Top Related Projects
Code release for NeRF (Neural Radiance Fields)
A PyTorch implementation of NeRF (Neural Radiance Fields) that reproduces the results.
Instant neural graphics primitives: lightning fast NeRF and more
PyTorch3D is FAIR's library of reusable components for deep learning with 3D data
Google Research
A collaboration friendly studio for NeRFs
Quick Overview
kwea123/nerf_pl is a PyTorch Lightning implementation of NeRF (Neural Radiance Fields). It provides a flexible and efficient framework for training and evaluating NeRF models, which are used for novel view synthesis and 3D scene reconstruction from 2D images.
Pros
- Implements NeRF using PyTorch Lightning, offering better code organization and easier training management
- Supports multiple datasets and provides pre-trained models for quick experimentation
- Includes various optimizations and improvements over the original NeRF implementation
- Offers a modular design, making it easier to extend and modify for custom use cases
Cons
- Requires significant computational resources for training, especially for complex scenes
- May have a steeper learning curve for users unfamiliar with PyTorch Lightning
- Limited documentation compared to some other NeRF implementations
- Might not include all the latest NeRF variants and improvements
Code Examples
- Loading a dataset:
from datasets import dataset_dict
dataset = dataset_dict['blender']('config/blender.yml')
- Creating a NeRF model:
from models.nerf import NeRF
model = NeRF(num_layers=8, hidden_dim=256)
- Training the model:
from pytorch_lightning import Trainer
from models.rendering import NeRFRenderer
renderer = NeRFRenderer(model)
trainer = Trainer(max_epochs=30, gpus=1)
trainer.fit(renderer, train_dataloader, val_dataloader)
Getting Started
- Clone the repository:
git clone https://github.com/kwea123/nerf_pl.git
cd nerf_pl
- Install dependencies:
pip install -r requirements.txt
- Download a dataset (e.g., Blender):
bash download_example_data.sh
- Train the model:
python train.py --dataset_name blender --root_dir data/nerf_synthetic/lego --exp_name lego --num_epochs 30
- Render novel views:
python eval.py --root_dir data/nerf_synthetic/lego --dataset_name blender --scene_name lego --exp_name lego
Competitor Comparisons
Code release for NeRF (Neural Radiance Fields)
Pros of NeRF
- Original implementation by the authors of the NeRF paper
- Provides a reference implementation for understanding the core NeRF algorithm
- Includes additional features like depth supervision and view dependence
Cons of NeRF
- Written in TensorFlow, which may be less popular among some researchers
- Less optimized for speed compared to more recent implementations
- Lacks some modern features and improvements found in newer NeRF variants
Code Comparison
NeRF (TensorFlow):
def create_nerf(args):
embed_fn, input_ch = get_embedder(args.multires, args.i_embed)
embeddirs_fn, input_ch_views = get_embedder(args.multires_views, args.i_embed)
output_ch = 5 if args.N_importance > 0 else 4
skips = [4]
model = init_nerf_model(D=args.netdepth, W=args.netwidth,
input_ch=input_ch, output_ch=output_ch, skips=skips,
input_ch_views=input_ch_views, use_viewdirs=args.use_viewdirs)
nerf_pl (PyTorch):
class NeRF(nn.Module):
def __init__(self,
D=8, W=256,
in_channels_xyz=63, in_channels_dir=27,
skips=[4]):
super().__init__()
self.D = D
self.W = W
self.in_channels_xyz = in_channels_xyz
self.in_channels_dir = in_channels_dir
self.skips = skips
A PyTorch implementation of NeRF (Neural Radiance Fields) that reproduces the results.
Pros of nerf-pytorch
- More straightforward implementation, closely following the original NeRF paper
- Easier to understand for those new to NeRF concepts
- Includes a colab notebook for quick experimentation
Cons of nerf-pytorch
- Less optimized for performance compared to nerf_pl
- Fewer features and customization options
- Limited support for advanced NeRF variants
Code Comparison
nerf-pytorch:
def get_rays(H, W, K, c2w):
i, j = torch.meshgrid(torch.linspace(0, W-1, W), torch.linspace(0, H-1, H))
i = i.t()
j = j.t()
dirs = torch.stack([(i-K[0][2])/K[0][0], -(j-K[1][2])/K[1][1], -torch.ones_like(i)], -1)
rays_d = torch.sum(dirs[..., np.newaxis, :] * c2w[:3,:3], -1)
rays_o = c2w[:3,-1].expand(rays_d.shape)
return rays_o, rays_d
nerf_pl:
def get_rays(directions, c2w):
rays_d = directions @ c2w[:3, :3].T
rays_o = c2w[:3, 3].expand(rays_d.shape)
return rays_o, rays_d
The nerf_pl implementation is more concise and optimized, while nerf-pytorch provides a more detailed breakdown of the ray generation process.
Instant neural graphics primitives: lightning fast NeRF and more
Pros of instant-ngp
- Significantly faster rendering and training times
- Supports real-time rendering and interactive visualization
- Utilizes GPU acceleration for improved performance
Cons of instant-ngp
- More complex implementation, potentially harder to understand and modify
- Requires specific hardware (NVIDIA GPU) for optimal performance
- Less flexibility in terms of customization compared to nerf_pl
Code Comparison
instant-ngp:
__global__ void render_kernel(
const uint32_t n_elements,
const uint32_t n_rays,
BoundingBox aabb,
const uint32_t max_samples,
const float cone_angle_constant,
// ... (additional parameters)
) {
// Complex CUDA kernel implementation
}
nerf_pl:
def render_rays(models, embeddings,
rays, N_samples, use_disp,
perturb, noise_std, N_importance,
chunk, white_back, test_time=False,
**kwargs):
# Python implementation of ray rendering
The code comparison shows that instant-ngp uses CUDA for GPU acceleration, while nerf_pl is implemented in Python, which is more accessible but potentially slower.
PyTorch3D is FAIR's library of reusable components for deep learning with 3D data
Pros of pytorch3d
- Comprehensive 3D deep learning library with a wide range of functionalities
- Backed by Facebook Research, ensuring regular updates and support
- Extensive documentation and tutorials available
Cons of pytorch3d
- Steeper learning curve due to its broader scope
- May be overkill for projects focused solely on NeRF implementations
Code Comparison
pytorch3d example:
import torch
from pytorch3d.structures import Meshes
from pytorch3d.renderer import Textures
verts = torch.randn(4, 3)
faces = torch.tensor([[0, 1, 2], [1, 2, 3]])
mesh = Meshes(verts=[verts], faces=[faces])
nerf_pl example:
import torch
from models.nerf import NeRF
model = NeRF()
rays_o = torch.randn(1, 3)
rays_d = torch.randn(1, 3)
output = model(rays_o, rays_d)
pytorch3d offers a more comprehensive set of tools for 3D deep learning, while nerf_pl provides a focused implementation of NeRF. The choice between them depends on the specific requirements of your project and your familiarity with 3D computer vision concepts.
Google Research
Pros of google-research
- Extensive collection of research projects covering various AI and ML domains
- Official repository from Google, ensuring high-quality and well-maintained code
- Regular updates and contributions from Google researchers
Cons of google-research
- Large repository size may be overwhelming for users seeking specific implementations
- Less focused on NeRF specifically, requiring more effort to find relevant code
- May have more complex dependencies due to the diverse range of projects
Code Comparison
google-research (NeRF-related code snippet):
def render_rays(ray_batch,
network_fn,
network_query_fn,
N_samples,
retraw=False,
lindisp=False,
perturb=0.,
N_importance=0,
network_fine=None,
white_bkgd=False,
raw_noise_std=0.,
verbose=False):
# ... (implementation details)
nerf_pl:
def render_rays(models,
embeddings,
rays,
N_samples=64,
use_disp=False,
perturb=0,
noise_std=1,
N_importance=0,
chunk=1024*32,
white_background=False,
test_time=False,
**kwargs):
# ... (implementation details)
Both repositories provide implementations for NeRF, but google-research offers a broader scope of research projects, while nerf_pl focuses specifically on NeRF implementation using PyTorch Lightning.
A collaboration friendly studio for NeRFs
Pros of nerfstudio
- More comprehensive and feature-rich, offering a wider range of NeRF methods and tools
- Better documentation and user-friendly interface, making it easier for beginners to get started
- Active development and community support, with frequent updates and improvements
Cons of nerfstudio
- Higher complexity and steeper learning curve for customization
- Potentially slower training and inference times due to additional features and abstractions
Code Comparison
nerf_pl:
class NeRF(nn.Module):
def __init__(self,
D=8, W=256,
in_channels_xyz=3, in_channels_dir=3,
skips=[4]):
super().__init__()
self.D = D
self.W = W
self.skips = skips
nerfstudio:
class NeRFModel(Model):
config: ModelConfig
def populate_modules(self):
"""Set the fields and modules."""
super().populate_modules()
self.field = TCNNNerfactoField(
self.config.scene_bounds.aabb,
num_layers=self.config.num_layers,
hidden_dim=self.config.hidden_dim,
geo_feat_dim=self.config.geo_feat_dim,
num_layers_color=self.config.num_layers_color,
hidden_dim_color=self.config.hidden_dim_color,
use_appearance_embedding=self.config.use_appearance_embedding,
)
Convert
designs to code with AI
Introducing Visual Copilot: A new AI model to turn Figma designs to high quality code using your components.
Try Visual CopilotREADME
nerf_pl
Update: NVIDIA open-sourced a lightning-fast version of NeRF: NGP. I re-implemented in pytorch here. This version is ~100x faster than this repo with also better quality!
Update: an improved NSFF implementation to handle dynamic scene is open!
Update: NeRF-W (NeRF in the Wild) implementation is added to nerfw branch!
Update: The lastest code (using the latest libraries) will be updated to dev branch. The master branch remains to support the colab files. If you don't use colab, it is recommended to switch to dev branch. Only issues of the dev and nerfw branch will be considered currently.
:gem: Project page (live demo!)
Unofficial implementation of NeRF (Neural Radiance Fields) using pytorch (pytorch-lightning). This repo doesn't aim at reproducibility, but aim at providing a simpler and faster training procedure (also simpler code with detailed comments to help to understand the work). Moreover, I try to extend much more opportunities by integrating this algorithm into game engine like Unity.
Official implementation: nerf .. Reference pytorch implementation: nerf-pytorch
Recommend to read: A detailed NeRF extension list: awesome-NeRF
:milky_way: Features
- Multi-gpu training: Training on 8 GPUs finishes within 1 hour for the synthetic dataset!
- Colab notebooks to allow easy usage!
- Reconstruct colored mesh!
- Mixed Reality in Unity!
- REAL TIME volume rendering in Unity!
- Portable Scenes to let you play with other people's scenes!
You can find the Unity project including mesh, mixed reality and volume rendering here! See README_Unity for generating your own data for Unity rendering!
:beginner: Tutorial
What can NeRF do?
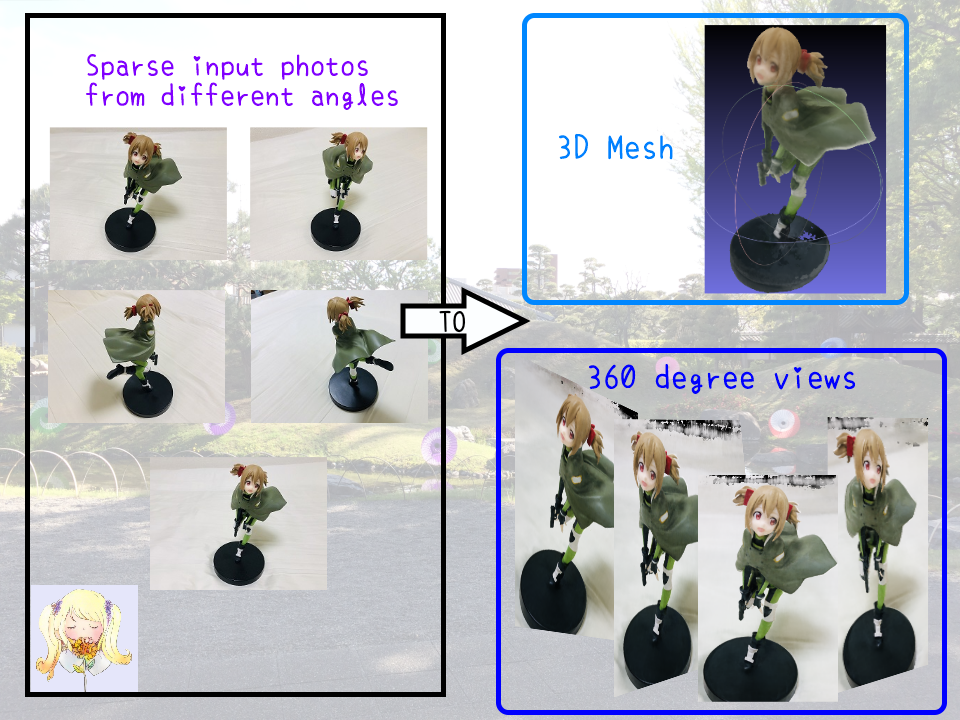
Tutorial videos
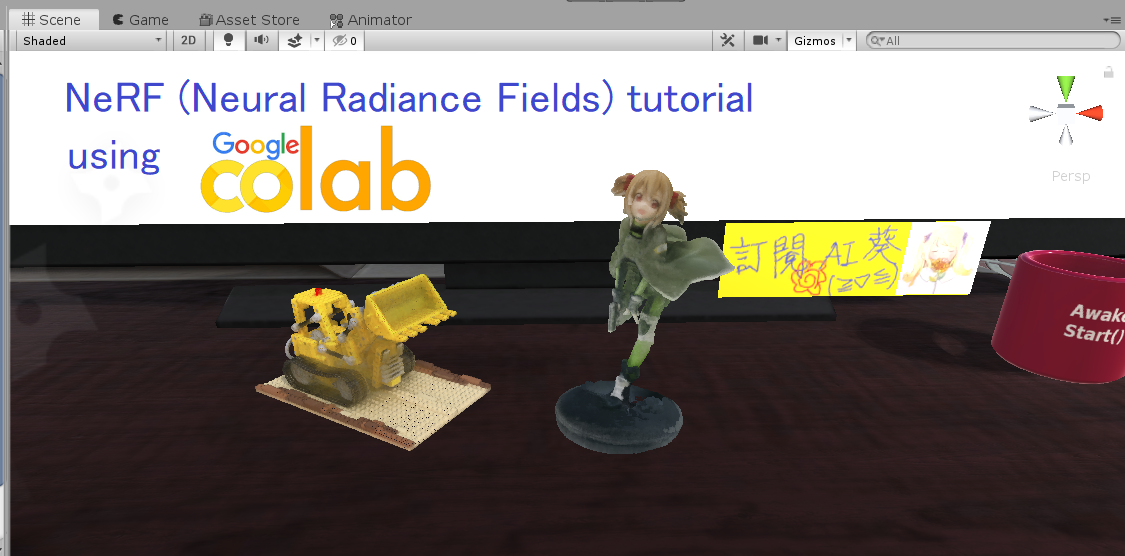
:computer: Installation
Hardware
- OS: Ubuntu 18.04
- NVIDIA GPU with CUDA>=10.1 (tested with 1 RTX2080Ti)
Software
- Clone this repo by
git clone --recursive https://github.com/kwea123/nerf_pl
- Python>=3.6 (installation via anaconda is recommended, use
conda create -n nerf_pl python=3.6
to create a conda environment and activate it byconda activate nerf_pl
) - Python libraries
- Install core requirements by
pip install -r requirements.txt
- Install
torchsearchsorted
bycd torchsearchsorted
thenpip install .
- Install core requirements by
:key: Training
Please see each subsection for training on different datasets. Available training datasets:
- Blender (Realistic Synthetic 360)
- LLFF (Real Forward-Facing)
- Your own data (Forward-Facing/360 inward-facing)
Blender
Steps
Data download
Download nerf_synthetic.zip
from here
Training model
Run (example)
python train.py \
--dataset_name blender \
--root_dir $BLENDER_DIR \
--N_importance 64 --img_wh 400 400 --noise_std 0 \
--num_epochs 16 --batch_size 1024 \
--optimizer adam --lr 5e-4 \
--lr_scheduler steplr --decay_step 2 4 8 --decay_gamma 0.5 \
--exp_name exp
These parameters are chosen to best mimic the training settings in the original repo. See opt.py for all configurations.
NOTE: the above configuration doesn't work for some scenes like drums
, ship
. In that case, consider increasing the batch_size
or change the optimizer
to radam
. I managed to train on all scenes with these modifications.
You can monitor the training process by tensorboard --logdir logs/
and go to localhost:6006
in your browser.
LLFF
Steps
Data download
Download nerf_llff_data.zip
from here
Training model
Run (example)
python train.py \
--dataset_name llff \
--root_dir $LLFF_DIR \
--N_importance 64 --img_wh 504 378 \
--num_epochs 30 --batch_size 1024 \
--optimizer adam --lr 5e-4 \
--lr_scheduler steplr --decay_step 10 20 --decay_gamma 0.5 \
--exp_name exp
These parameters are chosen to best mimic the training settings in the original repo. See opt.py for all configurations.
You can monitor the training process by tensorboard --logdir logs/
and go to localhost:6006
in your browser.
Your own data
Steps
- Install COLMAP following installation guide
- Prepare your images in a folder (around 20 to 30 for forward facing, and 40 to 50 for 360 inward-facing)
- Clone LLFF and run
python img2poses.py $your-images-folder
- Train the model using the same command as in LLFF. If the scene is captured in a 360 inward-facing manner, add
--spheric
argument.
For more details of training a good model, please see the video here.
Pretrained models and logs
Download the pretrained models and training logs in release.
Comparison with other repos
training GPU memory in GB | Speed (1 step) | |
---|---|---|
Original | 8.5 | 0.177s |
Ref pytorch | 6.0 | 0.147s |
This repo | 3.2 | 0.12s |
The speed is measured on 1 RTX2080Ti. Detailed profile can be found in release. Training memory is largely reduced, since the original repo loads the whole data to GPU at the beginning, while we only pass batches to GPU every step.
:mag_right: Testing
See test.ipynb for a simple view synthesis and depth prediction on 1 image.
Use eval.py to create the whole sequence of moving views. E.g.
python eval.py \
--root_dir $BLENDER \
--dataset_name blender --scene_name lego \
--img_wh 400 400 --N_importance 64 --ckpt_path $CKPT_PATH
IMPORTANT : Don't forget to add --spheric_poses
if the model is trained under --spheric
setting!
It will create folder results/{dataset_name}/{scene_name}
and run inference on all test data, finally create a gif out of them.
Example of lego scene using pretrained model and the reconstructed colored mesh: (PSNR=31.39, paper=32.54)
Example of fern scene using pretrained model:
Example of own scene (Silica GGO figure) and the reconstructed colored mesh. Click to link to youtube video.
Portable scenes
The concept of NeRF is that the whole scene is compressed into a NeRF model, then we can render from any pose we want. To render from plausible poses, we can leverage the training poses; therefore, you can generate video with only the trained model and the poses (hence the name of portable scenes). I provided my silica model in release, feel free to play around with it!
If you trained some interesting scenes, you are also welcomed to share the model (and the poses_bounds.npy
) by sending me an email, or post in issues! After all, a model is just around 5MB! Please run python utils/save_weights_only.py --ckpt_path $YOUR_MODEL_PATH
to extract the final model.
:ribbon: Mesh
See README_mesh for reconstruction of colored mesh. Only supported for blender dataset and 360 inward-facing data!
:warning: Notes on differences with the original repo
- The learning rate decay in the original repo is by step, which means it decreases every step, here I use learning rate decay by epoch, which means it changes only at the end of 1 epoch.
- The validation image for LLFF dataset is chosen as the most centered image here, whereas the original repo chooses every 8th image.
- The rendering spiral path is slightly different from the original repo (I use approximate values to simplify the code).
:mortar_board: COLAB
I also prepared colab notebooks that allow you to run the algorithm on any machine without GPU requirement.
- colmap to prepare camera poses for your own training data
- nerf to train on your data
- extract_mesh to extract colored mesh
Please see this playlist for the detailed tutorials.
:jack_o_lantern: SHOWOFF
We can incorporate ray tracing techniques into the volume rendering pipeline, and realize realistic scene editing (following is the materials
scene with an object removed, and a mesh is inserted and rendered with ray tracing). The code will not be released.
With my integration in Unity, I can realize realistic mixed reality photos (note my character casts shadow on the scene, zero post- image editing required):
BTW, I would like to visit the museum one day...
:book: Citation
If you use (part of) my code or find my work helpful, please consider citing
@misc{queianchen_nerf,
author={Quei-An, Chen},
title={Nerf_pl: a pytorch-lightning implementation of NeRF},
url={https://github.com/kwea123/nerf_pl/},
year={2020},
}
Top Related Projects
Code release for NeRF (Neural Radiance Fields)
A PyTorch implementation of NeRF (Neural Radiance Fields) that reproduces the results.
Instant neural graphics primitives: lightning fast NeRF and more
PyTorch3D is FAIR's library of reusable components for deep learning with 3D data
Google Research
A collaboration friendly studio for NeRFs
Convert
designs to code with AI
Introducing Visual Copilot: A new AI model to turn Figma designs to high quality code using your components.
Try Visual Copilot