weaviate
Weaviate is an open-source vector database that stores both objects and vectors, allowing for the combination of vector search with structured filtering with the fault tolerance and scalability of a cloud-native database.
Top Related Projects
Qdrant - High-performance, massive-scale Vector Database and Vector Search Engine for the next generation of AI. Also available in the cloud https://cloud.qdrant.io/
Milvus is a high-performance, cloud-native vector database built for scalable vector ANN search
the AI-native open-source embedding database
Free and Open Source, Distributed, RESTful Search Engine
AI + Data, online. https://vespa.ai
Quick Overview
Weaviate is an open-source vector database designed to store both objects and vectors, enabling semantic search, question answering, classification, and other machine learning tasks. It provides a cloud-native database with a GraphQL interface, making it easy to integrate with various AI and ML models.
Pros
- Scalable and cloud-native architecture
- Supports multiple vector index types for different use cases
- Provides a GraphQL API for easy integration and querying
- Offers multi-modal search capabilities (text, images, audio)
Cons
- Steep learning curve for beginners
- Limited support for traditional relational database operations
- Requires careful consideration of vector embedding choices
- Resource-intensive for large-scale deployments
Code Examples
- Creating a schema:
import weaviate
client = weaviate.Client("http://localhost:8080")
schema = {
"classes": [{
"class": "Article",
"properties": [
{"name": "title", "dataType": ["string"]},
{"name": "content", "dataType": ["text"]}
]
}]
}
client.schema.create(schema)
- Adding data:
article = {
"title": "Weaviate: The Vector Database",
"content": "Weaviate is a powerful vector database..."
}
client.data_object.create(
data_object=article,
class_name="Article"
)
- Performing a semantic search:
query = "What is a vector database?"
result = (
client.query
.get("Article", ["title", "content"])
.with_near_text({"concepts": [query]})
.with_limit(5)
.do()
)
print(result)
Getting Started
- Install Weaviate:
docker-compose up -d
- Install the Python client:
pip install weaviate-client
- Connect to Weaviate:
import weaviate
client = weaviate.Client("http://localhost:8080")
- Create a schema, add data, and perform queries as shown in the code examples above.
Competitor Comparisons
Qdrant - High-performance, massive-scale Vector Database and Vector Search Engine for the next generation of AI. Also available in the cloud https://cloud.qdrant.io/
Pros of Qdrant
- Written in Rust, offering high performance and memory safety
- Supports filtering during search, allowing for more precise queries
- Provides a simple and intuitive API for vector search operations
Cons of Qdrant
- Less mature ecosystem compared to Weaviate
- Fewer built-in integrations with other tools and services
- Limited support for schema management and data validation
Code Comparison
Qdrant (Python client):
from qdrant_client import QdrantClient
client = QdrantClient("localhost", port=6333)
client.create_collection("my_collection", vector_size=768)
client.upsert("my_collection", [(1, [0.1, 0.2, 0.3], {"name": "John"})])
Weaviate (Python client):
import weaviate
client = weaviate.Client("http://localhost:8080")
client.schema.create_class({
"class": "MyClass",
"vectorizer": "text2vec-transformers"
})
client.data_object.create({"name": "John"}, "MyClass")
Both Qdrant and Weaviate are vector databases, but they have different strengths. Qdrant excels in performance and filtering capabilities, while Weaviate offers a more comprehensive ecosystem and better schema management. The choice between them depends on specific project requirements and use cases.
Milvus is a high-performance, cloud-native vector database built for scalable vector ANN search
Pros of Milvus
- Better performance for large-scale vector similarity search
- More flexible deployment options (standalone, cluster, cloud-native)
- Supports multiple index types for different use cases
Cons of Milvus
- Steeper learning curve and more complex setup
- Limited support for non-vector data types
- Less integrated AI/ML capabilities out of the box
Code Comparison
Weaviate (Python client):
import weaviate
client = weaviate.Client("http://localhost:8080")
client.schema.create_class({
"class": "Article",
"vectorizer": "text2vec-transformers"
})
Milvus (Python client):
from pymilvus import connections, Collection, FieldSchema, CollectionSchema, DataType
connections.connect("default", host="localhost", port="19530")
fields = [
FieldSchema("id", DataType.INT64, is_primary=True),
FieldSchema("embedding", DataType.FLOAT_VECTOR, dim=128)
]
schema = CollectionSchema(fields, "Article")
collection = Collection("Article", schema)
Both repositories offer vector database solutions, but Milvus excels in performance and scalability for large datasets, while Weaviate provides a more integrated approach with built-in AI capabilities. Milvus offers more flexibility in deployment and indexing options, but may require more setup and configuration. Weaviate, on the other hand, offers a simpler setup process and better support for non-vector data types, making it more suitable for smaller-scale applications or those requiring a mix of vector and traditional data storage.
the AI-native open-source embedding database
Pros of Chroma
- Simpler setup and usage, ideal for quick prototyping and small-scale projects
- Native Python implementation, making it more accessible for Python developers
- Lightweight and easy to integrate into existing Python workflows
Cons of Chroma
- Less scalable for large-scale production environments compared to Weaviate
- Fewer advanced features and customization options
- Limited support for complex query types and data structures
Code Comparison
Chroma:
import chromadb
client = chromadb.Client()
collection = client.create_collection("my_collection")
collection.add(documents=["document1", "document2"], metadatas=[{"source": "web"}, {"source": "book"}], ids=["1", "2"])
results = collection.query(query_texts=["search query"], n_results=2)
Weaviate:
import weaviate
client = weaviate.Client("http://localhost:8080")
client.schema.create_class({
"class": "Document",
"properties": [{"name": "content", "dataType": ["text"]}]
})
client.data_object.create({"content": "document1"}, "Document")
result = client.query.get("Document", ["content"]).with_near_text({"concepts": ["search query"]}).do()
Free and Open Source, Distributed, RESTful Search Engine
Pros of Elasticsearch
- Mature ecosystem with extensive documentation and community support
- Powerful full-text search capabilities and advanced querying options
- Scalable and distributed architecture for handling large datasets
Cons of Elasticsearch
- Higher resource consumption and complexity in setup and maintenance
- Steeper learning curve for advanced features and optimizations
- Limited vector search capabilities compared to Weaviate's native support
Code Comparison
Elasticsearch query:
{
"query": {
"match": {
"title": "search example"
}
}
}
Weaviate query:
{
Get {
Article(
nearText: {
concepts: ["search example"]
}
) {
title
}
}
}
Both Elasticsearch and Weaviate offer powerful search capabilities, but they differ in their approach and specialization. Elasticsearch excels in traditional full-text search and analytics, while Weaviate focuses on vector search and AI-driven data operations. The choice between them depends on specific use cases and requirements, such as the need for vector search, scalability, and integration with AI models.
AI + Data, online. https://vespa.ai
Pros of Vespa
- More comprehensive feature set for large-scale applications
- Better support for real-time updates and complex queries
- Stronger focus on scalability and performance optimization
Cons of Vespa
- Steeper learning curve and more complex setup
- Requires more resources to run effectively
- Less user-friendly for smaller projects or beginners
Code Comparison
Weaviate (GraphQL query):
{
Get {
Article(
nearText: {
concepts: ["news"],
certainty: 0.7
}
) {
title
url
}
}
}
Vespa (YQL query):
select title, url from articles where {
{rank: nearestNeighbor(embedding, query_embedding)}
and has_embedding = true
}
limit 10;
Both repositories offer vector search capabilities, but Vespa provides a more SQL-like query language (YQL) compared to Weaviate's GraphQL approach. Vespa's query syntax may be more familiar to those with SQL experience, while Weaviate's GraphQL interface might be more intuitive for developers already working with GraphQL APIs.
Convert
designs to code with AI
Introducing Visual Copilot: A new AI model to turn Figma designs to high quality code using your components.
Try Visual CopilotREADME
Weaviate 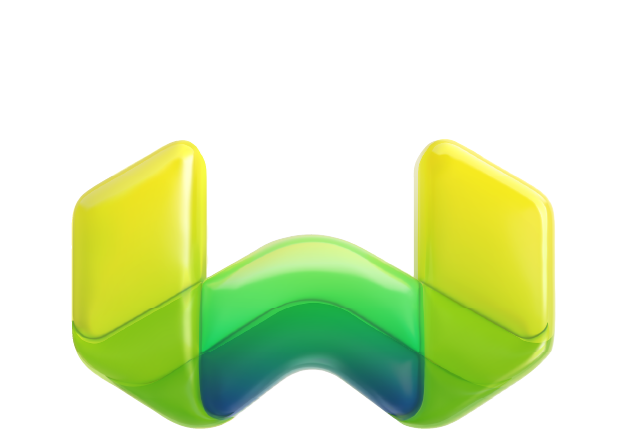
Overview
Weaviate is a cloud-native, open source vector database that is robust, fast, and scalable.
To get started quickly, have a look at one of these pages:
- Quickstart tutorial To see Weaviate in action
- Contributor guide To contribute to this project
For more details, read through the summary on this page or see the system documentation.
Why Weaviate?
Weaviate uses state-of-the-art machine learning (ML) models to turn your data - text, images, and more - into a searchable vector database.
Here are some highlights.
Speed
Weaviate is fast. The core engine can run a 10-NN nearest neighbor search on millions of objects in milliseconds. See benchmarks.
Flexibility
Weaviate can vectorize your data at import time. Or, if you have already vectorized your data, you can upload your own vectors instead.
Modules give you the flexibility to tune Weaviate for your needs. More than two dozen modules connect you to popular services and model hubs such as OpenAI, Cohere, VoyageAI and HuggingFace. Use custom modules to work with your own models or third party services.
Production-readiness
Weaviate is built with scaling, replication, and security in mind so you can go smoothly from rapid prototyping to production at scale.
Beyond search
Weaviate doesn't just power lightning-fast vector searches. Other superpowers include recommendation, summarization, and integration with neural search frameworks.
Who uses Weaviate?
-
Software Engineers
- Weaviate is an ML-first database engine
- Out-of-the-box modules for AI-powered searches, automatic classification, and LLM integration
- Full CRUD support
- Cloud-native, distributed system that runs well on Kubernetes
- Scales with your workloads
-
Data Engineers
- Weaviate is a fast, flexible vector database
- Use your own ML model or third party models
- Run locally or with an inference service
-
Data Scientists
- Seamless handover of Machine Learning models to engineers and MLOps
- Deploy and maintain your ML models in production reliably and efficiently
- Easily package custom trained models
What can you build with Weaviate?
A Weaviate vector database can search text, images, or a combination of both. Fast vector search provides a foundation for chatbots, recommendation systems, summarizers, and classification systems.
Here are some examples that show how Weaviate integrates with other AI and ML tools:
Use Weaviate with third party embeddings
Use Weaviate as a document store
Use Weaviate as a memory backend
Demos
These demos are working applications that highlight some of Weaviate's capabilities. Their source code is available on GitHub.
How can you connect to Weaviate?
Weaviate exposes a GraphQL API and a REST API. Starting in v1.23, a new gRPC API provides even faster access to your data.
Weaviate provides client libraries for several popular languages:
There are also community supported libraries for additional languages.
Where can You learn more?
Free, self-paced courses in Weaviate Academy teach you how to use Weaviate. The Tutorials repo has code for example projects. The Recipes repo has even more project code to get you started.
The Weaviate blog and podcast regularly post stories on Weaviate and AI.
Here are some popular posts:
Blogs
- What to expect from Weaviate in 2023
- Why is vector search so fast?
- Cohere Multilingual ML Models with Weaviate
- Vamana vs. HNSW - Exploring ANN algorithms Part 1
- HNSW+PQ - Exploring ANN algorithms Part 2.1
- The Tile Encoder - Exploring ANN algorithms Part 2.2
- How GPT4.0 and other Large Language Models Work
- Monitoring Weaviate in Production
- The ChatGPT Retrieval Plugin - Weaviate as a Long-term Memory Store for Generative AI
- Combining LangChain and Weaviate
- How to build an Image Search Application with Weaviate
- Cohere Multilingual ML Models with Weaviate
- Building Multimodal AI in TypeScript
- Giving Auto-GPT Long-Term Memory with Weaviate
Podcasts
Other reading
- Weaviate is an open-source search engine powered by ML, vectors, graphs, and GraphQL (ZDNet)
- Weaviate, an ANN Database with CRUD support (DB-Engines.com)
- A sub-50ms neural search with DistilBERT and Weaviate (Towards Datascience)
- Getting Started with Weaviate Python Library (Towards Datascience)
Join our community!
At Weaviate, we love to connect with our community. We love helping amazing people build cool things. And, we love to talk with you about you passion for vector databases and AI.
Please reach out, and join our community:
To keep up to date with new releases, meetup news, and more, subscribe to our newsletter
Top Related Projects
Qdrant - High-performance, massive-scale Vector Database and Vector Search Engine for the next generation of AI. Also available in the cloud https://cloud.qdrant.io/
Milvus is a high-performance, cloud-native vector database built for scalable vector ANN search
the AI-native open-source embedding database
Free and Open Source, Distributed, RESTful Search Engine
AI + Data, online. https://vespa.ai
Convert
designs to code with AI
Introducing Visual Copilot: A new AI model to turn Figma designs to high quality code using your components.
Try Visual Copilot