mmtracking
OpenMMLab Video Perception Toolbox. It supports Video Object Detection (VID), Multiple Object Tracking (MOT), Single Object Tracking (SOT), Video Instance Segmentation (VIS) with a unified framework.
Top Related Projects
Detectron2 is a platform for object detection, segmentation and other visual recognition tasks.
YOLOv5 🚀 in PyTorch > ONNX > CoreML > TFLite
YOLOv4 / Scaled-YOLOv4 / YOLO - Neural Networks for Object Detection (Windows and Linux version of Darknet )
Mask R-CNN for object detection and instance segmentation on Keras and TensorFlow
Models and examples built with TensorFlow
FAIR's research platform for object detection research, implementing popular algorithms like Mask R-CNN and RetinaNet.
Quick Overview
MMTracking is an open-source toolbox for video perception tasks, including single object tracking, multiple object tracking, and video object detection. It is built on PyTorch and is part of the OpenMMLab project, providing a unified framework for various tracking algorithms and datasets.
Pros
- Comprehensive collection of state-of-the-art tracking algorithms
- Modular design allowing easy customization and extension
- Well-documented and actively maintained
- Supports both single and multiple object tracking tasks
Cons
- Steep learning curve for beginners due to the complexity of tracking algorithms
- Requires significant computational resources for training and inference
- Limited support for real-time tracking applications
- Dependency on other OpenMMLab projects may increase setup complexity
Code Examples
- Single Object Tracking:
from mmtrack.apis import init_model, inference_sot
# Initialize the model
config = 'configs/sot/siamese_rpn/siamese_rpn_r50_20e_lasot.py'
checkpoint = 'checkpoints/siamese_rpn_r50_20e_lasot_20220420_181845-dd0f151e.pth'
model = init_model(config, checkpoint, device='cuda:0')
# Perform inference
video_path = 'demo/demo.mp4'
result = inference_sot(model, video_path, frame_id=0, init_bbox=[100, 100, 200, 200])
- Multiple Object Tracking:
from mmtrack.apis import init_model, inference_mot
# Initialize the model
config = 'configs/mot/deepsort/deepsort_faster-rcnn_fpn_4e_mot17-private-half.py'
checkpoint = 'checkpoints/deepsort_faster-rcnn_fpn_4e_mot17-private-half_20210517_001210-d9ab3169.pth'
model = init_model(config, checkpoint, device='cuda:0')
# Perform inference
video_path = 'demo/demo.mp4'
result = inference_mot(model, video_path, frame_id=0)
- Video Object Detection:
from mmtrack.apis import init_model, inference_vid
# Initialize the model
config = 'configs/vid/selsa/selsa_faster_rcnn_r50_dc5_1x_imagenetvid.py'
checkpoint = 'checkpoints/selsa_faster_rcnn_r50_dc5_1x_imagenetvid_20201218_172724-aa961bcc.pth'
model = init_model(config, checkpoint, device='cuda:0')
# Perform inference
video_path = 'demo/demo.mp4'
result = inference_vid(model, video_path)
Getting Started
- Install MMTracking:
pip install openmim
mim install mmtrack
- Download a pre-trained model:
mim download mmtrack --config siamese_rpn_r50_20e_lasot --dest .
- Run inference:
from mmtrack.apis import init_model, inference_sot
config = 'siamese_rpn_r50_20e_lasot.py'
checkpoint = 'siamese_rpn_r50_20e_lasot_20220420_181845-dd0f151e.pth'
model = init_model(config, checkpoint, device='cuda:0')
video_path = 'path/to/your/video.mp4'
result = inference_sot(model, video_path, frame_id=0, init_bbox=[100, 100, 200, 200])
Competitor Comparisons
Detectron2 is a platform for object detection, segmentation and other visual recognition tasks.
Pros of Detectron2
- More comprehensive object detection framework with broader scope
- Larger community and more extensive documentation
- Better performance and faster training on some benchmarks
Cons of Detectron2
- Steeper learning curve for beginners
- Less focused on video-based tasks like tracking
- Requires more computational resources for training
Code Comparison
MMTracking example:
from mmtrack.apis import init_model, inference_mot
config_file = 'configs/mot/deepsort/deepsort_faster-rcnn_fpn_4e_mot17-private-half.py'
checkpoint_file = 'checkpoints/deepsort_faster-rcnn_fpn_4e_mot17-private-half_20210517_001732-d066fa2a.pth'
model = init_model(config_file, checkpoint_file, device='cuda:0')
result = inference_mot(model, video_path)
Detectron2 example:
from detectron2.config import get_cfg
from detectron2.engine import DefaultPredictor
cfg = get_cfg()
cfg.merge_from_file("config.yaml")
cfg.MODEL.WEIGHTS = "model_weights.pth"
predictor = DefaultPredictor(cfg)
outputs = predictor(image)
YOLOv5 🚀 in PyTorch > ONNX > CoreML > TFLite
Pros of YOLOv5
- Simpler architecture and easier to use for beginners
- Faster inference speed, especially on edge devices
- More extensive documentation and community support
Cons of YOLOv5
- Limited to object detection tasks, less versatile than mmtracking
- May have lower accuracy compared to more complex models in mmtracking
- Fewer pre-trained models and datasets available out-of-the-box
Code Comparison
YOLOv5:
import torch
model = torch.hub.load('ultralytics/yolov5', 'yolov5s')
results = model('image.jpg')
results.print()
mmtracking:
from mmtrack.apis import init_model, inference_mot
config_file = 'configs/mot/deepsort/deepsort_faster-rcnn_fpn_4e_mot17-private-half.py'
checkpoint_file = 'checkpoints/deepsort_faster-rcnn_fpn_4e_mot17-private-half_20210803_001244-d025f200.pth'
model = init_model(config_file, checkpoint_file, device='cuda:0')
result = inference_mot(model, 'demo/demo.mp4', output_file='output.mp4')
YOLOv5 offers a more straightforward API for quick object detection tasks, while mmtracking provides a more comprehensive framework for various tracking tasks with more complex configuration options.
YOLOv4 / Scaled-YOLOv4 / YOLO - Neural Networks for Object Detection (Windows and Linux version of Darknet )
Pros of darknet
- Lightweight and efficient implementation in C
- Extensive support for YOLO object detection models
- Easy to use command-line interface for training and inference
Cons of darknet
- Limited support for other computer vision tasks beyond object detection
- Less modular architecture compared to mmtracking
- Fewer pre-trained models and datasets available out-of-the-box
Code comparison
darknet:
network *net = parse_network_cfg(cfgfile);
load_weights(net, weightfile);
detection_layer l = net->layers[net->n-1];
mmtracking:
from mmtrack.apis import init_model, inference_mot
config_file = 'configs/mot/deepsort/deepsort_faster-rcnn_fpn_4e_mot17-private-half.py'
checkpoint_file = 'checkpoints/deepsort_faster-rcnn_fpn_4e_mot17-private-half_20210517_001732-d066c24b.pth'
model = init_model(config_file, checkpoint_file, device='cuda:0')
result = inference_mot(model, video_path)
The darknet code showcases its C-based implementation, while mmtracking demonstrates its Python-based, modular approach with easy-to-use APIs for various tracking tasks.
Mask R-CNN for object detection and instance segmentation on Keras and TensorFlow
Pros of Mask_RCNN
- Focused specifically on instance segmentation, providing a simpler and more specialized implementation
- Well-documented with extensive tutorials and examples for ease of use
- Includes pre-trained models on COCO dataset, enabling quick start for transfer learning
Cons of Mask_RCNN
- Limited to instance segmentation tasks, lacking support for other computer vision tasks
- Less frequently updated compared to mmtracking, potentially missing recent advancements
- May require more manual configuration for custom datasets or tasks
Code Comparison
Mask_RCNN:
import mrcnn.model as modellib
model = modellib.MaskRCNN(mode="inference", config=config, model_dir=MODEL_DIR)
model.load_weights(COCO_MODEL_PATH, by_name=True)
results = model.detect([image], verbose=1)
mmtracking:
from mmtrack.apis import init_model, inference_mot
config_file = 'configs/mot/deepsort/deepsort_faster-rcnn_fpn_4e_mot17-private-half.py'
checkpoint_file = 'checkpoints/deepsort_faster-rcnn_fpn_4e_mot17-private-half_20210517_001732-a36a1f0c.pth'
model = init_model(config_file, checkpoint_file, device='cuda:0')
result = inference_mot(model, video_file, frame_rate=30)
Models and examples built with TensorFlow
Pros of models
- Broader scope, covering various ML tasks beyond just tracking
- Larger community and more frequent updates
- Official TensorFlow implementation, ensuring compatibility
Cons of models
- More complex structure, potentially harder to navigate
- Less specialized for tracking tasks compared to mmtracking
- May require more setup and configuration for specific use cases
Code comparison
mmtracking:
from mmtrack.apis import init_model, inference_mot
config_file = 'configs/mot/deepsort/deepsort_faster-rcnn_fpn_4e_mot17-private-half.py'
checkpoint_file = 'checkpoints/deepsort_faster-rcnn_fpn_4e_mot17-private-half_20210517_001210-d9ab3169.pth'
model = init_model(config_file, checkpoint_file, device='cuda:0')
models:
import tensorflow as tf
from object_detection import model_lib_v2
pipeline_config = 'pipeline.config'
model_dir = 'path/to/model_dir'
config = tf.estimator.RunConfig(model_dir=model_dir)
train_and_eval_dict = model_lib_v2.create_estimator_and_inputs(
run_config=config,
pipeline_config_path=pipeline_config)
FAIR's research platform for object detection research, implementing popular algorithms like Mask R-CNN and RetinaNet.
Pros of Detectron
- More established and widely adopted in the computer vision community
- Extensive documentation and tutorials for easier onboarding
- Broader scope, covering various object detection and segmentation tasks
Cons of Detectron
- Less focused on video-based tasks and multi-object tracking
- May require more setup and configuration for specific tracking tasks
- Not as actively maintained as mmtracking (last update was in 2019)
Code Comparison
mmtracking:
from mmtrack.apis import init_model, inference_mot
config_file = 'configs/mot/deepsort/deepsort_faster-rcnn_fpn_4e_mot17-private-half.py'
checkpoint_file = 'checkpoints/deepsort_faster-rcnn_fpn_4e_mot17-private-half_20210517_001732-d066fa53.pth'
model = init_model(config_file, checkpoint_file, device='cuda:0')
result = inference_mot(model, video_path, frame_rate=30)
Detectron:
from detectron2.config import get_cfg
from detectron2.engine import DefaultPredictor
cfg = get_cfg()
cfg.merge_from_file("configs/COCO-Detection/faster_rcnn_R_50_FPN_3x.yaml")
cfg.MODEL.WEIGHTS = "detectron2://COCO-Detection/faster_rcnn_R_50_FPN_3x/137849458/model_final_280758.pkl"
predictor = DefaultPredictor(cfg)
outputs = predictor(image)
Convert
designs to code with AI
Introducing Visual Copilot: A new AI model to turn Figma designs to high quality code using your components.
Try Visual CopilotREADME

ðDocumentation | ð ï¸Installation | ðModel Zoo | ðUpdate News | ð¤Reporting Issues
English | ç®ä½ä¸æ
Introduction
MMTracking is an open source video perception toolbox by PyTorch. It is a part of OpenMMLab project.
The master branch works with PyTorch1.5+.
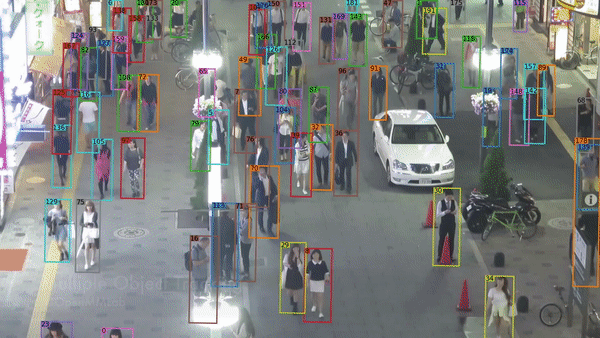
Major features
-
The First Unified Video Perception Platform
We are the first open source toolbox that unifies versatile video perception tasks include video object detection, multiple object tracking, single object tracking and video instance segmentation.
-
Modular Design
We decompose the video perception framework into different components and one can easily construct a customized method by combining different modules.
-
Simple, Fast and Strong
Simple: MMTracking interacts with other OpenMMLab projects. It is built upon MMDetection that we can capitalize any detector only through modifying the configs.
Fast: All operations run on GPUs. The training and inference speeds are faster than or comparable to other implementations.
Strong: We reproduce state-of-the-art models and some of them even outperform the official implementations.
What's New
We release MMTracking 1.0.0rc0, the first version of MMTracking 1.x.
Built upon the new training engine, MMTracking 1.x unifies the interfaces of datasets, models, evaluation, and visualization.
We also support more methods in MMTracking 1.x, such as StrongSORT for MOT, Mask2Former for VIS, PrDiMP for SOT.
Please refer to dev-1.x branch for the using of MMTracking 1.x.
Installation
Please refer to install.md for install instructions.
Getting Started
Please see dataset.md and quick_run.md for the basic usage of MMTracking.
A Colab tutorial is provided. You may preview the notebook here or directly run it on Colab.
There are also usage tutorials, such as learning about configs, an example about detailed description of vid config, an example about detailed description of mot config, an example about detailed description of sot config, customizing dataset, customizing data pipeline, customizing vid model, customizing mot model, customizing sot model, customizing runtime settings and useful tools.
Benchmark and model zoo
Results and models are available in the model zoo.
Video Object Detection
Supported Methods
- DFF (CVPR 2017)
- FGFA (ICCV 2017)
- SELSA (ICCV 2019)
- Temporal RoI Align (AAAI 2021)
Supported Datasets
Single Object Tracking
Supported Methods
- SiameseRPN++ (CVPR 2019)
- STARK (ICCV 2021)
- MixFormer (CVPR 2022)
- PrDiMP (CVPR2020) (WIP)
Supported Datasets
Multi-Object Tracking
Supported Methods
- SORT/DeepSORT (ICIP 2016/2017)
- Tracktor (ICCV 2019)
- QDTrack (CVPR 2021)
- ByteTrack (ECCV 2022)
- OC-SORT (arXiv 2022)
Supported Datasets
Video Instance Segmentation
Supported Methods
- MaskTrack R-CNN (ICCV 2019)
Supported Datasets
Contributing
We appreciate all contributions to improve MMTracking. Please refer to CONTRIBUTING.md for the contributing guideline and this discussion for development roadmap.
Acknowledgement
MMTracking is an open source project that welcome any contribution and feedback. We wish that the toolbox and benchmark could serve the growing research community by providing a flexible as well as standardized toolkit to reimplement existing methods and develop their own new video perception methods.
Citation
If you find this project useful in your research, please consider cite:
@misc{mmtrack2020,
title={{MMTracking: OpenMMLab} video perception toolbox and benchmark},
author={MMTracking Contributors},
howpublished = {\url{https://github.com/open-mmlab/mmtracking}},
year={2020}
}
License
This project is released under the Apache 2.0 license.
Projects in OpenMMLab
- MMCV: OpenMMLab foundational library for computer vision.
- MIM: MIM installs OpenMMLab packages.
- MMClassification: OpenMMLab image classification toolbox and benchmark.
- MMDetection: OpenMMLab detection toolbox and benchmark.
- MMDetection3D: OpenMMLab's next-generation platform for general 3D object detection.
- MMYOLO: OpenMMLab YOLO series toolbox and benchmark.
- MMRotate: OpenMMLab rotated object detection toolbox and benchmark.
- MMSegmentation: OpenMMLab semantic segmentation toolbox and benchmark.
- MMOCR: OpenMMLab text detection, recognition and understanding toolbox.
- MMPose: OpenMMLab pose estimation toolbox and benchmark.
- MMHuman3D: OpenMMLab 3D human parametric model toolbox and benchmark.
- MMSelfSup: OpenMMLab self-supervised learning Toolbox and Benchmark.
- MMRazor: OpenMMLab Model Compression Toolbox and Benchmark.
- MMFewShot: OpenMMLab FewShot Learning Toolbox and Benchmark.
- MMAction2: OpenMMLab's next-generation action understanding toolbox and benchmark.
- MMTracking: OpenMMLab video perception toolbox and benchmark.
- MMFlow: OpenMMLab optical flow toolbox and benchmark.
- MMEditing: OpenMMLab image and video editing toolbox.
- MMGeneration: OpenMMLab Generative Model toolbox and benchmark.
- MMDeploy: OpenMMlab deep learning model deployment toolset.
Top Related Projects
Detectron2 is a platform for object detection, segmentation and other visual recognition tasks.
YOLOv5 🚀 in PyTorch > ONNX > CoreML > TFLite
YOLOv4 / Scaled-YOLOv4 / YOLO - Neural Networks for Object Detection (Windows and Linux version of Darknet )
Mask R-CNN for object detection and instance segmentation on Keras and TensorFlow
Models and examples built with TensorFlow
FAIR's research platform for object detection research, implementing popular algorithms like Mask R-CNN and RetinaNet.
Convert
designs to code with AI
Introducing Visual Copilot: A new AI model to turn Figma designs to high quality code using your components.
Try Visual Copilot