interactive-deep-colorization
Deep learning software for colorizing black and white images with a few clicks.
Top Related Projects
Automatic colorization using deep neural networks. "Colorful Image Colorization." In ECCV, 2016.
A Deep Learning based project for colorizing and restoring old images (and video!)
The source code of 'Visual Attribute Transfer through Deep Image Analogy'.
Quick Overview
The interactive-deep-colorization repository is a project that implements an interactive deep learning-based image colorization system. It allows users to add color hints to a grayscale image, which the AI then uses to generate a fully colorized version. This project combines state-of-the-art deep learning techniques with user interaction for more controlled and accurate colorization results.
Pros
- Provides an interactive approach to image colorization, allowing for user input and control
- Utilizes advanced deep learning techniques for high-quality colorization results
- Offers both a local GUI application and a remote web interface for accessibility
- Includes pre-trained models for immediate use
Cons
- Requires significant computational resources, especially GPU, for optimal performance
- Limited to colorizing still images, not applicable to videos
- May produce less accurate results for complex or unusual scenes without user guidance
- Requires some technical knowledge to set up and run, especially for the local application
Code Examples
# Load and preprocess the grayscale image
img = load_img('path/to/grayscale_image.jpg')
h, w = img.shape[:2]
img_rs = resize_img(img, h=256)
img_rs_np = np.asarray(img_rs)
img_ab_313 = np.load('path/to/pts_in_hull.npy')
# Add color hint
color_hint = np.zeros((2, h, w))
color_hint[0, 100:150, 100:150] = 100 # Add a blue hint
color_hint[1, 100:150, 100:150] = -100
# Colorize the image
output = colorize_image(img_rs_np, color_hint, img_ab_313)
# Load the pre-trained colorization model
colorizer = load_model('path/to/colorization_model.pth')
# Prepare the input tensor
input_tensor = preprocess_image(grayscale_image)
# Generate colorized output
with torch.no_grad():
output = colorizer(input_tensor)
# Post-process and display the result
colorized_image = postprocess_output(output)
plt.imshow(colorized_image)
plt.show()
Getting Started
-
Clone the repository:
git clone https://github.com/junyanz/interactive-deep-colorization.git cd interactive-deep-colorization
-
Install dependencies:
pip install -r requirements.txt
-
Download pre-trained models:
bash download_models.sh
-
Run the local GUI application:
python ideepcolor_local.py
For the web interface, follow the additional setup instructions in the repository's README.
Competitor Comparisons
Automatic colorization using deep neural networks. "Colorful Image Colorization." In ECCV, 2016.
Pros of colorization
- Simpler implementation, easier to understand and modify
- Supports both automatic and interactive colorization modes
- Includes pre-trained models for immediate use
Cons of colorization
- Less advanced user interface for interactive colorization
- May produce less vibrant or realistic colors in some cases
- Limited options for fine-tuning the colorization process
Code comparison
interactive-deep-colorization:
def compute_dist(mask):
mask = mask.astype(np.float32)
dist = cv2.distanceTransform(mask, cv2.DIST_L2, cv2.DIST_MASK_PRECISE)
return dist
colorization:
def postprocess_tens(tens_orig, out_ab, mask_mult):
tens_orig = tens_orig.cpu().numpy()
out_ab = out_ab.cpu().numpy()
mask_mult = mask_mult.cpu().numpy()
return np.concatenate((tens_orig, out_ab * mask_mult), axis=1)
Both repositories focus on image colorization using deep learning techniques. interactive-deep-colorization offers a more sophisticated interactive interface, allowing users to provide color hints for specific areas. colorization, on the other hand, provides a simpler implementation that supports both automatic and interactive modes. The code comparison shows different approaches to image processing, with interactive-deep-colorization focusing on distance computation for masks, while colorization emphasizes post-processing of colorized outputs.
A Deep Learning based project for colorizing and restoring old images (and video!)
Pros of DeOldify
- Fully automated colorization process, requiring minimal user input
- Supports both image and video colorization
- Utilizes more recent deep learning techniques, potentially yielding better results
Cons of DeOldify
- Less control over specific color choices for individual elements
- May struggle with certain complex scenes or unusual color patterns
- Requires more computational resources for processing
Code Comparison
DeOldify:
colorizer = get_video_colorizer()
render_factor = 21
colorizer.get_transformed_image("input.jpg", render_factor=render_factor)
Interactive Deep Colorization:
img = cv2.imread('input.jpg')
(H_orig,W_orig) = img.shape[:2]
img = cv2.resize(img, (256, 256))
lab_img = cv2.cvtColor(img, cv2.COLOR_BGR2LAB)
Summary
DeOldify offers a more automated approach to colorization with support for both images and videos, while Interactive Deep Colorization provides greater user control over the colorization process. DeOldify may produce better results in some cases due to newer techniques, but it requires more computational power. Interactive Deep Colorization allows for more precise color selection but demands more user input and expertise.
The source code of 'Visual Attribute Transfer through Deep Image Analogy'.
Pros of Deep-Image-Analogy
- Focuses on image-to-image translation and style transfer
- Provides more advanced image manipulation capabilities
- Suitable for complex image editing tasks
Cons of Deep-Image-Analogy
- Less user-friendly for non-technical users
- Requires more computational resources
- Limited to specific image transformation tasks
Code Comparison
Deep-Image-Analogy:
void DeepAnalogy::NNFsearch(int level)
{
for (int i = 0; i < _iters; i++)
{
NNFsearch_random(level);
NNFsearch_propagation(level);
}
}
interactive-deep-colorization:
def create_model(self, opt):
model = networks.define_G(opt.input_nc, opt.output_nc, opt.ngf, opt.netG, opt.norm,
not opt.no_dropout, opt.init_type, opt.init_gain, self.gpu_ids)
return model
The code snippets show that Deep-Image-Analogy uses C++ for its implementation, focusing on NNF search algorithms, while interactive-deep-colorization uses Python and relies on a more modular approach with network definitions. This reflects the different focus areas and complexity levels of the two projects.
Convert
designs to code with AI
Introducing Visual Copilot: A new AI model to turn Figma designs to high quality code using your components.
Try Visual CopilotREADME
Interactive Deep Colorization
Project Page | Paper | Demo Video | SIGGRAPH Talk
04/10/2020 Update: @mabdelhack provided a windows installation guide for the PyTorch model in Python 3.6. Check out the Windows branch for the guide.
10/3/2019 Update: Our technology is also now available in Adobe Photoshop Elements 2020. See this blog and video for more details.
9/3/2018 Update: The code now supports a backend PyTorch model (with PyTorch 0.5.0+). Please find the Local Hints Network training code in the colorization-pytorch repository.
Real-Time User-Guided Image Colorization with Learned Deep Priors.
Richard Zhang*, Jun-Yan Zhu*, Phillip Isola, Xinyang Geng, Angela S. Lin, Tianhe Yu, and Alexei A. Efros.
In ACM Transactions on Graphics (SIGGRAPH 2017).
(*indicates equal contribution)
We first describe the system (0) Prerequisities and steps for (1) Getting started. We then describe the interactive colorization demo (2) Interactive Colorization (Local Hints Network). There are two demos: (a) a "barebones" version in iPython notebook and (b) the full GUI we used in our paper. We then provide an example of the (3) Global Hints Network.
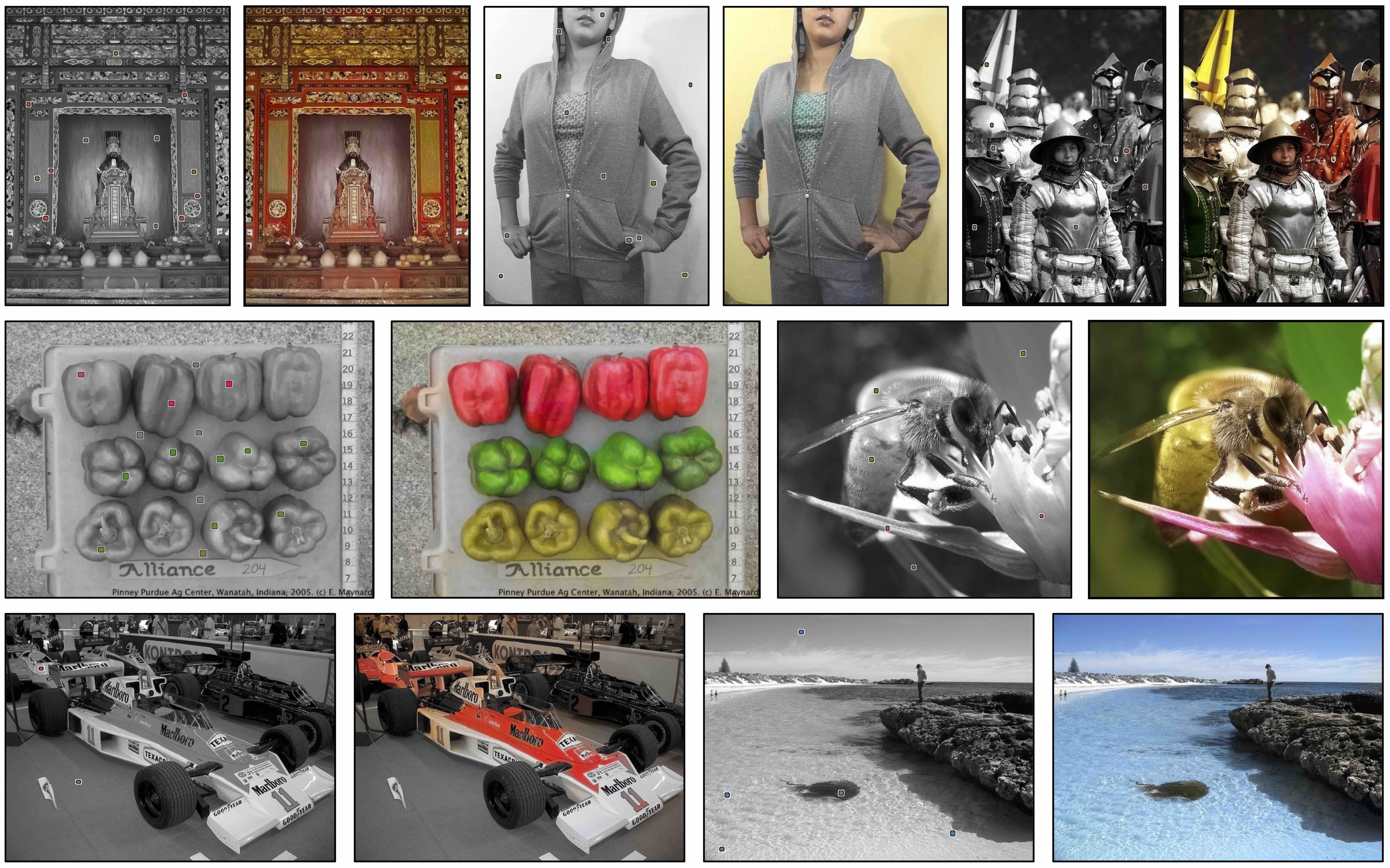
(0) Prerequisites
- Linux or OSX
- Caffe or PyTorch
- CPU or NVIDIA GPU + CUDA CuDNN.
(1) Getting Started
- Clone this repo:
git clone https://github.com/junyanz/interactive-deep-colorization ideepcolor
cd ideepcolor
- Download the reference model
bash ./models/fetch_models.sh
- Install Caffe or PyTorch and 3rd party Python libraries (OpenCV, scikit-learn and scikit-image). See the Requirements for more details.
(2) Interactive Colorization (Local Hints Network)

We provide a "barebones" demo in iPython notebook, which does not require QT. We also provide our full GUI demo.
(2a) Barebones Interactive Colorization Demo
- Run
ipython notebook
and click onDemoInteractiveColorization.ipynb
.
If you need to convert the Notebook to an older version, use jupyter nbconvert --to notebook --nbformat 3 ./DemoInteractiveColorization.ipynb
.
(2b) Full Demo GUI
-
Install Qt5 and QDarkStyle. (See Installation)
-
Run the UI:
python ideepcolor.py --gpu [GPU_ID] --backend [CAFFE OR PYTORCH]
. Arguments are described below:
--win_size [512] GUI window size
--gpu [0] GPU number
--image_file ['./test_imgs/mortar_pestle.jpg'] path to the image file
--backend ['caffe'] either use 'caffe' or 'pytorch'; 'caffe' is the official model from siggraph 2017, and 'pytorch' is the same weights converted
- User interactions

- Adding points: Left-click somewhere on the input pad
- Moving points: Left-click and hold on a point on the input pad, drag to desired location, and let go
- Changing colors: For currently selected point, choose a recommended color (middle-left) or choose a color on the ab color gamut (top-left)
- Removing points: Right-click on a point on the input pad
- Changing patch size: Mouse wheel changes the patch size from 1x1 to 9x9
- Load image: Click the load image button and choose desired image
- Restart: Click on the restart button. All points on the pad will be removed.
- Save result: Click on the save button. This will save the resulting colorization in a directory where the
image_file
was, along with the user input ab values. - Quit: Click on the quit button.
(3) Global Hints Network
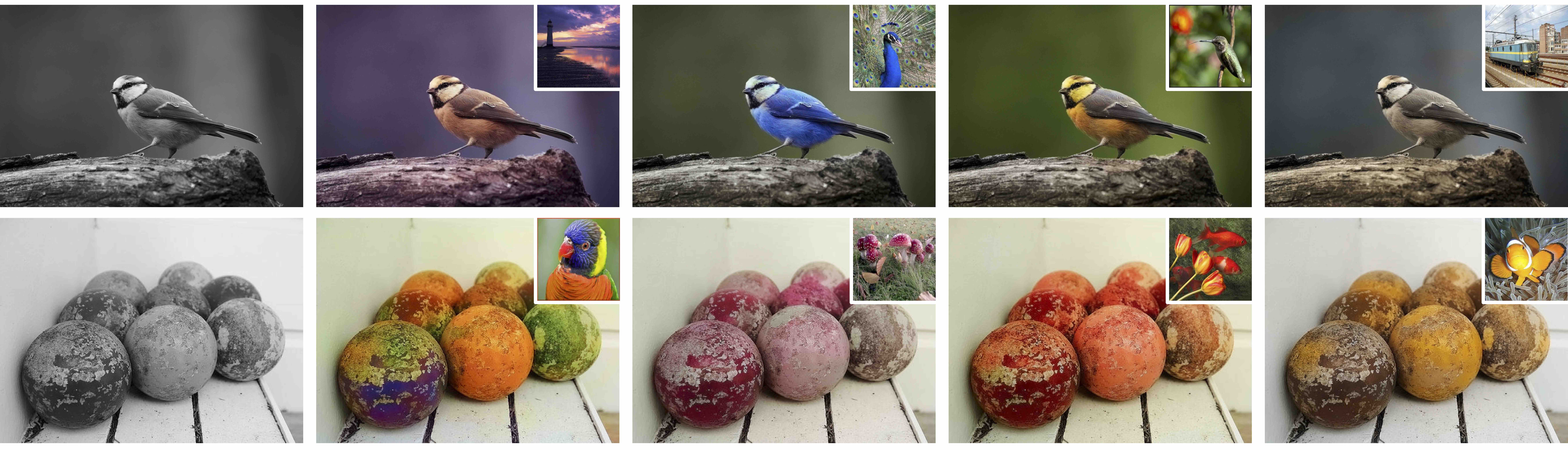
We include an example usage of our Global Hints Network, applied to global histogram transfer. We show its usage in an iPython notebook.
-
Add
./caffe_files
to yourPYTHONPATH
-
Run
ipython notebook
. Click on./DemoGlobalHistogramTransfer.ipynb
Installation
-
Install Caffe or PyTorch. The Caffe model is official. PyTorch is a reimplementation.
- Install Caffe: see the Caffe installation and Ubuntu installation document. Please compile the Caffe with the python layer support (set
WITH_PYTHON_LAYER=1
in theMakefile.config
) and build Caffe python library bymake pycaffe
.
You also need to add
pycaffe
to yourPYTHONPATH
. Usevi ~/.bashrc
to edit the environment variables.PYTHONPATH=/path/to/caffe/python:$PYTHONPATH LD_LIBRARY_PATH=/path/to/caffe/build/lib:$LD_LIBRARY_PATH
- Install PyTorch: see the PyTorch installation guide.
- Install Caffe: see the Caffe installation and Ubuntu installation document. Please compile the Caffe with the python layer support (set
-
Install scikit-image, scikit-learn, opencv, Qt5, and QDarkStyle pacakges:
# ./install/install_deps.sh
sudo pip install scikit-image
sudo pip install scikit-learn
sudo apt-get install python-opencv
sudo apt-get install qt5-default
sudo pip install qdarkstyle
For Conda users, type the following command lines (this may work for full Anaconda but not Miniconda):
# ./install/install_conda.sh
conda install -c anaconda protobuf ## photobuf
conda install -c anaconda scikit-learn=0.19.1 ## scikit-learn
conda install -c anaconda scikit-image=0.13.0 ## scikit-image
conda install -c menpo opencv=2.4.11 ## opencv
conda install -c anaconda qt ## qt5
conda install -c auto qdarkstyle ## qdarkstyle
For Docker users, please follow the Docker document.
-
Docker: [OSX Docker file] and [OSX Installation video] by @vbisbest, [Docker file 2] (by @sabrinawallner) based on DL Docker.
-
More installation help (by @SleepProgger).
Training
Please find a PyTorch reimplementation of the Local Hints Network training code in the colorization-pytorch repository.
Citation
If you use this code for your research, please cite our paper:
@article{zhang2017real,
title={Real-Time User-Guided Image Colorization with Learned Deep Priors},
author={Zhang, Richard and Zhu, Jun-Yan and Isola, Phillip and Geng, Xinyang and Lin, Angela S and Yu, Tianhe and Efros, Alexei A},
journal={ACM Transactions on Graphics (TOG)},
volume={9},
number={4},
year={2017},
publisher={ACM}
}
Cat Paper Collection
One of the authors objects to the inclusion of this list, due to an allergy. Another author objects on the basis that cats are silly creatures and this is a serious, scientific paper. However, if you love cats, and love reading cool graphics, vision, and learning papers, please check out the Cat Paper Collection: [Github] [Webpage]
Top Related Projects
Automatic colorization using deep neural networks. "Colorful Image Colorization." In ECCV, 2016.
A Deep Learning based project for colorizing and restoring old images (and video!)
The source code of 'Visual Attribute Transfer through Deep Image Analogy'.
Convert
designs to code with AI
Introducing Visual Copilot: A new AI model to turn Figma designs to high quality code using your components.
Try Visual Copilot