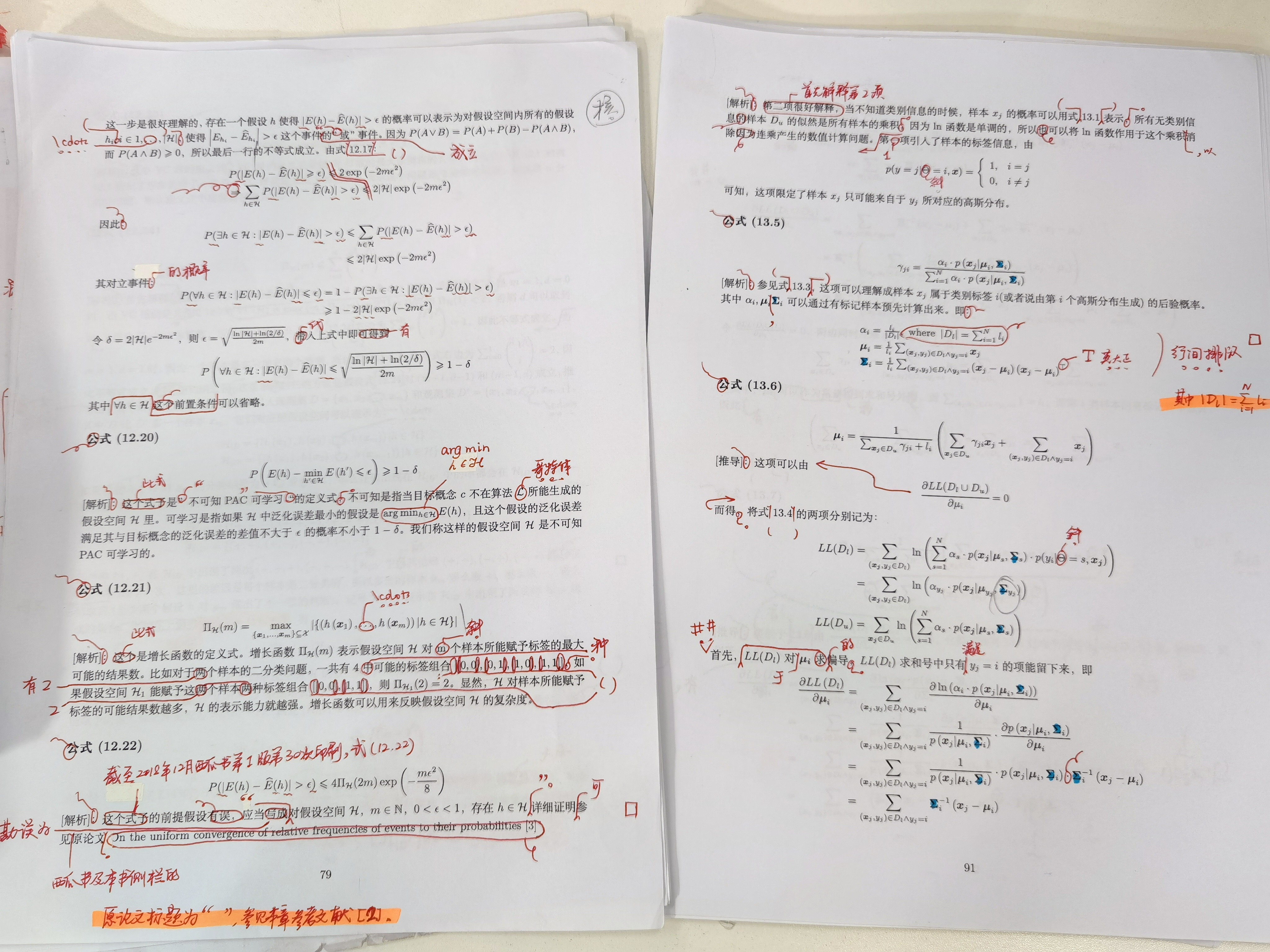

é å¥ç西çä¹¦çæ¬

çæ¬¡ï¼2016å¹´1æç¬¬1ç
å误表ï¼http://cs.nju.edu.cn/zhouzh/zhouzh.files/publication/MLbook2016.htm
ç¼å§ä¼
èè´£ | åå |
---|---|
ä¸»ç¼ | @Sm1les @archwalker @jbb0523 |
ç¼å§ | @juxiao @Majingmin @MrBigFan @shanry @Ye980226 |
å°é¢è®¾è®¡
ææ | åä½ |
---|---|
@Sm1les | æçèç |
è´è°¢
ç¹å«æè°¢@awyd234ã@feijuanã@Ggmatchã@Heitao5200ã@xhqingã@LongJHã@LilRachelã@LeoLRHã@Nono17ã@spareribsã@sunchaothuã@StevenLzq å¨ææ©æçæ¶å对åç书æåçè´¡ç®ã
å ³æ³¨æä»¬
æ«æä¸æ¹äºç»´ç å ³æ³¨å ¬ä¼å·ï¼Datawhaleï¼ç¶ååéâåç书âï¼å³å¯è·åâåç书读è 交æµç¾¤âå ¥ç¾¤æ¹å¼
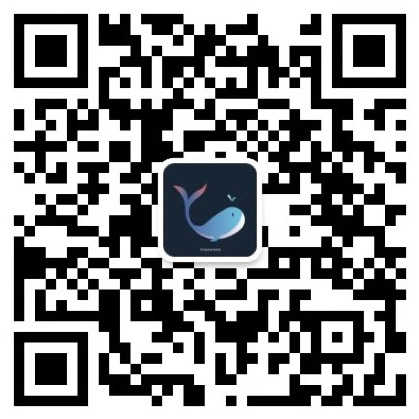
LICENSE
æ¬ä½åéç¨ç¥è¯å ±äº«ç½²å-éå䏿§ä½¿ç¨-ç¸åæ¹å¼å ±äº« 4.0 å½é 许å¯åè®®è¿è¡è®¸å¯ã
Top Related Projects
12 weeks, 26 lessons, 52 quizzes, classic Machine Learning for all
A series of Jupyter notebooks that walk you through the fundamentals of Machine Learning and Deep Learning in Python using Scikit-Learn, Keras and TensorFlow 2.
VIP cheatsheets for Stanford's CS 229 Machine Learning
The "Python Machine Learning (3rd edition)" book code repository
"Probabilistic Machine Learning" - a book series by Kevin Murphy
Interactive deep learning book with multi-framework code, math, and discussions. Adopted at 500 universities from 70 countries including Stanford, MIT, Harvard, and Cambridge.
Convert
designs to code with AI
Introducing Visual Copilot: A new AI model to turn Figma designs to high quality code using your components.
Try Visual Copilot