Top Related Projects
Quick Overview
Xarray is an open-source Python library that introduces labeled multi-dimensional arrays and datasets. It extends NumPy and pandas to work with labeled datasets, making it easier to work with multi-dimensional scientific data. Xarray is particularly useful for working with netCDF files and other gridded data formats common in geoscience and climate science.
Pros
- Provides intuitive, pandas-like interface for multi-dimensional data
- Powerful indexing and computation capabilities with labeled dimensions
- Seamless integration with dask for parallel computing and out-of-memory datasets
- Built-in plotting functionality and interoperability with matplotlib
Cons
- Steeper learning curve compared to NumPy for users new to labeled data
- Can be slower than pure NumPy operations for simple array manipulations
- Limited support for some specialized scientific data formats
- Occasional API changes in major versions may require code updates
Code Examples
- Creating and manipulating a DataArray:
import xarray as xr
import numpy as np
# Create a DataArray with labeled dimensions
data = xr.DataArray(
np.random.rand(4, 3),
dims=("x", "y"),
coords={"x": [10, 20, 30, 40], "y": ["a", "b", "c"]}
)
# Perform operations using dimension names
result = data.mean(dim="x")
- Working with multi-dimensional datasets:
# Create a Dataset with multiple variables
ds = xr.Dataset(
{
"temperature": (("time", "lat", "lon"), np.random.rand(5, 10, 15)),
"precipitation": (("time", "lat", "lon"), np.random.rand(5, 10, 15)),
},
coords={
"time": pd.date_range("2021-01-01", periods=5),
"lat": np.linspace(0, 90, 10),
"lon": np.linspace(-180, 180, 15),
}
)
# Select data for a specific time and latitude range
subset = ds.sel(time="2021-01-03", lat=slice(30, 60))
- Plotting with xarray:
import matplotlib.pyplot as plt
# Create a contour plot of temperature data
ds.temperature.isel(time=0).plot.contourf(x="lon", y="lat")
plt.title("Temperature Distribution")
plt.show()
Getting Started
To get started with xarray, first install it using pip:
pip install xarray
Then, import the library and create a simple DataArray:
import xarray as xr
import numpy as np
data = xr.DataArray(
np.random.rand(3, 4),
dims=("x", "y"),
coords={"x": [0, 1, 2], "y": [10, 20, 30, 40]}
)
print(data)
This will create a 2D array with labeled dimensions and coordinates, demonstrating the basic structure of xarray objects.
Competitor Comparisons
Flexible and powerful data analysis / manipulation library for Python, providing labeled data structures similar to R data.frame objects, statistical functions, and much more
Pros of pandas
- Excellent for handling tabular data with heterogeneous types
- Robust time series functionality and date range generation
- Extensive data manipulation and analysis capabilities
Cons of pandas
- Less suitable for multi-dimensional data
- Memory-intensive for large datasets
- Limited support for labeled dimensions
Code comparison
pandas:
import pandas as pd
df = pd.DataFrame({'A': [1, 2, 3], 'B': [4, 5, 6]})
result = df.groupby('A').sum()
xarray:
import xarray as xr
ds = xr.Dataset({'A': ('x', [1, 2, 3]), 'B': ('x', [4, 5, 6])})
result = ds.groupby('A').sum()
pandas excels at handling tabular data and time series analysis, making it ideal for financial data and general-purpose data manipulation. It offers powerful data alignment and merging capabilities.
xarray, on the other hand, is designed for working with multi-dimensional labeled arrays, making it more suitable for scientific and geospatial data. It provides better support for handling large datasets with named dimensions and coordinates.
While pandas is more widely used and has a larger ecosystem, xarray offers advantages when dealing with multi-dimensional data and provides better integration with scientific computing libraries like dask for parallel computing.
A high-level plotting API for pandas, dask, xarray, and networkx built on HoloViews
Pros of hvplot
- Simpler and more intuitive API for creating interactive plots
- Seamless integration with various data structures (pandas, xarray, dask)
- Built-in support for geographic plotting and interactive widgets
Cons of hvplot
- Less flexible for complex, custom visualizations
- Smaller community and ecosystem compared to xarray
- Dependent on HoloViews and Bokeh, which may add complexity
Code Comparison
hvplot:
import hvplot.xarray
import xarray as xr
ds = xr.tutorial.open_dataset('air_temperature')
ds.air.hvplot.quadmesh(x='lon', y='lat', cmap='viridis')
xarray:
import xarray as xr
import matplotlib.pyplot as plt
ds = xr.tutorial.open_dataset('air_temperature')
ds.air.plot(x='lon', y='lat', cmap='viridis')
plt.show()
Summary
hvplot provides a higher-level, more user-friendly interface for creating interactive plots, especially when working with xarray datasets. It offers built-in support for various data types and geographic plotting. However, xarray's plotting capabilities, while requiring more code, offer greater flexibility for customization and are part of a larger, more established ecosystem. The choice between the two depends on the specific visualization needs and the desired level of interactivity.
Convert
designs to code with AI
Introducing Visual Copilot: A new AI model to turn Figma designs to high quality code using your components.
Try Visual CopilotREADME
xarray: N-D labeled arrays and datasets
xarray (pronounced "ex-array", formerly known as xray) is an open source project and Python package that makes working with labelled multi-dimensional arrays simple, efficient, and fun!
Xarray introduces labels in the form of dimensions, coordinates and attributes on top of raw NumPy-like arrays, which allows for a more intuitive, more concise, and less error-prone developer experience. The package includes a large and growing library of domain-agnostic functions for advanced analytics and visualization with these data structures.
Xarray was inspired by and borrows heavily from pandas, the popular data analysis package focused on labelled tabular data. It is particularly tailored to working with netCDF files, which were the source of xarray's data model, and integrates tightly with dask for parallel computing.
Why xarray?
Multi-dimensional (a.k.a. N-dimensional, ND) arrays (sometimes called "tensors") are an essential part of computational science. They are encountered in a wide range of fields, including physics, astronomy, geoscience, bioinformatics, engineering, finance, and deep learning. In Python, NumPy provides the fundamental data structure and API for working with raw ND arrays. However, real-world datasets are usually more than just raw numbers; they have labels which encode information about how the array values map to locations in space, time, etc.
Xarray doesn't just keep track of labels on arrays -- it uses them to provide a powerful and concise interface. For example:
- Apply operations over dimensions by name:
x.sum('time')
. - Select values by label instead of integer location:
x.loc['2014-01-01']
orx.sel(time='2014-01-01')
. - Mathematical operations (e.g.,
x - y
) vectorize across multiple dimensions (array broadcasting) based on dimension names, not shape. - Flexible split-apply-combine operations with groupby:
x.groupby('time.dayofyear').mean()
. - Database like alignment based on coordinate labels that smoothly
handles missing values:
x, y = xr.align(x, y, join='outer')
. - Keep track of arbitrary metadata in the form of a Python dictionary:
x.attrs
.
Documentation
Learn more about xarray in its official documentation at https://docs.xarray.dev/.
Try out an interactive Jupyter notebook.
Contributing
You can find information about contributing to xarray at our Contributing page.
Get in touch
- Ask usage questions ("How do I?") on GitHub Discussions.
- Report bugs, suggest features or view the source code on GitHub.
- For less well defined questions or ideas, or to announce other projects of interest to xarray users, use the mailing list.
NumFOCUS
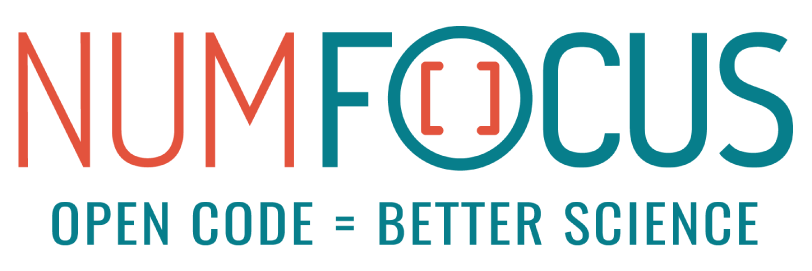
Xarray is a fiscally sponsored project of NumFOCUS, a nonprofit dedicated to supporting the open source scientific computing community. If you like Xarray and want to support our mission, please consider making a donation to support our efforts.
History
Xarray is an evolution of an internal tool developed at The Climate Corporation. It was originally written by Climate Corp researchers Stephan Hoyer, Alex Kleeman and Eugene Brevdo and was released as open source in May 2014. The project was renamed from "xray" in January 2016. Xarray became a fiscally sponsored project of NumFOCUS in August 2018.
Contributors
Thanks to our many contributors!
License
Copyright 2014-2024, xarray Developers
Licensed under the Apache License, Version 2.0 (the "License"); you may not use this file except in compliance with the License. You may obtain a copy of the License at
https://www.apache.org/licenses/LICENSE-2.0
Unless required by applicable law or agreed to in writing, software distributed under the License is distributed on an "AS IS" BASIS, WITHOUT WARRANTIES OR CONDITIONS OF ANY KIND, either express or implied. See the License for the specific language governing permissions and limitations under the License.
Xarray bundles portions of pandas, NumPy and Seaborn, all of which are available under a "3-clause BSD" license:
- pandas:
setup.py
,xarray/util/print_versions.py
- NumPy:
xarray/core/npcompat.py
- Seaborn:
_determine_cmap_params
inxarray/core/plot/utils.py
Xarray also bundles portions of CPython, which is available under the
"Python Software Foundation License" in xarray/core/pycompat.py
.
Xarray uses icons from the icomoon package (free version), which is available under the "CC BY 4.0" license.
The full text of these licenses are included in the licenses directory.
Top Related Projects
Convert
designs to code with AI
Introducing Visual Copilot: A new AI model to turn Figma designs to high quality code using your components.
Try Visual Copilot