trieve
All-in-one infrastructure for search, recommendations, RAG, and analytics offered via API
Top Related Projects
⚡️ A fully-featured and blazing-fast JavaScript API client to interact with Algolia.
A lightning-fast search API that fits effortlessly into your apps, websites, and workflow
Open Source alternative to Algolia + Pinecone and an Easier-to-Use alternative to ElasticSearch ⚡ 🔍 ✨ Fast, typo tolerant, in-memory fuzzy Search Engine for building delightful search experiences
Official Elasticsearch client library for Node.js
🦔 Fast, lightweight & schema-less search backend. An alternative to Elasticsearch that runs on a few MBs of RAM.
Quick Overview
Trieve is an open-source vector database and semantic search engine. It provides a powerful platform for building AI-powered search applications, with features like semantic search, document chunking, and integration with large language models.
Pros
- Offers both vector database and semantic search capabilities in one solution
- Supports integration with popular large language models for enhanced search functionality
- Provides flexible document chunking options for optimized search results
- Open-source and self-hostable, allowing for customization and data privacy
Cons
- Relatively new project, which may lead to potential stability issues or lack of extensive community support
- Documentation could be more comprehensive, especially for advanced use cases
- Limited language support compared to some more established vector databases
- May require significant computational resources for large-scale deployments
Code Examples
# Initialize Trieve client
from trieve import TrieveClient
client = TrieveClient(api_key="your_api_key", server_url="https://your-trieve-instance.com")
# Add a document to the database
document = {
"content": "This is a sample document for semantic search.",
"metadata": {"author": "John Doe", "category": "Sample"}
}
client.add_document(document)
# Perform a semantic search
query = "Find documents about semantic search"
results = client.search(query, top_k=5)
for result in results:
print(f"Score: {result.score}, Content: {result.content[:100]}...")
# Use document chunking
long_document = {
"content": "This is a very long document that needs to be chunked...",
"metadata": {"title": "Long Document Example"}
}
client.add_document(long_document, chunk_size=500, chunk_overlap=50)
Getting Started
To get started with Trieve:
-
Install the Trieve client:
pip install trieve-client
-
Set up a Trieve instance (self-hosted or cloud-based)
-
Initialize the client and start using Trieve:
from trieve import TrieveClient client = TrieveClient(api_key="your_api_key", server_url="https://your-trieve-instance.com") # Add documents, perform searches, and utilize other features
For more detailed instructions and advanced usage, refer to the official Trieve documentation.
Competitor Comparisons
⚡️ A fully-featured and blazing-fast JavaScript API client to interact with Algolia.
Pros of algoliasearch-client-javascript
- More mature and widely adopted, with extensive documentation and community support
- Offers a broader range of features for search and indexing
- Provides seamless integration with Algolia's hosted search service
Cons of algoliasearch-client-javascript
- Requires a paid subscription to Algolia's service for production use
- Less flexibility for customization compared to self-hosted solutions
- May have higher latency due to reliance on external API calls
Code Comparison
algoliasearch-client-javascript:
const client = algoliasearch('YOUR_APP_ID', 'YOUR_API_KEY');
const index = client.initIndex('your_index_name');
index.search('query').then(({ hits }) => {
console.log(hits);
});
trieve:
let client = Client::new("YOUR_API_KEY");
let search_result = client.search("your_index_name", "query").await?;
println!("{:?}", search_result);
The code comparison shows that algoliasearch-client-javascript uses a JavaScript-based API, while trieve employs a Rust-based approach. algoliasearch-client-javascript provides a more familiar syntax for web developers, whereas trieve offers the benefits of Rust's performance and safety features. Both libraries aim to simplify the process of integrating search functionality into applications, but they cater to different language ecosystems and deployment models.
A lightning-fast search API that fits effortlessly into your apps, websites, and workflow
Pros of Meilisearch
- More mature and widely adopted search engine with a larger community
- Offers a wider range of features, including typo tolerance and faceted search
- Provides official SDKs for multiple programming languages
Cons of Meilisearch
- Requires more system resources and may be overkill for smaller projects
- Less focused on AI-powered search and natural language processing
- Steeper learning curve for advanced configurations
Code Comparison
Meilisearch index creation:
client = MeiliSearch::Client.new('http://127.0.0.1:7700', 'masterKey')
index = client.create_index('movies')
Trieve index creation:
from trieve import Trieve
trieve = Trieve(api_key="your_api_key")
index = trieve.create_index("movies")
Both Meilisearch and Trieve offer simple APIs for index creation, but Trieve's approach appears more streamlined. Meilisearch requires separate client initialization, while Trieve combines this step with index creation.
Meilisearch is a more established search engine with a broader feature set, making it suitable for larger projects with complex search requirements. Trieve, on the other hand, focuses on AI-powered search and may be more appropriate for projects requiring advanced natural language processing capabilities. The choice between the two depends on the specific needs of your project and the desired balance between features and simplicity.
Open Source alternative to Algolia + Pinecone and an Easier-to-Use alternative to ElasticSearch ⚡ 🔍 ✨ Fast, typo tolerant, in-memory fuzzy Search Engine for building delightful search experiences
Pros of Typesense
- More mature and established project with a larger community and ecosystem
- Offers a wider range of features and integrations out-of-the-box
- Provides official client libraries for multiple programming languages
Cons of Typesense
- Requires more setup and configuration compared to Trieve
- May have a steeper learning curve for beginners
- Less focused on AI-specific search capabilities
Code Comparison
Typesense query example:
client.collections['books'].search({
'q': 'harry potter',
'query_by': 'title,author',
'sort_by': 'ratings_count:desc'
})
Trieve query example:
client.search(
collection_name="books",
query="harry potter",
fields=["title", "author"],
sort=[("ratings_count", "desc")]
)
Both projects aim to provide efficient search capabilities, but Typesense offers a more comprehensive solution for general-purpose search, while Trieve focuses on AI-powered search and retrieval. Typesense may be better suited for larger projects with diverse search requirements, whereas Trieve could be more appropriate for applications specifically targeting AI-enhanced search functionality.
Official Elasticsearch client library for Node.js
Pros of elasticsearch-js
- Mature and widely adopted library with extensive documentation
- Supports a wide range of Elasticsearch features and operations
- Backed by Elastic, ensuring long-term support and updates
Cons of elasticsearch-js
- Larger codebase and potentially steeper learning curve
- Focused solely on Elasticsearch, limiting its use for other databases
- May require more configuration and setup for basic use cases
Code Comparison
elasticsearch-js:
const { Client } = require('@elastic/elasticsearch')
const client = new Client({ node: 'http://localhost:9200' })
await client.index({
index: 'my-index',
document: { title: 'Test', content: 'Hello world!' }
})
Trieve:
use trieve::Client;
let client = Client::new("http://localhost:8000");
client.index("my-index", json!({
"title": "Test",
"content": "Hello world!"
})).await?;
Summary
Elasticsearch-js is a robust and feature-rich library for interacting with Elasticsearch, offering comprehensive support for its ecosystem. It's ideal for projects deeply integrated with Elasticsearch. Trieve, on the other hand, appears to be a more lightweight and potentially easier-to-use alternative, possibly supporting multiple database types. The choice between them would depend on specific project requirements, existing infrastructure, and desired flexibility.
🦔 Fast, lightweight & schema-less search backend. An alternative to Elasticsearch that runs on a few MBs of RAM.
Pros of Sonic
- Written in Rust, offering high performance and memory safety
- Lightweight and designed for speed, with minimal resource usage
- Supports multiple search methods including word, phrase, and fuzzy search
Cons of Sonic
- Limited to text search and indexing, lacking advanced features like vector search
- Requires more manual configuration and integration compared to Trieve
- Less active development and smaller community support
Code Comparison
Sonic (search query):
let search_results = channel.search("default", "collection", "query", Some(10), None);
Trieve (search query):
results = client.search_chunks(
query="your search query",
dataset_id="your_dataset_id",
filters={"key": "value"},
page=1,
limit=10
)
Summary
Sonic is a fast, lightweight search backend written in Rust, focusing on text search and indexing. It offers excellent performance but has a narrower feature set. Trieve, on the other hand, provides a more comprehensive solution with advanced features like vector search and semantic analysis, but may have higher resource requirements. The choice between the two depends on specific project needs, with Sonic being ideal for simple, high-performance text search, and Trieve offering more advanced capabilities for complex search and analysis tasks.
Convert
designs to code with AI
Introducing Visual Copilot: A new AI model to turn Figma designs to high quality code using your components.
Try Visual CopilotREADME
Sign Up (1k chunks free) | PDF2MD | Hacker News Search Engine | Documentation | Meet a Maintainer | Discord | Matrix
All-in-one solution for search, recommendations, and RAG
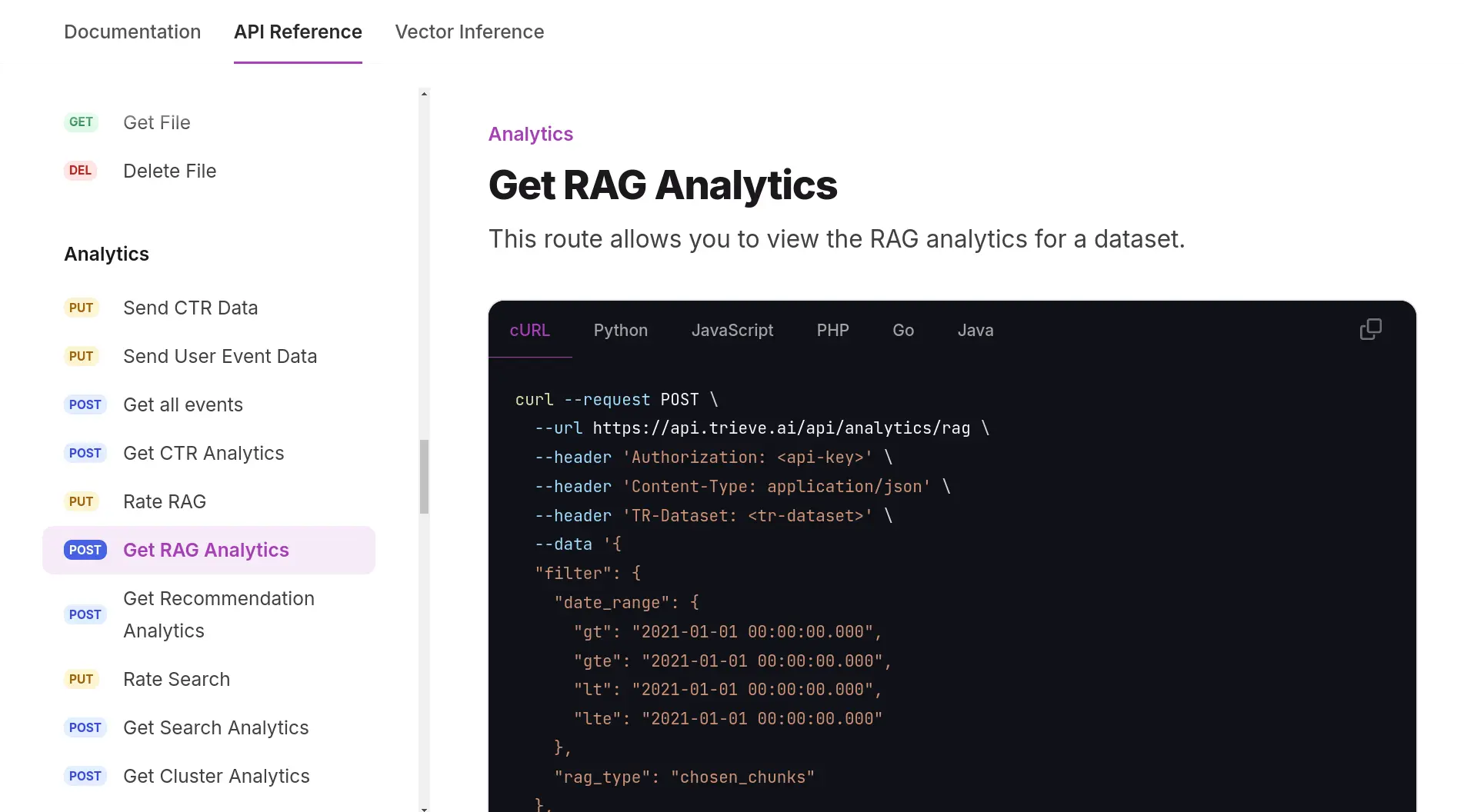
Quick Links
Features
- ð Self-Hosting in your VPC or on-prem: We have full self-hosting guides for AWS, GCP, Kubernetes generally, and docker compose available on our documentation page here.
- ð§ Semantic Dense Vector Search: Integrates with OpenAI or Jina embedding models and Qdrant to provide semantic vector search.
- ð Typo Tolerant Full-Text/Neural Search: Every uploaded chunk is vector'ized with naver/efficient-splade-VI-BT-large-query for typo tolerant, quality neural sparse-vector search.
- ðï¸ Sub-Sentence Highlighting: Highlight the matching words or sentences within a chunk and bold them on search to enhance UX for your users. Shout out to the simsearch crate!
- ð Recommendations: Find similar chunks (or files if using grouping) with the recommendation API. Very helpful if you have a platform where users' favorite, bookmark, or upvote content.
- ð¤ Convenient RAG API Routes: We integrate with OpenRouter to provide you with access to any LLM you would like for RAG. Try our routes for fully-managed RAG with topic-based memory management or select your own context RAG.
- ð¼ Bring Your Own Models: If you'd like, you can bring your own text-embedding, SPLADE, cross-encoder re-ranking, and/or large-language model (LLM) and plug it into our infrastructure.
- ð Hybrid Search with cross-encoder re-ranking: For the best results, use hybrid search with BAAI/bge-reranker-large re-rank optimization.
- ð Recency Biasing: Easily bias search results for what was most recent to prevent staleness
- ð ï¸ Tunable Merchandizing: Adjust relevance using signals like clicks, add-to-carts, or citations
- ð³ï¸ Filtering: Date-range, substring match, tag, numeric, and other filter types are supported.
- ð¥ Grouping: Mark multiple chunks as being part of the same file and search on the file-level such that the same top-level result never appears twice
Are we missing a feature that your use case would need? - call us at 628-222-4090, make a Github issue, or join the Matrix community and tell us! We are a small company who is still very hands-on and eager to build what you need; professional services are available.
Local development with Linux
Installing via Smithery
To install Trieve for Claude Desktop automatically via Smithery:
npx -y @smithery/cli install trieve-mcp-server --client claude
Debian/Ubuntu Packages needed packages
sudo apt install curl \
gcc \
g++ \
make \
pkg-config \
python3 \
python3-pip \
libpq-dev \
libssl-dev \
openssl
Arch Packages needed
sudo pacman -S base-devel postgresql-libs
Install NodeJS and Yarn
You can install NVM using its install script.
curl -o- https://raw.githubusercontent.com/nvm-sh/nvm/v0.39.5/install.sh | bash
You should restart the terminal to update bash profile with NVM. Then, you can install NodeJS LTS release and Yarn.
nvm install --lts
npm install -g yarn
Make server tmp dir
mkdir server/tmp
Install rust
curl --proto '=https' --tlsv1.2 -sSf https://sh.rustup.rs | sh
Install cargo-watch
cargo install cargo-watch
Setup env's
You might need to create the analytics
directory in ./frontends
cp .env.analytics ./frontends/analytics/.env
cp .env.chat ./frontends/chat/.env
cp .env.search ./frontends/search/.env
cp .env.example ./server/.env
cp .env.dashboard ./frontends/dashboard/.env
Add your LLM_API_KEY
to ./server/.env
Here is a guide for acquiring that.
Steps once you have the key
- Open the
./server/.env
file - Replace the value for
LLM_API_KEY
to be your own OpenAI API key. - Replace the value for
OPENAI_API_KEY
to be your own OpenAI API key.
Export the following keys in your terminal for local dev
The PAGEFIND_CDN_BASE_URL and S3_SECRET_KEY_CSVJSONL could be set to a random list of strings.
export OPENAI_API_KEY="your_OpenAI_api_key" \
LLM_API_KEY="your_OpenAI_api_key" \
PAGEFIND_CDN_BASE_URL="lZP8X4h0Q5Sj2ZmV,aAmu1W92T6DbFUkJ,DZ5pMvz8P1kKNH0r,QAqwvKh8rI5sPmuW,YMwgsBz7jLfN0oX8" \
S3_SECRET_KEY_CSVJSONL="Gq6wzS3mjC5kL7i4KwexnL3gP8Z1a5Xv,V2c4ZnL0uHqBzFvR2NcN8Pb1g6CjmX9J,TfA1h8LgI5zYkH9A9p7NvWlL0sZzF9p8N,pKr81pLq5n6MkNzT1X09R7Qb0Vn5cFr0d,DzYwz82FQiW6T3u9A4z9h7HLOlJb7L2V1" \
GROQ_API_KEY="GROQ_API_KEY_if_applicable"
Start docker container services needed for local dev
cat .env.chat .env.search .env.server .env.docker-compose > .env
./convenience.sh -l
Start services for local dev
cd clients/ts-sdk
yarn build
cd frontends
yarn
yarn dev
We recommend managing this through tmuxp, see the guide here or terminal tabs.
cd server
cargo watch -x run
cd server
cargo run --bin ingestion-worker
cd server
cargo run --bin file-worker
cd server
cargo run --bin delete-worker
cd search
yarn
yarn dev
Verify Working Setup
After the cargo build has finished (after the tmuxp load trieve
):
- check that you can see redoc with the OpenAPI reference at localhost:8090/redoc
- make an account create a dataset with test data at localhost:5173
- search that dataset with test data at localhost:5174
Additional Instructions for testing cross encoder reranking models
To test the Cross Encoder rerankers in local dev,
- click on the dataset, go to the Dataset Settings -> Dataset Options -> Additional Options and uncheck the
Fulltext Enabled
option. - in the Embedding Settings, select your reranker model and enter the respective key in the adjacent textbox, and hit save.
- in the search playground, set Type -> Semantic and select Rerank By -> Cross Encoder
- if AIMon Reranker is selected in the Embedding Settings, you can enter an optional Task Definition in the search playground to specify the domain of context documents to the AIMon reranker.
Debugging issues with local dev
Reach out to us on discord for assistance. We are available and more than happy to assist.
Debug diesel by getting the exact generated SQL
diesel::debug_query(&query).to_string();
Local Setup for Testing Stripe Features
Install Stripe CLI.
stripe login
stripe listen --forward-to localhost:8090/api/stripe/webhook
- set the
STRIPE_WEBHOOK_SECRET
in theserver/.env
to the resulting webhook signing secret stripe products create --name trieve --default-price-data.unit-amount 1200 --default-price-data.currency usd
stripe plans create --amount=1200 --currency=usd --interval=month --product={id from response of step 3}
Contributors
Top Related Projects
⚡️ A fully-featured and blazing-fast JavaScript API client to interact with Algolia.
A lightning-fast search API that fits effortlessly into your apps, websites, and workflow
Open Source alternative to Algolia + Pinecone and an Easier-to-Use alternative to ElasticSearch ⚡ 🔍 ✨ Fast, typo tolerant, in-memory fuzzy Search Engine for building delightful search experiences
Official Elasticsearch client library for Node.js
🦔 Fast, lightweight & schema-less search backend. An alternative to Elasticsearch that runs on a few MBs of RAM.
Convert
designs to code with AI
Introducing Visual Copilot: A new AI model to turn Figma designs to high quality code using your components.
Try Visual Copilot