gradio
Build and share delightful machine learning apps, all in Python. 🌟 Star to support our work!
Top Related Projects
Streamlit — A faster way to build and share data apps.
Data Apps & Dashboards for Python. No JavaScript Required.
Voilà turns Jupyter notebooks into standalone web applications
Interactive Widgets for the Jupyter Notebook
Panel: The powerful data exploration & web app framework for Python
Open source platform for the machine learning lifecycle
Quick Overview
Gradio is an open-source Python library that provides a simple way to create customizable web interfaces for machine learning models, APIs, and functions. It allows developers to quickly build and share interactive demos for their projects, making it easier to showcase and test AI models without extensive web development knowledge.
Pros
- Easy to use and requires minimal code to create functional web interfaces
- Supports a wide range of input and output types, including text, image, audio, and video
- Offers built-in sharing capabilities, allowing users to generate temporary public links for demos
- Integrates well with popular machine learning frameworks like TensorFlow, PyTorch, and Hugging Face
Cons
- Limited customization options for advanced UI designs compared to full-fledged web frameworks
- Performance may be slower for complex models or large datasets
- Documentation can be sparse for some advanced features
- Dependency on external services for certain functionalities (e.g., temporary public links)
Code Examples
- Creating a simple text-to-text interface:
import gradio as gr
def greet(name):
return f"Hello, {name}!"
demo = gr.Interface(fn=greet, inputs="text", outputs="text")
demo.launch()
- Building an image classification demo:
import gradio as gr
from PIL import Image
import numpy as np
def classify_image(img):
# Placeholder for actual classification logic
classes = ["cat", "dog", "bird"]
scores = np.random.rand(3)
return {class_name: float(score) for class_name, score in zip(classes, scores)}
demo = gr.Interface(
fn=classify_image,
inputs=gr.Image(type="pil"),
outputs=gr.Label(num_top_classes=3)
)
demo.launch()
- Creating a chatbot interface:
import gradio as gr
def chatbot(message, history):
# Placeholder for actual chatbot logic
return f"You said: {message}"
demo = gr.ChatInterface(fn=chatbot)
demo.launch()
Getting Started
To get started with Gradio, follow these steps:
-
Install Gradio using pip:
pip install gradio
-
Import Gradio in your Python script:
import gradio as gr
-
Define your function and create an interface:
def my_function(input): # Your logic here return output demo = gr.Interface(fn=my_function, inputs="text", outputs="text")
-
Launch the interface:
demo.launch()
This will start a local web server, and you can access your Gradio interface in your web browser.
Competitor Comparisons
Streamlit — A faster way to build and share data apps.
Pros of Streamlit
- More mature and established ecosystem with extensive documentation
- Wider range of built-in components and data visualization options
- Easier to create complex, multi-page applications
Cons of Streamlit
- Steeper learning curve for beginners
- Less flexibility in UI customization compared to Gradio
- Slower performance for larger applications
Code Comparison
Streamlit:
import streamlit as st
def greet(name):
return f"Hello, {name}!"
name = st.text_input("Enter your name")
if st.button("Greet"):
st.write(greet(name))
Gradio:
import gradio as gr
def greet(name):
return f"Hello, {name}!"
iface = gr.Interface(fn=greet, inputs="text", outputs="text")
iface.launch()
Both Streamlit and Gradio are popular choices for creating web-based user interfaces for machine learning models and data applications. Streamlit offers a more comprehensive set of features and is better suited for complex applications, while Gradio excels in simplicity and ease of use, especially for quick prototyping and demos. The choice between the two depends on the specific requirements of your project and your familiarity with each framework.
Data Apps & Dashboards for Python. No JavaScript Required.
Pros of Dash
- More mature and feature-rich framework for building complex dashboards
- Extensive documentation and large community support
- Seamless integration with Plotly's charting library
Cons of Dash
- Steeper learning curve, especially for beginners
- Requires more boilerplate code for simple applications
- Less focus on rapid prototyping and quick demos
Code Comparison
Gradio example:
import gradio as gr
def greet(name):
return f"Hello, {name}!"
gr.Interface(fn=greet, inputs="text", outputs="text").launch()
Dash example:
import dash
import dash_core_components as dcc
import dash_html_components as html
from dash.dependencies import Input, Output
app = dash.Dash(__name__)
app.layout = html.Div([
dcc.Input(id='input-box', type='text', placeholder='Enter your name'),
html.Div(id='output-text')
])
@app.callback(Output('output-text', 'children'), Input('input-box', 'value'))
def update_output(value):
return f'Hello, {value}!'
if __name__ == '__main__':
app.run_server(debug=True)
Voilà turns Jupyter notebooks into standalone web applications
Pros of Voila
- Seamless integration with Jupyter notebooks, allowing easy conversion of existing notebooks to web applications
- Supports a wide range of Jupyter widgets and custom JavaScript libraries
- Offers more flexibility in layout and design compared to Gradio
Cons of Voila
- Requires more setup and configuration compared to Gradio's simpler interface
- Less focus on machine learning model deployment, which is a key feature of Gradio
- May have a steeper learning curve for users not familiar with Jupyter ecosystem
Code Comparison
Voila (using a Jupyter notebook):
from ipywidgets import interact, interactive, fixed
import ipywidgets as widgets
@interact(x=10)
def square(x):
print(x**2)
Gradio:
import gradio as gr
def square(x):
return x**2
iface = gr.Interface(fn=square, inputs="number", outputs="number")
iface.launch()
Both Gradio and Voila are tools for creating web interfaces, but they cater to different use cases. Gradio is more focused on quickly deploying machine learning models, while Voila offers more flexibility for converting Jupyter notebooks into interactive dashboards. The choice between them depends on the specific requirements of your project and your familiarity with the Jupyter ecosystem.
Interactive Widgets for the Jupyter Notebook
Pros of ipywidgets
- Deeply integrated with Jupyter ecosystem, ideal for interactive data analysis
- Extensive widget library with rich customization options
- Supports bidirectional communication between Python and JavaScript
Cons of ipywidgets
- Limited to Jupyter environments, not easily shareable as standalone applications
- Steeper learning curve, especially for users unfamiliar with Jupyter
Code Comparison
ipywidgets:
import ipywidgets as widgets
from IPython.display import display
slider = widgets.IntSlider(description='Value')
output = widgets.Output()
display(slider, output)
gradio:
import gradio as gr
def update(value):
return f"Selected value: {value}"
gr.Interface(fn=update, inputs="slider", outputs="text").launch()
Key Differences
- ipywidgets is tailored for Jupyter notebooks, while gradio focuses on creating shareable web interfaces
- gradio offers simpler API for quick prototyping, while ipywidgets provides more granular control
- ipywidgets requires Jupyter environment, gradio can run as standalone Python script
Panel: The powerful data exploration & web app framework for Python
Pros of Panel
- More flexible and customizable UI components
- Better integration with data visualization libraries like Bokeh and Matplotlib
- Supports a wider range of data types and formats
Cons of Panel
- Steeper learning curve for beginners
- Less focus on machine learning and AI-specific use cases
- Requires more code to create basic interfaces
Code Comparison
Panel:
import panel as pn
def hello(name):
return f"Hello, {name}!"
pn.interact(hello, name="World")
Gradio:
import gradio as gr
def hello(name):
return f"Hello, {name}!"
gr.Interface(fn=hello, inputs="text", outputs="text").launch()
Both Gradio and Panel are powerful tools for creating interactive web interfaces for Python applications. Gradio excels in simplicity and ease of use, especially for machine learning models, while Panel offers more flexibility and advanced features for data visualization and complex dashboards. The choice between the two depends on the specific requirements of your project and your familiarity with web development concepts.
Open source platform for the machine learning lifecycle
Pros of MLflow
- Comprehensive end-to-end ML lifecycle management
- Robust experiment tracking and model versioning
- Integration with various ML frameworks and deployment platforms
Cons of MLflow
- Steeper learning curve for beginners
- More complex setup and configuration
- Less focus on quick prototyping and demo creation
Code Comparison
MLflow example:
import mlflow
mlflow.start_run()
mlflow.log_param("param1", 5)
mlflow.log_metric("accuracy", 0.85)
mlflow.end_run()
Gradio example:
import gradio as gr
def greet(name):
return f"Hello {name}!"
gr.Interface(fn=greet, inputs="text", outputs="text").launch()
Key Differences
- MLflow is designed for comprehensive ML lifecycle management, while Gradio focuses on creating interactive demos and prototypes.
- MLflow offers more extensive tracking and versioning capabilities, whereas Gradio excels in rapid UI creation for ML models.
- MLflow requires more setup but provides deeper integration with ML workflows, while Gradio emphasizes simplicity and quick deployment.
Both tools serve different purposes in the ML ecosystem, with MLflow being more suited for production-level ML pipelines and Gradio for quick demos and prototyping.
Convert
designs to code with AI
Introducing Visual Copilot: A new AI model to turn Figma designs to high quality code using your components.
Try Visual CopilotREADME
Gradio: Build Machine Learning Web Apps â in Python
Gradio is an open-source Python package that allows you to quickly build a demo or web application for your machine learning model, API, or any arbitrary Python function. You can then share a link to your demo or web application in just a few seconds using Gradio's built-in sharing features. No JavaScript, CSS, or web hosting experience needed!
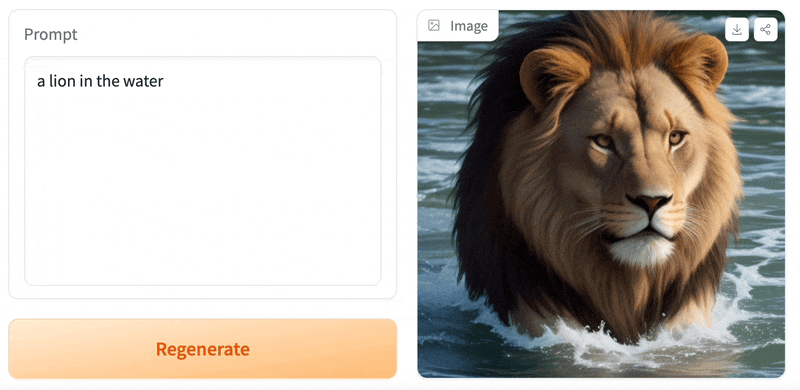
It just takes a few lines of Python to create a beautiful demo like the one above, so let's get started ð«
Installation
Prerequisite: Gradio requires Python 3.8 or higher
We recommend installing Gradio using pip
, which is included by default in Python. Run this in your terminal or command prompt:
pip install gradio
[!TIP] It is best to install Gradio in a virtual environment. Detailed installation instructions for all common operating systems are provided here.
Building Your First Demo
You can run Gradio in your favorite code editor, Jupyter notebook, Google Colab, or anywhere else you write Python. Let's write your first Gradio app:
import gradio as gr
def greet(name, intensity):
return "Hello " * intensity + name + "!"
demo = gr.Interface(
fn=greet,
inputs=["text", "slider"],
outputs=["text"],
)
demo.launch()
[!TIP] We shorten the imported name from
gradio
togr
for better readability of code. This is a widely adopted convention that you should follow so that anyone working with your code can easily understand it.
Now, run your code. If you've written the Python code in a file named, for example, app.py
, then you would run python app.py
from the terminal.
The demo below will open in a browser on http://localhost:7860 if running from a file. If you are running within a notebook, the demo will appear embedded within the notebook.
Type your name in the textbox on the left, drag the slider, and then press the Submit button. You should see a friendly greeting on the right.
[!TIP] When developing locally, you can run your Gradio app in hot reload mode, which automatically reloads the Gradio app whenever you make changes to the file. To do this, simply type in
gradio
before the name of the file instead ofpython
. In the example above, you would type:gradio app.py
in your terminal. Learn more about hot reloading in the Hot Reloading Guide.
Understanding the Interface
Class
You'll notice that in order to make your first demo, you created an instance of the gr.Interface
class. The Interface
class is designed to create demos for machine learning models which accept one or more inputs, and return one or more outputs.
The Interface
class has three core arguments:
fn
: the function to wrap a user interface (UI) aroundinputs
: the Gradio component(s) to use for the input. The number of components should match the number of arguments in your function.outputs
: the Gradio component(s) to use for the output. The number of components should match the number of return values from your function.
The fn
argument is very flexible -- you can pass any Python function that you want to wrap with a UI. In the example above, we saw a relatively simple function, but the function could be anything from a music generator to a tax calculator to the prediction function of a pretrained machine learning model.
The input
and output
arguments take one or more Gradio components. As we'll see, Gradio includes more than 30 built-in components (such as the gr.Textbox()
, gr.Image()
, and gr.HTML()
components) that are designed for machine learning applications.
[!TIP] For the
inputs
andoutputs
arguments, you can pass in the name of these components as a string ("textbox"
) or an instance of the class (gr.Textbox()
).
If your function accepts more than one argument, as is the case above, pass a list of input components to inputs
, with each input component corresponding to one of the arguments of the function, in order. The same holds true if your function returns more than one value: simply pass in a list of components to outputs
. This flexibility makes the Interface
class a very powerful way to create demos.
We'll dive deeper into the gr.Interface
on our series on building Interfaces.
Sharing Your Demo
What good is a beautiful demo if you can't share it? Gradio lets you easily share a machine learning demo without having to worry about the hassle of hosting on a web server. Simply set share=True
in launch()
, and a publicly accessible URL will be created for your demo. Let's revisit our example demo, but change the last line as follows:
import gradio as gr
def greet(name):
return "Hello " + name + "!"
demo = gr.Interface(fn=greet, inputs="textbox", outputs="textbox")
demo.launch(share=True) # Share your demo with just 1 extra parameter ð
When you run this code, a public URL will be generated for your demo in a matter of seconds, something like:
ð https://a23dsf231adb.gradio.live
Now, anyone around the world can try your Gradio demo from their browser, while the machine learning model and all computation continues to run locally on your computer.
To learn more about sharing your demo, read our dedicated guide on sharing your Gradio application.
An Overview of Gradio
So far, we've been discussing the Interface
class, which is a high-level class that lets to build demos quickly with Gradio. But what else does Gradio do?
Chatbots with gr.ChatInterface
Gradio includes another high-level class, gr.ChatInterface
, which is specifically designed to create Chatbot UIs. Similar to Interface
, you supply a function and Gradio creates a fully working Chatbot UI. If you're interested in creating a chatbot, you can jump straight our dedicated guide on gr.ChatInterface
.
Custom Demos with gr.Blocks
Gradio also offers a low-level approach for designing web apps with more flexible layouts and data flows with the gr.Blocks
class. Blocks allows you to do things like control where components appear on the page, handle complex data flows (e.g. outputs can serve as inputs to other functions), and update properties/visibility of components based on user interaction â still all in Python.
You can build very custom and complex applications using gr.Blocks()
. For example, the popular image generation Automatic1111 Web UI is built using Gradio Blocks. We dive deeper into the gr.Blocks
on our series on building with Blocks.
The Gradio Python & JavaScript Ecosystem
That's the gist of the core gradio
Python library, but Gradio is actually so much more! It's an entire ecosystem of Python and JavaScript libraries that let you build machine learning applications, or query them programmatically, in Python or JavaScript. Here are other related parts of the Gradio ecosystem:
- Gradio Python Client (
gradio_client
): query any Gradio app programmatically in Python. - Gradio JavaScript Client (
@gradio/client
): query any Gradio app programmatically in JavaScript. - Gradio-Lite (
@gradio/lite
): write Gradio apps in Python that run entirely in the browser (no server needed!), thanks to Pyodide. - Hugging Face Spaces: the most popular place to host Gradio applications â for free!
What's Next?
Keep learning about Gradio sequentially using the Gradio Guides, which include explanations as well as example code and embedded interactive demos. Next up: key features about Gradio demos.
Or, if you already know the basics and are looking for something specific, you can search the more technical API documentation.
Questions?
If you'd like to report a bug or have a feature request, please create an issue on GitHub. For general questions about usage, we are available on our Discord server and happy to help.
If you like Gradio, please leave us a â on GitHub!
Open Source Stack
Gradio is built on top of many wonderful open-source libraries!
License
Gradio is licensed under the Apache License 2.0 found in the LICENSE file in the root directory of this repository.
Citation
Also check out the paper Gradio: Hassle-Free Sharing and Testing of ML Models in the Wild, ICML HILL 2019, and please cite it if you use Gradio in your work.
@article{abid2019gradio,
title = {Gradio: Hassle-Free Sharing and Testing of ML Models in the Wild},
author = {Abid, Abubakar and Abdalla, Ali and Abid, Ali and Khan, Dawood and Alfozan, Abdulrahman and Zou, James},
journal = {arXiv preprint arXiv:1906.02569},
year = {2019},
}
Top Related Projects
Streamlit — A faster way to build and share data apps.
Data Apps & Dashboards for Python. No JavaScript Required.
Voilà turns Jupyter notebooks into standalone web applications
Interactive Widgets for the Jupyter Notebook
Panel: The powerful data exploration & web app framework for Python
Open source platform for the machine learning lifecycle
Convert
designs to code with AI
Introducing Visual Copilot: A new AI model to turn Figma designs to high quality code using your components.
Try Visual Copilot