Top Related Projects
Data Apps & Dashboards for Python. No JavaScript Required.
Streamlit — A faster way to build and share data apps.
Panel: The powerful data exploration & web app framework for Python
Interactive Data Visualization in the browser, from Python
Declarative visualization library for Python
1 Line of code data quality profiling & exploratory data analysis for Pandas and Spark DataFrames.
Quick Overview
Manifold is an open-source library developed by Uber that provides a unified interface for training and deploying machine learning models. It aims to simplify the process of building and deploying ML models by abstracting away the underlying complexities of different ML frameworks and platforms.
Pros
- Unified Interface: Manifold provides a consistent API for working with various ML frameworks, including TensorFlow, PyTorch, and ONNX, making it easier to switch between them.
- Deployment Flexibility: Manifold supports deployment to a variety of platforms, including cloud services, edge devices, and mobile devices, allowing for seamless model deployment.
- Scalability: Manifold is designed to handle large-scale ML workloads, making it suitable for enterprise-level applications.
- Extensibility: Manifold is designed to be extensible, allowing developers to add support for new ML frameworks and deployment platforms.
Cons
- Limited Documentation: The project's documentation, while improving, could be more comprehensive, making it challenging for new users to get started.
- Steep Learning Curve: Manifold's abstraction layer and the need to understand its conventions and patterns can present a learning curve for developers who are new to the library.
- Dependency on Uber: As Manifold is an Uber-developed project, there may be concerns about its long-term sustainability and community support outside of Uber.
- Performance Overhead: The abstraction layer provided by Manifold may introduce some performance overhead compared to directly using the underlying ML frameworks.
Code Examples
Example 1: Training a TensorFlow Model
import manifold
from manifold.frameworks.tensorflow import TensorflowModel
# Define the model
model = TensorflowModel(
model_fn=your_model_fn,
input_specs=your_input_specs,
output_specs=your_output_specs,
)
# Train the model
model.train(
train_data=your_train_data,
val_data=your_val_data,
epochs=10,
batch_size=32,
)
This example demonstrates how to use the TensorflowModel
class in Manifold to train a TensorFlow model.
Example 2: Deploying a PyTorch Model
import manifold
from manifold.frameworks.pytorch import PyTorchModel
# Define the model
model = PyTorchModel(
model_fn=your_model_fn,
input_specs=your_input_specs,
output_specs=your_output_specs,
)
# Deploy the model
model.deploy(
deployment_config=your_deployment_config,
target_platform=your_target_platform,
)
This example shows how to use the PyTorchModel
class in Manifold to deploy a PyTorch model to a target platform.
Example 3: Evaluating an ONNX Model
import manifold
from manifold.frameworks.onnx import ONNXModel
# Define the model
model = ONNXModel(
model_path=your_onnx_model_path,
input_specs=your_input_specs,
output_specs=your_output_specs,
)
# Evaluate the model
metrics = model.evaluate(
test_data=your_test_data,
batch_size=32,
)
This example demonstrates how to use the ONNXModel
class in Manifold to evaluate an ONNX model.
Getting Started
To get started with Manifold, follow these steps:
- Install the Manifold library using pip:
pip install manifold
- Import the necessary modules and define your model:
from manifold.frameworks.tensorflow import TensorflowModel
model = TensorflowModel(
model_fn=your_model_fn,
input_specs=your_input_specs,
output_specs=your_output_specs,
)
- Train your model using the
train()
method:
model.train(
train_data=your_train_data,
val_data=your_val_data,
epochs=
Competitor Comparisons
Data Apps & Dashboards for Python. No JavaScript Required.
Pros of Dash
- More comprehensive web application framework for building interactive dashboards
- Extensive documentation and community support
- Integrates seamlessly with other Plotly libraries
Cons of Dash
- Steeper learning curve for beginners
- Can be slower for large datasets compared to Manifold's performance optimizations
- Less focused on machine learning model analysis
Code Comparison
Dash example:
import dash
import dash_core_components as dcc
import dash_html_components as html
app = dash.Dash(__name__)
app.layout = html.Div([
dcc.Graph(id='example-graph')
])
Manifold example:
import manifold
data = manifold.Data(df)
fig = manifold.Figure(data)
fig.scatter('feature1', 'feature2', color='target')
Summary
Dash is a more versatile web application framework for creating interactive dashboards, while Manifold focuses specifically on machine learning model analysis and visualization. Dash offers greater flexibility but may require more setup, whereas Manifold provides a more streamlined experience for ML-specific tasks. The choice between the two depends on the specific requirements of your project and your familiarity with web development concepts.
Streamlit — A faster way to build and share data apps.
Pros of Streamlit
- Easier to learn and use for beginners
- Faster development of simple web applications
- More extensive documentation and community support
Cons of Streamlit
- Less flexibility for complex visualizations
- Limited customization options for UI components
- Performance can be slower for large datasets
Code Comparison
Streamlit:
import streamlit as st
import pandas as pd
df = pd.read_csv("data.csv")
st.line_chart(df)
Manifold:
import React from 'react';
import {LineChart} from '@uber/manifold';
const MyChart = ({data}) => (
<LineChart data={data} />
);
Both libraries aim to simplify data visualization, but Streamlit focuses on rapid prototyping and ease of use, while Manifold offers more advanced features for complex visualizations. Streamlit uses Python and integrates well with data science workflows, whereas Manifold is built on React and provides more customization options for experienced developers.
Panel: The powerful data exploration & web app framework for Python
Pros of Panel
- More versatile and general-purpose data visualization framework
- Supports a wider range of data sources and plotting libraries
- Easier integration with existing Python workflows and Jupyter notebooks
Cons of Panel
- Steeper learning curve for complex dashboards
- Less specialized for machine learning model analysis
- May require more custom coding for specific ML visualization tasks
Code Comparison
Panel example:
import panel as pn
import numpy as np
def plot(n):
return pn.Column(
pn.pane.Markdown(f"# Sine wave with {n} points"),
pn.pane.Bokeh(np.sin(np.linspace(0, 10, n)))
)
pn.interact(plot, n=(10, 100))
Manifold example:
import React from 'react';
import {Manifold} from '@mlvis/manifold';
const MyComponent = () => (
<Manifold
data={data}
features={features}
segments={segments}
/>
);
Note: The code examples demonstrate basic usage and may not reflect the full capabilities of each library. Panel uses Python, while Manifold uses JavaScript/React, making direct comparison challenging.
Interactive Data Visualization in the browser, from Python
Pros of Bokeh
- More mature and established project with a larger community and ecosystem
- Supports a wider range of interactive visualization types and customization options
- Better documentation and learning resources available
Cons of Bokeh
- Steeper learning curve for beginners compared to Manifold
- Can be slower to render complex visualizations with large datasets
- Requires more code to create basic visualizations
Code Comparison
Bokeh:
from bokeh.plotting import figure, show
p = figure(title="Simple Line Plot")
p.line([1, 2, 3, 4, 5], [6, 7, 2, 4, 5])
show(p)
Manifold:
import manifold
df = pd.DataFrame({'x': [1, 2, 3, 4, 5], 'y': [6, 7, 2, 4, 5]})
manifold.plot(df, x='x', y='y')
Both Bokeh and Manifold are powerful data visualization libraries, but they cater to different use cases and skill levels. Bokeh offers more flexibility and customization options, making it suitable for complex visualizations and advanced users. Manifold, on the other hand, provides a simpler interface for quick and easy visualizations, particularly for machine learning model analysis. The choice between the two depends on the specific requirements of your project and your familiarity with data visualization concepts.
Declarative visualization library for Python
Pros of Altair
- Declarative approach to visualization, making it easier to create complex charts with less code
- Extensive documentation and examples, aiding in learning and implementation
- Integration with Jupyter notebooks for interactive data exploration
Cons of Altair
- Limited customization options compared to lower-level libraries
- Performance can be slower for large datasets
- Steeper learning curve for users unfamiliar with the grammar of graphics concept
Code Comparison
Altair:
import altair as alt
import pandas as pd
data = pd.DataFrame({'x': [1, 2, 3], 'y': [4, 5, 6]})
chart = alt.Chart(data).mark_line().encode(x='x', y='y')
Manifold:
import { ScatterplotLayer } from '@deck.gl/layers';
import { Manifold } from '@uber/manifold';
const layer = new ScatterplotLayer({
data: [{x: 1, y: 4}, {x: 2, y: 5}, {x: 3, y: 6}],
getPosition: d => [d.x, d.y]
});
Altair focuses on a high-level, declarative approach to creating visualizations, while Manifold provides more low-level control and is designed for large-scale data visualization. Altair is Python-based and integrates well with data science workflows, whereas Manifold is JavaScript-based and is better suited for web-based applications and custom visualizations.
1 Line of code data quality profiling & exploratory data analysis for Pandas and Spark DataFrames.
Pros of ydata-profiling
- Focuses on comprehensive data profiling and generates detailed HTML reports
- Supports a wide range of data types and provides in-depth statistical analysis
- Easy to use with a simple API, requiring minimal code to generate reports
Cons of ydata-profiling
- Limited to data profiling and doesn't offer interactive visualizations
- May be slower for large datasets compared to Manifold's performance
- Less flexibility in customizing visualizations and analysis
Code Comparison
ydata-profiling:
from ydata_profiling import ProfileReport
profile = ProfileReport(df)
profile.to_file("output.html")
Manifold:
from manifold import Manifold
manifold = Manifold(df)
manifold.plot(features=['feature1', 'feature2'], label='target')
Summary
ydata-profiling excels in comprehensive data profiling and generating detailed reports, making it ideal for initial data exploration and understanding. It's user-friendly and requires minimal code to produce insightful results. However, it lacks the interactive visualizations and customization options that Manifold offers.
Manifold, on the other hand, provides a more interactive and flexible approach to data analysis, focusing on model debugging and performance visualization. It's better suited for machine learning practitioners who need to dive deep into model behavior and feature importance.
The choice between the two depends on the specific needs of the project: ydata-profiling for quick, comprehensive data profiling, or Manifold for interactive model analysis and debugging.
Convert
designs to code with AI
Introducing Visual Copilot: A new AI model to turn Figma designs to high quality code using your components.
Try Visual CopilotREADME
Manifold
This project is stable and being incubated for long-term support.
Manifold is a model-agnostic visual debugging tool for machine learning.
Understanding ML model performance and behavior is a non-trivial process, given the intrisic opacity of ML algorithms. Performance summary statistics such as AUC, RMSE, and others are not instructive enough for identifying what went wrong with a model or how to improve it.
As a visual analytics tool, Manifold allows ML practitioners to look beyond overall summary metrics to detect which subset of data a model is inaccurately predicting. Manifold also explains the potential cause of poor model performance by surfacing the feature distribution difference between better and worse-performing subsets of data.
Table of contents
- Prepare your data
- Interpret visualizations
- Using the demo app
- Using the component
- Contributing
- Versioning
- License
Prepare Your Data
There are 2 ways to input data into Manifold:
- csv upload if you use the Manifold demo app, or
- convert data programatically if you use the Manifold component in your own app.
In either case, data that's directly input into Manifold should follow this format:
const data = {
x: [...], // feature data
yPred: [[...], ...] // prediction data
yTrue: [...], // ground truth data
};
Each element in these arrays represents one data point in your evaluation dataset, and the order of data instances in x
, yPred
and yTrue
should all match.
The recommended instance count for each of these datasets is 10000 - 15000. If you have a larger dataset that you want to analyze, a random subset of your data generally suffices to reveal the important patterns in it.
x
: {Object[]}
A list of instances with features. Example (2 data instances):
[{feature_0: 21, feature_1: 'B'}, {feature_0: 36, feature_1: 'A'}];
yPred
: {Object[][]}
A list of lists, where each child list is a prediction array from one model for each data instance. Example (3 models, 2 data instances, 2 classes ['false', 'true']
):
[
[{false: 0.1, true: 0.9}, {false: 0.8, true: 0.2}],
[{false: 0.3, true: 0.7}, {false: 0.9, true: 0.1}],
[{false: 0.6, true: 0.4}, {false: 0.4, true: 0.6}],
];
yTrue
: {Number[] | String[]}
A list, ground truth for each data instance. Values must be numbers for regression models, must be strings that match object keys in yPred
for classification models. Example (2 data instances, 2 classes ['false', 'true']):
['true', 'false'];
Interpret visualizations
This guide explains how to interpret Manifold visualizations.
Manifold consists of:
- Performance Comparison View which compares prediction performance across models, across data subsets
- Feature Attribution View which visualizes feature distributions of data subsets with various performance levels
Performance Comparison View
This visualization is an overview of performance of your model(s) across different segments of your data. It helps you identify under-performing data subsets for further inspection.
Reading the chart
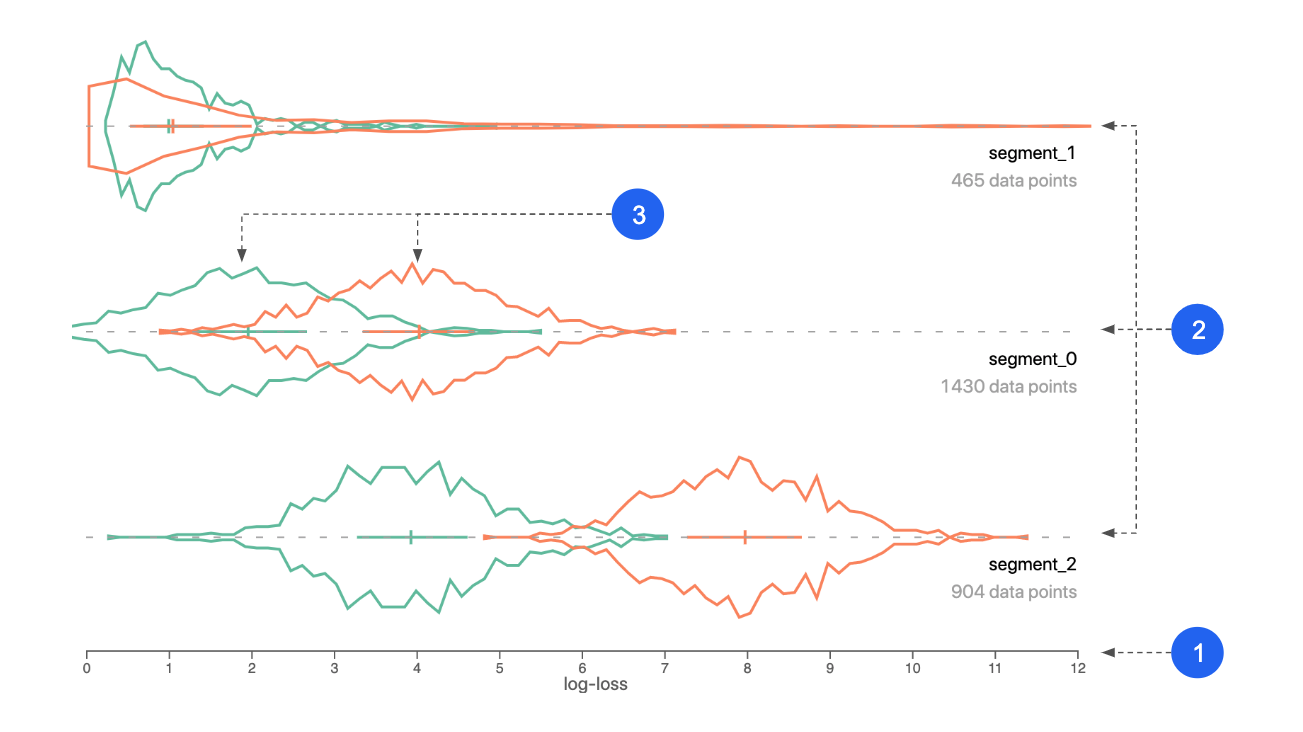
- X axis: performance metric. Could be log-loss, squared-error, or raw prediction.
- Segments: your dataset is automatically divided into segments based on performance similarity between instances, across models.
- Colors: represent different models.
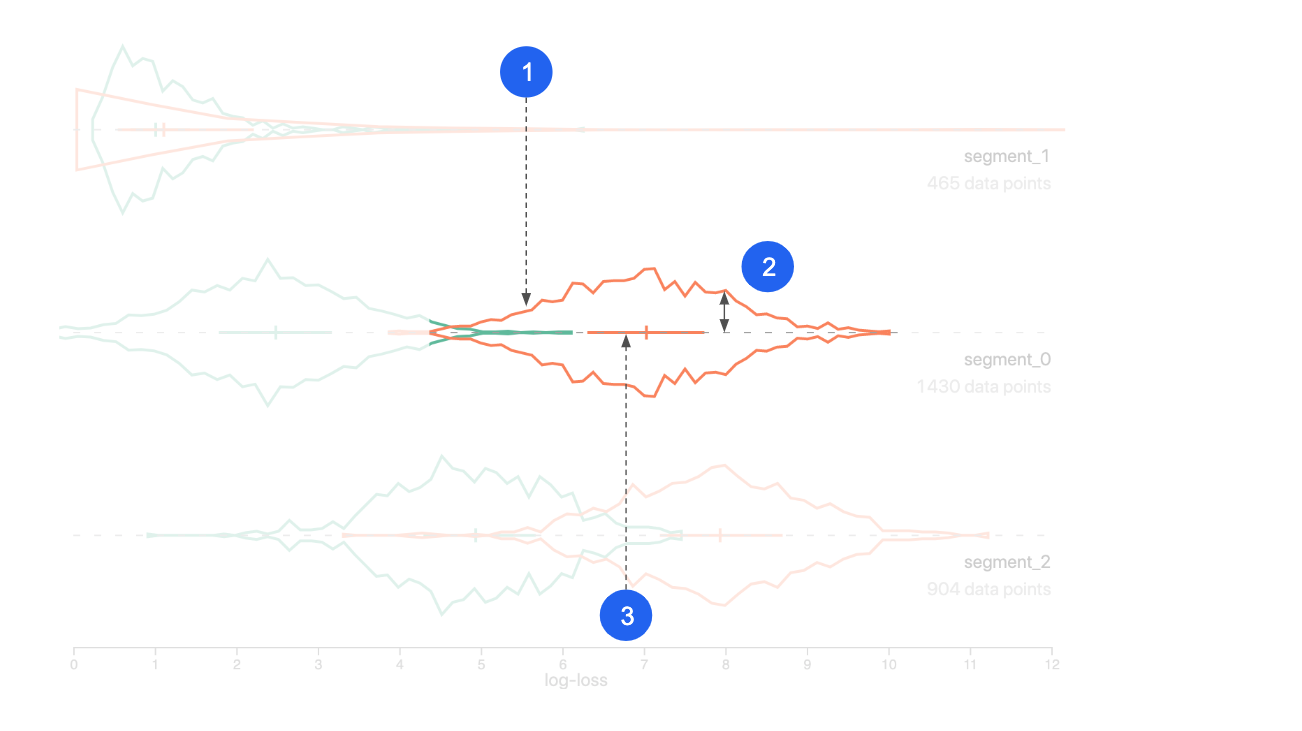
- Curve: performance distribution (of one model, for one segment).
- Y axis: data count/density.
- Cross: the left end, center line, and right end are the 25th, 50th and 75th percentile of the distribution.
Explanation
Manifold uses a clustering algorithm (k-Means) to break prediction data into N segments based on performance similarity.
The input of the k-Means is per-instance performance scores. By default, that is the log-loss value for classification models and the squared-error value for regression models. Models with a lower log-loss/squared-error perform better than models with a higher log-loss/squared-error.
If you're analyzing multiple models, all model performance metrics will be included in the input.
Usage
-
Look for segments of data where the error is higher (plotted to the right). These are areas you should analyze and try to improve.
-
If you're comparing models, look for segments where the log-loss is different for each model. If two models perform differently on the same set of data, consider using the better-performing model for that part of the data to boost performance.
-
After you notice any performance patterns/issues in the segments, slice the data to compare feature distribution for the data subset(s) of interest. You can create two segment groups to compare (colored pink and blue), and each group can have 1 or more segments.
Example
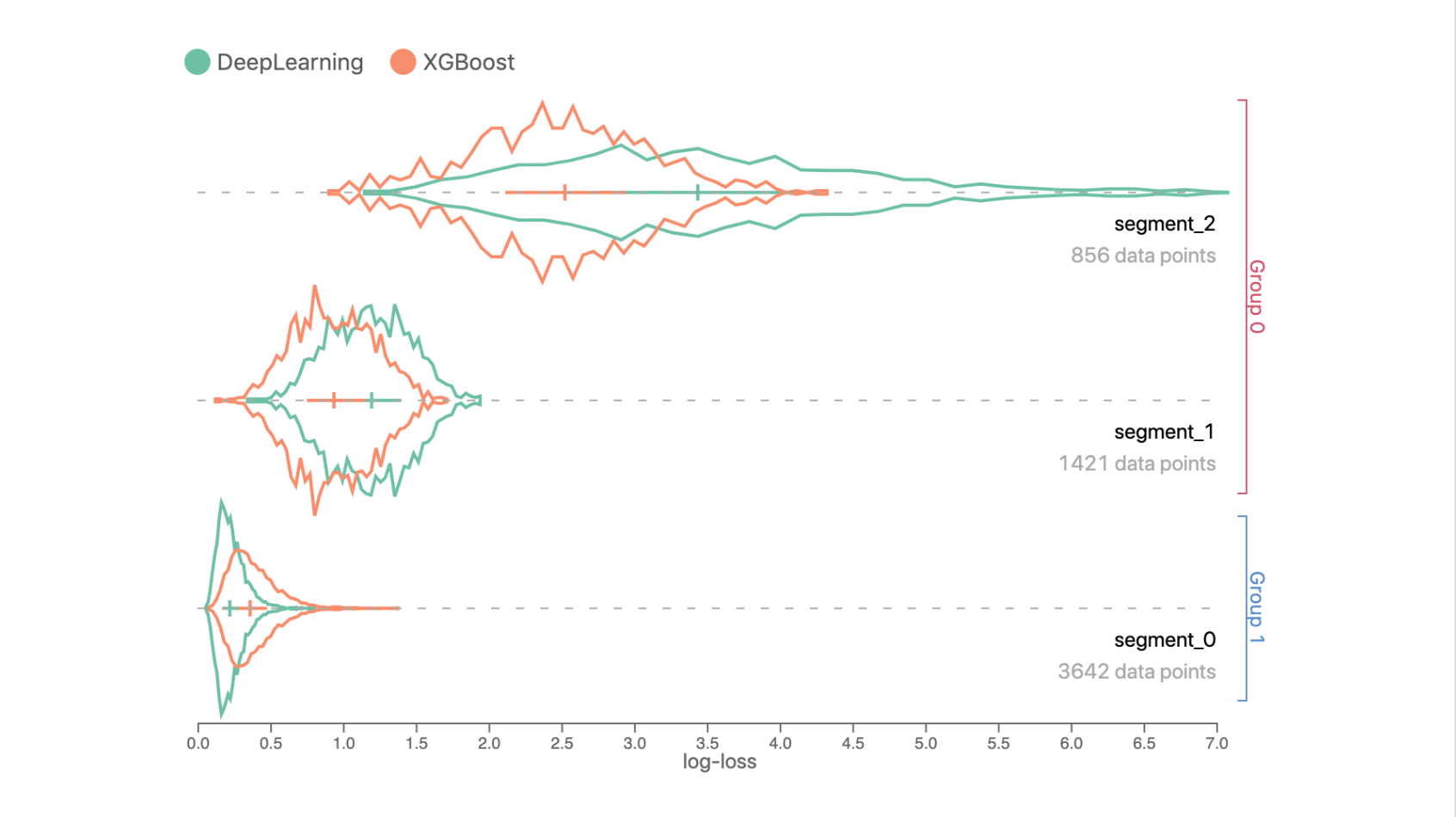
Data in Segment 0 has a lower log-loss prediction error compared to Segments 1 and 2, since curves in Segment 0 are closer to the left side.
In Segments 1 and 2, the XGBoost model performs better than the DeepLearning model, but DeepLearning outperforms XGBoost in Segment 0.
Feature Attribution View
This visualization shows feature values of your data, aggregated by user-defined segments. It helps you identify any input feature distribution that might correlate with inaccurate prediction output.
Reading the chart
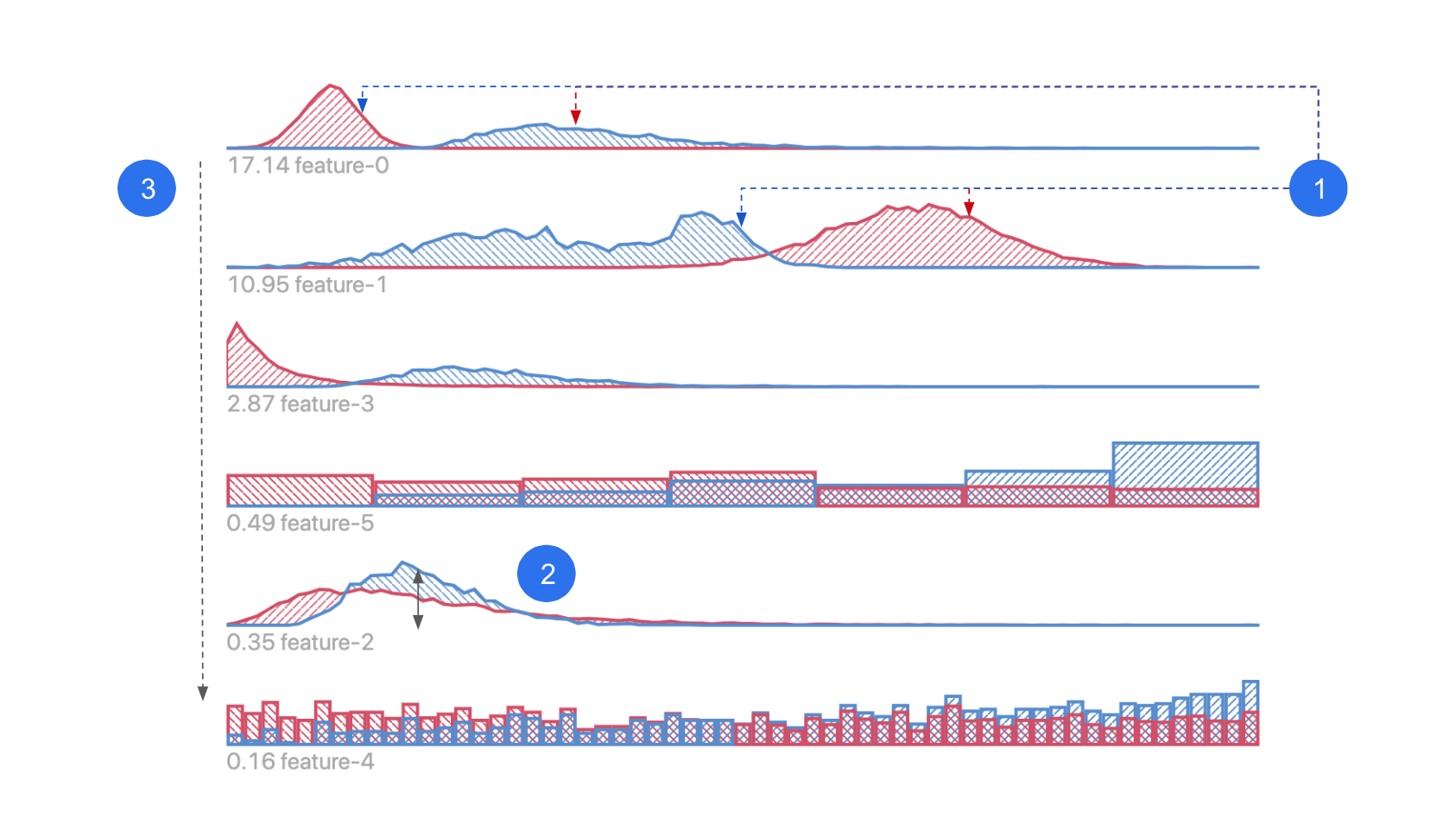
- Histogram / heatmap: distribution of data from each data slice, shown in the corresponding color.
- Segment groups: indicates data slices you choose to compare against each other.
- Ranking: features are ranked by distribution difference between slices.
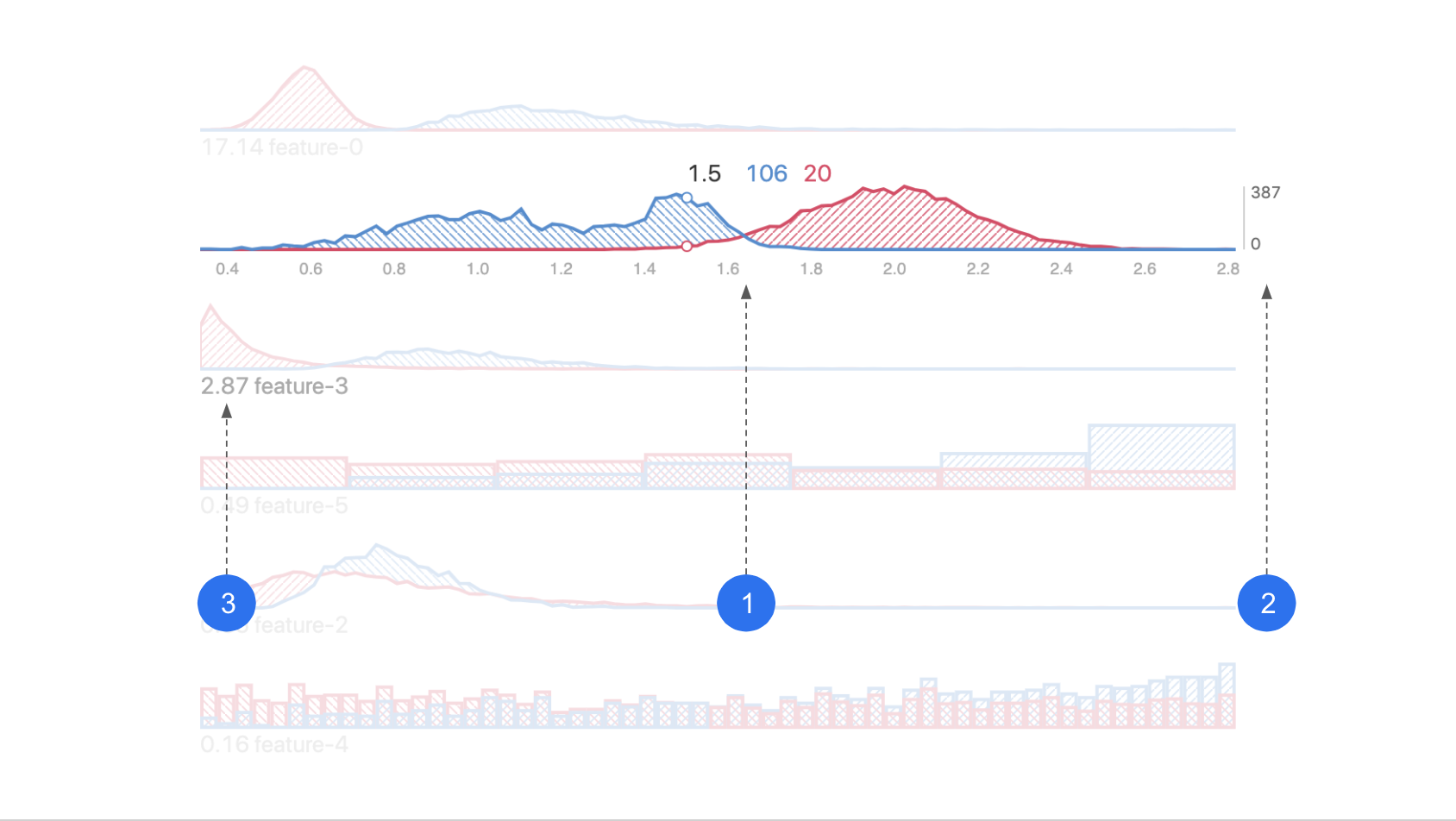
- X axis: feature value.
- Y axis: data count/density.
- Divergence score: measure of difference in distributions between slices.
Explanation
After you slice the data to create segment groups, feature distribution histograms/heatmaps from the two segment groups are shown in this view.
Depending on the feature type, features can be shown as heatmaps on a map for geo features, distribution curve for numerical features, or distribution bar chart for categorical features. (In bar charts, categories on the x-axis are sorted by instance count difference. Look for differences between the two distributions in each feature.)
Features are ranked by their KL-Divergence - a measure of difference between the two contrasting distributions. The higher the divergence is, the more likely this feature is correlated with the factor that differentiates the two Segment Groups.
Usage
- Look for the differences between the two distributions (pink and blue) in each feature. They represent the difference in data from the two segment groups you selected in the Performance Comparison View.
Example
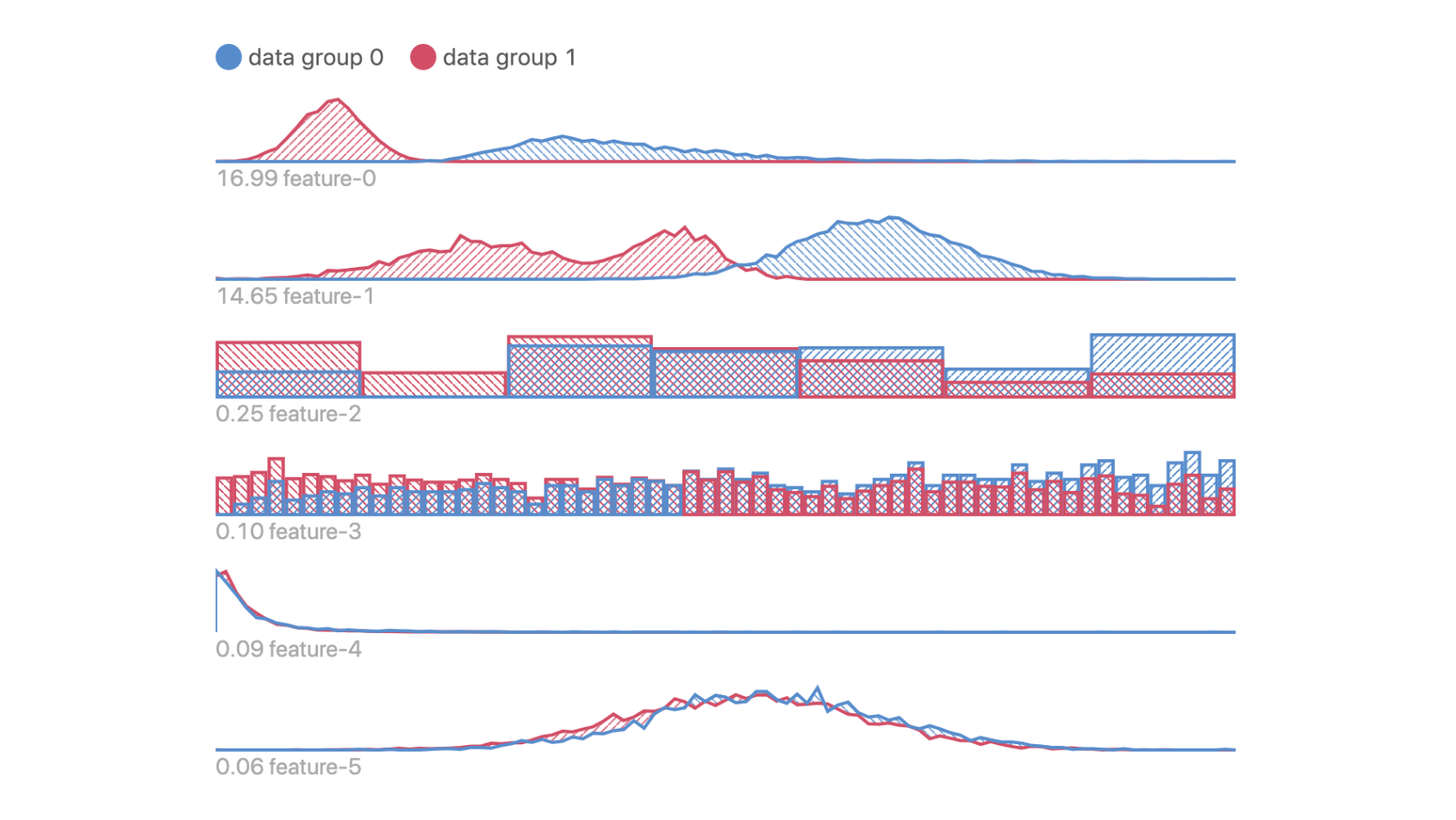
Data in Groups 0 and 1 have obvious differences in Features 0, 1, 2 and 3; but they are not so different in features 4 and 5.
Suppose Data Groups 0 and 1 correspond to data instances with low and high prediction error respectively, this means that data with higher errors tend to have lower feature values in Features 0 and 1, since peak of pink curve is to the left side of the blue curve.
Geo Feature View
If there are geospatial features in your dataset, they will be displayed on a map. Lat-lng coordinates and h3 hexagon ids are currently supoorted geo feature types.
Reading the chart
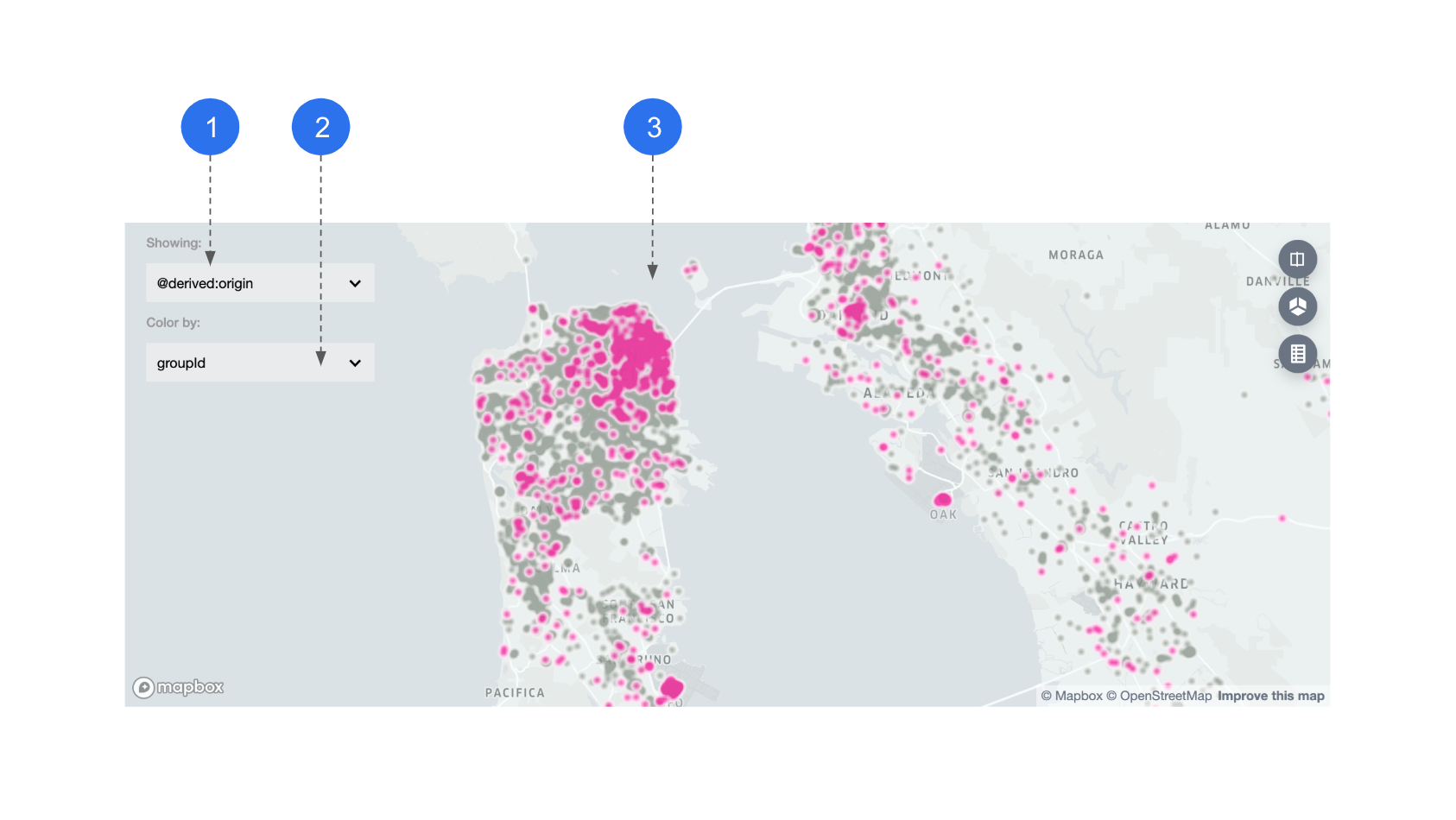
- Feature name: when multiple geo features exist, you can choose which one to display on the map.
- Color-by: if a lat-lng feature is chosen, datapoints are colored by group ids.
- Map: Manifold defaults to display the location and density of these datapoints using a heatmap.
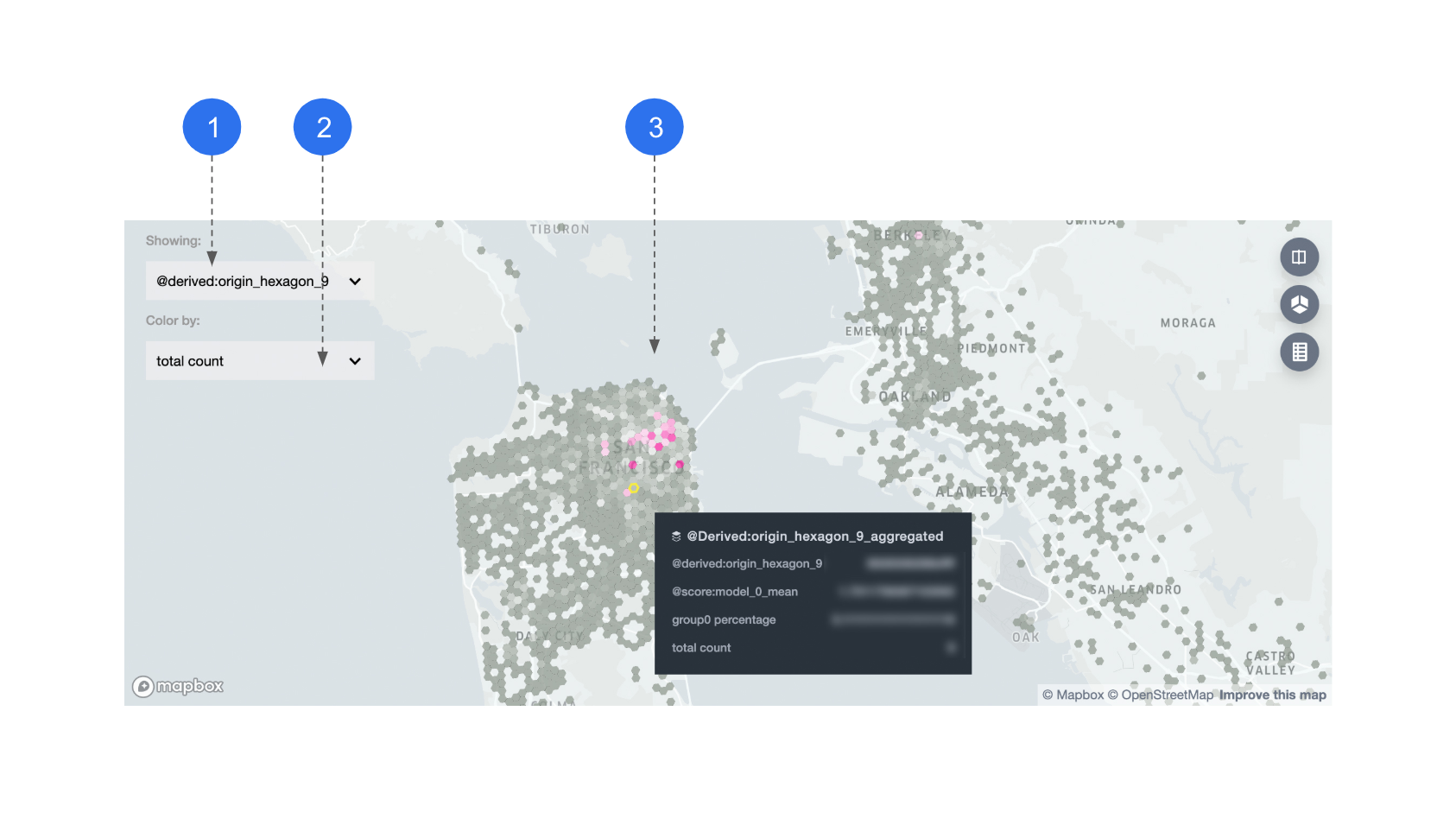
- Feature name: when choosing a hex-id feature to display, datapoints with the same hex-id are displayed in aggregate.
- Color-by: you can color the hexagons by: average model performance, percentage of segment group 0, or total count per hexagon.
- Map: all metrics that are used for coloring are also shown in tooltips, on the hexagon level.
Usage
- Look for the differences in geo location between the two segment groups (pink and grey). They represent the spation distribution difference between the two subsets you previously selected.
Example
In the first map above, Group 0 has a more obvious tendency to be concentrated in downtown San Francisco area.
Using the Demo App
To do a one-off evaluation using static outputs of your ML models, use the demo app. Otherwise, if you have a system that programmatically generates ML model outputs, you might consider using the Manifold component directly.
Running Demo App Locally
Run the following commands to set up your environment and run the demo:
# install all dependencies in the root directory
yarn
# demo app is in examples/manifold directory
cd examples/manifold
# install dependencies for the demo app
yarn
# run the app
yarn start
Now you should see the demo app running at localhost:8080
.
Upload CSV to Demo App
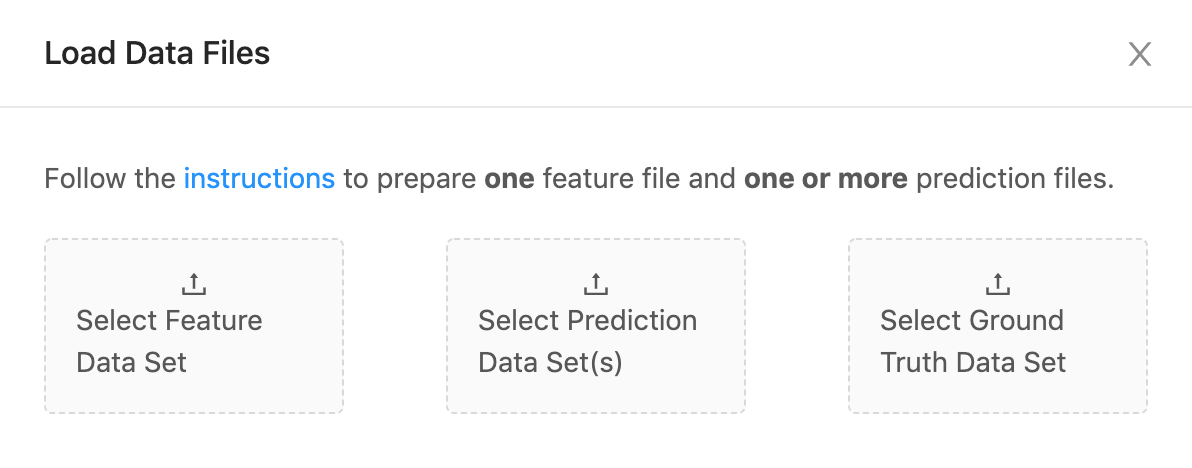
Once the app starts running, you will see the interface above asking you to upload "feature", "prediction" and "ground truth" datasets to Manifold.
They correspond to x
, yPred
, and yTrue
in the "prepare your data" section, and you should prepare your CSV files accordingly, illustrated below:
Field | x (feature) | yPred (prediction) | yTrue (ground truth) |
---|---|---|---|
Number of CSVs | 1 | multiple | 1 |
Illustration of CSV format | ![]() | ![]() | ![]() |
Note, the index columns should be excluded from the input file(s). Once the datasets are uploaded, you will see visualizations generated by these datasets.
Using the Component
Embedding the Manifold component in your app allows you to programmatically generate ML model data and visualize. Otherwise, if you have some static output from some models and want to do a one-off evaluation, you might consider using the demo app directly.
Here are the basic steps to import Manifold into your app and load data for visualizing. You can also take a look at the examples folder.
Install Manifold
$ npm install @mlvis/manifold styled-components styletron-engine-atomic styletron-react
Load and Convert Data
In order to load your data files to Manifold, use the loadLocalData
action. You could also reshape your data into the required Manifold format using dataTransformer
.
import {loadLocalData} from '@mlvis/manifold/actions';
// create the following action and pass to dispatch
loadLocalData({
fileList,
dataTransformer,
});
fileList
: {Object[]}
One or more datasets, in CSV format. Could be ones that your backend returns.
dataTransformer
: {Function}
A function that transforms fileList
into the Manifold input data format. Default:
const defaultDataTransformer = fileList => ({
x: [],
yPred: [],
yTrue: [],
});
Mount reducer
Manifold uses Redux to manage its internal state. You need to register manifoldReducer
to the main reducer of your app:
import manifoldReducer from '@mlvis/manifold/reducers';
import {combineReducers, createStore, compose} from 'redux';
const initialState = {};
const reducers = combineReducers({
// mount manifold reducer in your app
manifold: manifoldReducer,
// Your other reducers here
app: appReducer,
});
// using createStore
export default createStore(reducer, initialState);
Mount Component
If you mount manifoldReducer
in another address instead of manifold
in the step above, you need to specify the path to it when you mount the component with the getState
prop. width
and height
are both needed explicitly. If you have geospatial features and need to see them on a map, you also need a mapbox token.
import Manifold from '@mlvis/manifold';
const manifoldGetState = state => state.pathTo.manifold;
const yourMapboxToken = ...;
const Main = props => (
<Manifold
getState={manifoldGetState}
width={width}
height={height}
mapboxToken={yourMapboxToken}
/>
);
Styling
Manifold uses baseui, which uses Styletron as a styling engine. If you don't already use Styletron in other parts of your app, make sure to wrap Manifold with the styletron provider.
Manifold uses the baseui theming API. The default theme used by Manifold is exported as THEME
. You can customize the styling by extending THEME
and passing it as a theme
prop of the Manifold
component.
import Manifold, {THEME} from '@mlvis/manifold';
import {Client as Styletron} from 'styletron-engine-atomic';
import {Provider as StyletronProvider} from 'styletron-react';
const engine = new Styletron();
const myTheme = {
...THEME,
colors: {
...THEME.colors,
primary: '#ff0000',
},
}
const Main = props => (
<StyletronProvider value={engine}>
<Manifold
getState={manifoldGetState}
theme={myTheme}
/>
</StyletronProvider>
);
Built With
Contributing
Please read our code of conduct before you contribute! You can find details for submitting pull requests in the CONTRIBUTING.md file. Refer to the issue template.
Versioning
We document versions and changes in our changelog - see the CHANGELOG.md file for details.
License
Apache 2.0 License
Top Related Projects
Data Apps & Dashboards for Python. No JavaScript Required.
Streamlit — A faster way to build and share data apps.
Panel: The powerful data exploration & web app framework for Python
Interactive Data Visualization in the browser, from Python
Declarative visualization library for Python
1 Line of code data quality profiling & exploratory data analysis for Pandas and Spark DataFrames.
Convert
designs to code with AI
Introducing Visual Copilot: A new AI model to turn Figma designs to high quality code using your components.
Try Visual Copilot