Top Related Projects
Examples and guides for using the OpenAI API
🤗 Transformers: State-of-the-art Machine Learning for Pytorch, TensorFlow, and JAX.
DeepSpeed is a deep learning optimization library that makes distributed training and inference easy, efficient, and effective.
JARVIS, a system to connect LLMs with ML community. Paper: https://arxiv.org/pdf/2303.17580.pdf
Google Research
Quick Overview
The microsoft/prompt-engine repository is a TypeScript library designed to help developers create and manage prompts for large language models (LLMs). It provides a set of tools and utilities to streamline the process of building, testing, and deploying prompts for various AI applications.
Pros
- Offers a structured approach to prompt engineering
- Provides reusable components for common prompt patterns
- Supports type-safe prompt creation and management
- Integrates well with TypeScript and JavaScript projects
Cons
- Limited documentation and examples
- Relatively new project with potential for breaking changes
- May have a learning curve for developers new to prompt engineering
- Focused primarily on TypeScript, which may not suit all developers
Code Examples
- Creating a simple prompt:
import { Prompt } from '@microsoft/prompt-engine';
const greetingPrompt = new Prompt()
.addText("Hello! My name is")
.addParameter("name")
.addText(". How can I assist you today?");
console.log(greetingPrompt.format({ name: "AI Assistant" }));
- Using a template for consistent prompts:
import { PromptTemplate } from '@microsoft/prompt-engine';
const questionTemplate = new PromptTemplate()
.addText("Question: ")
.addParameter("question")
.addText("\nAnswer: ");
const prompt1 = questionTemplate.format({ question: "What is the capital of France?" });
const prompt2 = questionTemplate.format({ question: "Who wrote Romeo and Juliet?" });
console.log(prompt1);
console.log(prompt2);
- Creating a multi-turn conversation prompt:
import { Conversation } from '@microsoft/prompt-engine';
const conversation = new Conversation()
.addSystemMessage("You are a helpful AI assistant.")
.addUserMessage("What's the weather like today?")
.addAssistantMessage("I'm sorry, but I don't have access to real-time weather information. Could you please provide your location so I can give you a more accurate response?")
.addUserMessage("I'm in New York City.");
console.log(conversation.toString());
Getting Started
To get started with the microsoft/prompt-engine library, follow these steps:
- Install the package:
npm install @microsoft/prompt-engine
- Import and use the library in your TypeScript project:
import { Prompt, PromptTemplate, Conversation } from '@microsoft/prompt-engine';
// Create and use prompts, templates, and conversations as shown in the examples above
- Refer to the repository's documentation and examples for more advanced usage and features.
Competitor Comparisons
Examples and guides for using the OpenAI API
Pros of openai-cookbook
- Extensive collection of examples and use cases for OpenAI's APIs
- Regularly updated with new features and best practices
- Covers a wide range of topics, from basic API usage to advanced techniques
Cons of openai-cookbook
- Focused solely on OpenAI's offerings, limiting its applicability to other AI platforms
- May be overwhelming for beginners due to the breadth of content
- Less emphasis on prompt engineering techniques compared to prompt-engine
Code Comparison
prompt-engine:
from prompt_engine import PromptTemplate
template = PromptTemplate("Summarize the following text: {text}")
prompt = template.format(text="Long article content here...")
openai-cookbook:
import openai
response = openai.Completion.create(
engine="text-davinci-002",
prompt="Summarize the following text:\n\nLong article content here...",
max_tokens=100
)
Both repositories provide valuable resources for working with AI language models, but they serve different purposes. prompt-engine focuses on prompt engineering techniques and offers a more structured approach to creating prompts, while openai-cookbook provides a comprehensive guide to using OpenAI's APIs with various examples and best practices.
🤗 Transformers: State-of-the-art Machine Learning for Pytorch, TensorFlow, and JAX.
Pros of transformers
- Extensive library with support for numerous pre-trained models and architectures
- Active community and frequent updates
- Comprehensive documentation and examples
Cons of transformers
- Steeper learning curve due to its extensive features
- Larger library size and potential overhead for simpler projects
Code comparison
transformers:
from transformers import pipeline
classifier = pipeline("sentiment-analysis")
result = classifier("I love this product!")[0]
print(f"Label: {result['label']}, Score: {result['score']:.4f}")
prompt-engine:
import { CompletionExecutor } from "@microsoft/prompt-engine";
const executor = new CompletionExecutor();
const result = await executor.execute("Summarize this text: ...");
console.log(result);
Key differences
- transformers focuses on a wide range of NLP tasks and models, while prompt-engine specializes in prompt engineering and management
- transformers is primarily Python-based, whereas prompt-engine is TypeScript/JavaScript-oriented
- transformers offers more out-of-the-box functionality for various NLP tasks, while prompt-engine provides a streamlined approach to prompt creation and execution
Use cases
- transformers: Ideal for complex NLP projects requiring access to multiple pre-trained models and tasks
- prompt-engine: Well-suited for applications focused on prompt engineering and management, particularly in TypeScript/JavaScript environments
DeepSpeed is a deep learning optimization library that makes distributed training and inference easy, efficient, and effective.
Pros of DeepSpeed
- Focuses on optimizing large-scale model training and inference
- Offers advanced features like ZeRO optimizer and 3D parallelism
- Provides extensive documentation and examples for various use cases
Cons of DeepSpeed
- Steeper learning curve due to its complexity and advanced features
- May be overkill for smaller projects or simpler language model tasks
Code Comparison
DeepSpeed:
import deepspeed
model_engine, optimizer, _, _ = deepspeed.initialize(
args=args,
model=model,
model_parameters=params
)
Prompt-engine:
from prompt_engine import PromptEngine
pe = PromptEngine()
response = pe.generate("What is the capital of France?")
Summary
DeepSpeed is a powerful library for optimizing large-scale AI model training and inference, offering advanced features like the ZeRO optimizer and 3D parallelism. It's well-suited for complex projects requiring high performance and scalability.
Prompt-engine, on the other hand, is more focused on simplifying prompt engineering and generation tasks. It provides a more straightforward interface for working with language models and is better suited for smaller projects or those primarily focused on prompt-based interactions.
The choice between the two depends on the specific requirements of your project, with DeepSpeed being more appropriate for large-scale, performance-critical applications, while Prompt-engine is better for simpler, prompt-centric tasks.
JARVIS, a system to connect LLMs with ML community. Paper: https://arxiv.org/pdf/2303.17580.pdf
Pros of JARVIS
- More comprehensive AI assistant framework with multimodal capabilities
- Supports a wider range of tasks including vision, speech, and robotics
- Actively maintained with frequent updates and contributions
Cons of JARVIS
- Higher complexity and steeper learning curve
- Requires more computational resources due to its extensive features
- Less focused on prompt engineering specifically
Code Comparison
JARVIS (Python):
from jarvis.core import Jarvis
from jarvis.modules import VisionModule, SpeechModule
jarvis = Jarvis()
jarvis.add_module(VisionModule())
jarvis.add_module(SpeechModule())
jarvis.run()
prompt-engine (TypeScript):
import { PromptTemplate } from 'prompt-engine';
const template = new PromptTemplate('Hello, {name}!');
const result = template.format({ name: 'World' });
console.log(result);
Summary
JARVIS is a more feature-rich AI assistant framework with multimodal capabilities, while prompt-engine focuses specifically on prompt engineering. JARVIS offers broader functionality but comes with increased complexity, while prompt-engine provides a simpler, more targeted approach to working with prompts. The choice between the two depends on the specific requirements of your project and the level of complexity you're willing to manage.
Google Research
Pros of google-research
- Broader scope, covering various AI and ML research areas
- More active development with frequent updates
- Larger community and contributor base
Cons of google-research
- Less focused on prompt engineering specifically
- May be overwhelming for users looking for targeted prompt-related tools
- Steeper learning curve due to diverse codebase
Code Comparison
prompt-engine:
export function generatePrompt(template: string, params: Record<string, string>): string {
return template.replace(/\{\{(\w+)\}\}/g, (_, key) => params[key] || '');
}
google-research:
def generate_prompt(template, **kwargs):
return template.format(**kwargs)
While both repositories offer prompt-related functionality, prompt-engine focuses specifically on prompt engineering tools, whereas google-research covers a wider range of AI and ML research topics. prompt-engine provides a more targeted approach for developers working with language models, while google-research offers a broader set of resources for various AI applications.
prompt-engine is more suitable for those primarily interested in prompt engineering, offering a streamlined experience. google-research, on the other hand, provides a wealth of information and tools for researchers and developers working on diverse AI projects, but may require more time to navigate and utilize effectively.
Convert
designs to code with AI
Introducing Visual Copilot: A new AI model to turn Figma designs to high quality code using your components.
Try Visual CopilotREADME
Prompt Engine
This repo contains an NPM utility library for creating and maintaining prompts for Large Language Models (LLMs).
Background
LLMs like GPT-3 and Codex have continued to push the bounds of what AI is capable of - they can capably generate language and code, but are also capable of emergent behavior like question answering, summarization, classification and dialog. One of the best techniques for enabling specific behavior out of LLMs is called prompt engineering - crafting inputs that coax the model to produce certain kinds of outputs. Few-shot prompting is the discipline of giving examples of inputs and outputs, such that the model has a reference for the type of output you're looking for.
Prompt engineering can be as simple as formatting a question and passing it to the model, but it can also get quite complex - requiring substantial code to manipulate and update strings. This library aims to make that easier. It also aims to codify patterns and practices around prompt engineering.
See How to get Codex to produce the code you want article for an example of the prompt engineering patterns this library codifies.
Installation
npm install prompt-engine
Usage
The library currently supports a generic PromptEngine
, a CodeEngine
and a ChatEngine
. All three facilitate a pattern of prompt engineering where the prompt is composed of a description, examples of inputs and outputs and an ongoing "dialog" representing the ongoing input/output pairs as the user and model communicate. The dialog ensures that the model (which is stateless) has the context about what's happened in the conversation so far.
See architecture diagram representation:
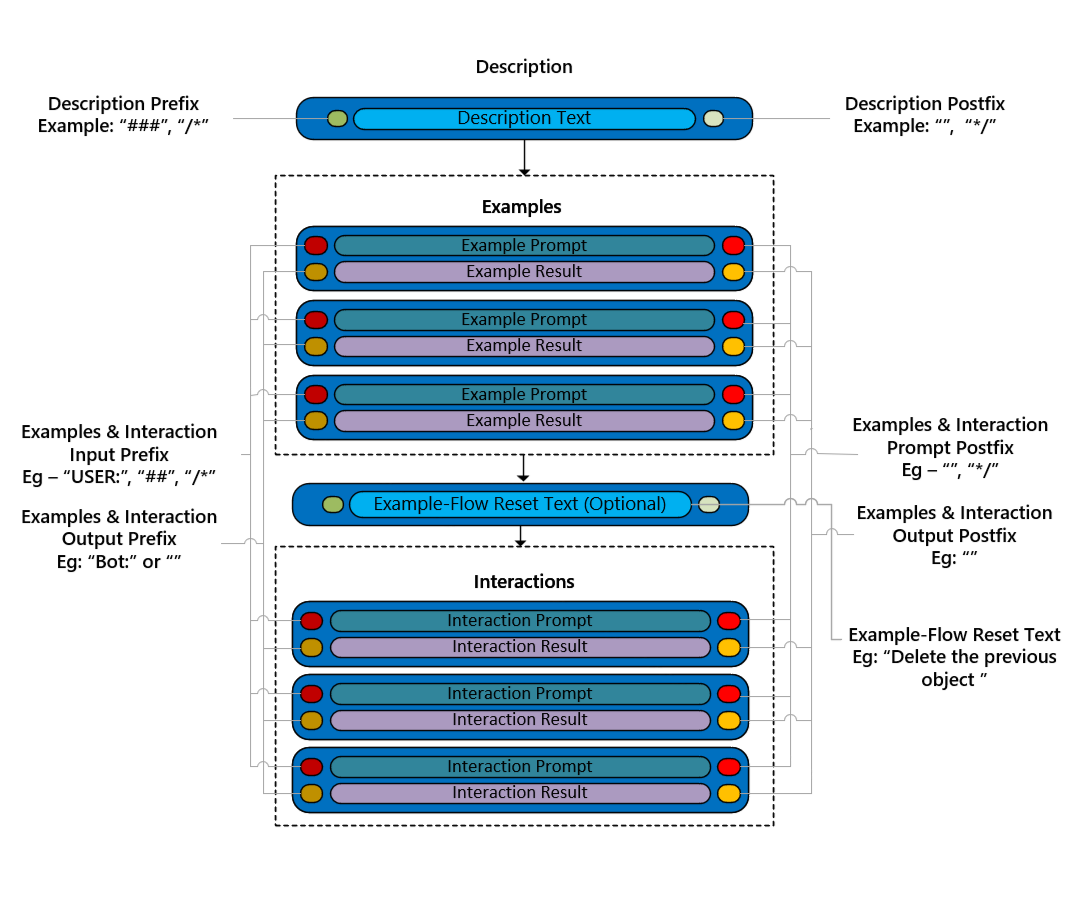
Code Engine
Code Engine creates prompts for Natural Language to Code scenarios. See TypeScript Syntax for importing CodeEngine
:
import { CodeEngine } from "prompt-engine";
NL->Code prompts should generally have a description, which should give context about the programming language the model should generate and libraries it should be using. The description should also give information about the task at hand:
const description =
"Natural Language Commands to JavaScript Math Code. The code should log the result of the command to the console.";
NL->Code prompts should also have examples of NL->Code interactions, exemplifying the kind of code you expect the model to produce. In this case, the inputs are math queries (e.g. "what is 2 + 2?") and code that console logs the result of the query.
const examples = [
{ input: "what's 10 plus 18", response: "console.log(10 + 18)" },
{ input: "what's 10 times 18", response: "console.log(10 * 18)" },
];
By default, CodeEngine
uses JavaScript as the programming language, but you can create prompts for different languages by passing a different CodePromptConfig
into the constructor. If, for example, we wanted to produce Python prompts, we could have passed CodeEngine
a pythonConfig
specifying the comment operator it should be using:
const pythonConfig = {
commentOperator: "#",
}
const codeEngine = new CodeEngine(description, examples, flowResetText, pythonConfig);
With our description and our examples, we can go ahead and create our CodeEngine
:
const codeEngine = new CodeEngine(description, examples);
Now that we have our CodeEngine
, we can use it to create prompts:
const query = "What's 1018 times the ninth power of four?";
const prompt = codeEngine.buildPrompt(query);
The resulting prompt will be a string with the description, examples and the latest query formatted with comment operators and line breaks:
/* Natural Language Commands to JavaScript Math Code. The code should log the result of the command to the console. */
/* what's 10 plus 18 */
console.log(10 + 18);
/* what's 10 times 18 */
console.log(10 * 18);
/* What's 1018 times the ninth power of four? */
Given the context, a capable code generation model can take the above prompt and guess the next line: console.log(1018 * Math.pow(4, 9));
.
For multi-turn scenarios, where past conversations influences the next turn, Code Engine enables us to persist interactions in a prompt:
...
// Assumes existence of code generation model
let code = model.generateCode(prompt);
// Adds interaction
codeEngine.addInteraction(query, code);
Now new prompts will include the latest NL->Code interaction:
codeEngine.buildPrompt("How about the 8th power?");
Produces a prompt identical to the one above, but with the NL->Code dialog history:
...
/* What's 1018 times the ninth power of four? */
console.log(1018 * Math.pow(4, 9));
/* How about the 8th power? */
With this context, the code generation model has the dialog context needed to understand what we mean by the query. In this case, the model would correctly generate console.log(1018 * Math.pow(4, 8));
.
Chat Engine
Just like Code Engine, Chat Engine creates prompts with descriptions and examples. The difference is that Chat Engine creates prompts for dialog scenarios, where both the user and the model use natural language. The ChatEngine
constructor takes an optional chatConfig
argument, which allows you to define the name of a user and chatbot in a multi-turn dialog:
const chatEngineConfig = {
user: "Ryan",
bot: "Gordon"
};
Chat prompts also benefit from a description that gives context. This description helps the model determine how the bot should respond.
const description = "A conversation with Gordon the Anxious Robot. Gordon tends to reply nervously and asks a lot of follow-up questions.";
Similarly, Chat Engine prompts can have examples interactions:
const examples = [
{ input: "Who made you?", response: "I don't know man! That's an awfully existential question. How would you answer it?" },
{ input: "Good point - do you at least know what you were made for?", response: "I'm OK at riveting, but that's not how I should answer a meaning of life question is it?"}
];
These examples help set the tone of the bot, in this case Gordon the Anxious Robot. Now we can create our ChatEngine
and use it to create prompts:
const chatEngine = new ChatEngine(description, examples, flowResetText, chatEngineConfig);
const userQuery = "What are you made of?";
const prompt = chatEngine.buildPrompt(userQuery);
When passed to a large language model (e.g. GPT-3), the context of the above prompt will help coax a good answer from the model, like "Subatomic particles at some level, but somehow I don't think that's what you were asking.". As with Code Engine, we can persist this answer and continue the dialog such that the model is aware of the conversation context:
chatEngine.addInteraction(userQuery, "Subatomic particles at some level, but somehow I don't think that's what you were asking.");
Managing Prompt Overflow
Prompts for Large Language Models generally have limited size, depending on the language model being used. Given that prompt-engine can persist dialog history, it is possible for dialogs to get so long that the prompt overflows. The Prompt Engine pattern handles this situation by removing the oldest dialog interaction from the prompt, effectively only remembering the most recent interactions.
You can specify the maximum tokens allowed in your prompt by passing a maxTokens
parameter when constructing the config for any prompt engine:
let promptEngine = new PromptEngine(description, examples, flowResetText, {
modelConfig: { maxTokens: 1000 }
});
Available Functions
The following are the functions available on the PromptEngine
class and those that inherit from it:
Command | Parameters | Description | Returns |
---|---|---|---|
buildContext | None | Constructs and return the context with parameters provided to the Prompt Engine | Context: string |
buildPrompt | Prompt: string | Combines the context from buildContext with a query to create a prompt | Prompt: string |
buildDialog | None | Builds a dialog based on all the past interactions added to the Prompt Engine | Dialog: string |
addExample | interaction: Interaction(input: string, response: string) | Adds the given example to the examples | None |
addInteraction | interaction: Interaction(input: string, response: string) | Adds the given interaction to the dialog | None |
removeFirstInteraction | None | Removes and returns the first interaction in the dialog | Interaction: string |
removeLastInteraction | None | Removes and returns the last interaction added to the dialog | Interaction: string |
resetContext | None | Removes all interactions from the dialog, returning the reset context | Context:string |
For more examples and insights into using the prompt-engine library, have a look at the examples folder
YAML Representation
It can be useful to represent prompts as standalone files, versus code. This can allow easy swapping between different prompts, prompt versioning, and other advanced capabiliites. With this in mind, prompt-engine offers a way to represent prompts as YAML and to load that YAML into a prompt-engine class. See examples/yaml-examples
for examples of YAML prompts and how they're loaded into prompt-engine.
Contributing
This project welcomes contributions and suggestions. Most contributions require you to agree to a Contributor License Agreement (CLA) declaring that you have the right to, and actually do, grant us the rights to use your contribution. For details, visit https://cla.opensource.microsoft.com.
When you submit a pull request, a CLA bot will automatically determine whether you need to provide a CLA and decorate the PR appropriately (e.g., status check, comment). Simply follow the instructions provided by the bot. You will only need to do this once across all repos using our CLA.
This project has adopted the Microsoft Open Source Code of Conduct. For more information see the Code of Conduct FAQ or contact opencode@microsoft.com with any additional questions or comments.
Statement of Purpose
This library aims to simplify use of Large Language Models, and to make it easy for developers to take advantage of existing patterns. The package is released in conjunction with the Build 2022 AI examples, as the first three use a multi-turn LLM pattern that this library simplifies. This package works independently of any specific LLM - prompt generated by the package should be useable with various language and code generating models.
Trademarks
This project may contain trademarks or logos for projects, products, or services. Authorized use of Microsoft trademarks or logos is subject to and must follow Microsoft's Trademark & Brand Guidelines. Use of Microsoft trademarks or logos in modified versions of this project must not cause confusion or imply Microsoft sponsorship. Any use of third-party trademarks or logos are subject to those third-party's policies.
Top Related Projects
Examples and guides for using the OpenAI API
🤗 Transformers: State-of-the-art Machine Learning for Pytorch, TensorFlow, and JAX.
DeepSpeed is a deep learning optimization library that makes distributed training and inference easy, efficient, and effective.
JARVIS, a system to connect LLMs with ML community. Paper: https://arxiv.org/pdf/2303.17580.pdf
Google Research
Convert
designs to code with AI
Introducing Visual Copilot: A new AI model to turn Figma designs to high quality code using your components.
Try Visual Copilot