Top Related Projects
PyTorch3D is FAIR's library of reusable components for deep learning with 3D data
A Code Release for Mip-NeRF 360, Ref-NeRF, and RawNeRF
Instant neural graphics primitives: lightning fast NeRF and more
Code release for NeRF (Neural Radiance Fields)
NeRF (Neural Radiance Fields) and NeRF in the Wild using pytorch-lightning
Plenoxels: Radiance Fields without Neural Networks
Quick Overview
Nerfstudio is an open-source project aimed at making Neural Radiance Fields (NeRF) research more accessible and efficient. It provides a modular and extensible framework for developing, training, and evaluating NeRF models, with a focus on ease of use and rapid experimentation.
Pros
- Modular architecture allowing easy customization and extension of NeRF models
- Comprehensive documentation and tutorials for beginners and advanced users
- Supports multiple NeRF variants and includes state-of-the-art implementations
- Efficient training and rendering pipelines optimized for various hardware setups
Cons
- Steep learning curve for users unfamiliar with NeRF concepts
- Resource-intensive, requiring powerful GPUs for optimal performance
- Limited support for real-time rendering in complex scenes
- Some advanced features may require additional setup or dependencies
Code Examples
- Loading a dataset and creating a NeRF model:
from nerfstudio.data.dataparsers.blender_dataparser import BlenderDataParserConfig
from nerfstudio.models.instant_ngp import InstantNGPModelConfig
dataparser = BlenderDataParserConfig(data="path/to/dataset")
model = InstantNGPModelConfig()
- Training a NeRF model:
from nerfstudio.pipelines.base_pipeline import VanillaPipelineConfig
from nerfstudio.engine.trainer import Trainer
pipeline = VanillaPipelineConfig(dataparser=dataparser, model=model)
trainer = Trainer(pipeline=pipeline, max_num_iterations=30000)
trainer.train()
- Rendering images from a trained model:
from nerfstudio.cameras.cameras import Cameras
from nerfstudio.model_components.renderers import RGBRenderer
cameras = Cameras.from_json("path/to/cameras.json")
renderer = RGBRenderer()
outputs = model.get_outputs_for_camera(cameras[0])
rgb_image = renderer(outputs["rgb"])
Getting Started
To get started with Nerfstudio, follow these steps:
- Install Nerfstudio:
pip install nerfstudio
- Download a sample dataset:
ns-download-data blender
- Train a NeRF model:
ns-train instant-ngp --data data/blender/lego
- View the results:
ns-viewer --load-config outputs/instant-ngp/lego/config.yml
For more detailed instructions and advanced usage, refer to the official Nerfstudio documentation.
Competitor Comparisons
PyTorch3D is FAIR's library of reusable components for deep learning with 3D data
Pros of PyTorch3D
- Broader scope: Covers a wide range of 3D deep learning tasks beyond NeRF
- Extensive documentation and tutorials
- Backed by Facebook Research, ensuring long-term support and updates
Cons of PyTorch3D
- Steeper learning curve due to its broader focus
- Less specialized for NeRF-specific tasks
- May require more setup and configuration for NeRF projects
Code Comparison
PyTorch3D example (rendering a mesh):
renderer = MeshRenderer(
rasterizer=MeshRasterizer(cameras=cameras, raster_settings=raster_settings),
shader=HardPhongShader(device=device, cameras=cameras)
)
images = renderer(meshes, lights=lights, materials=materials)
Nerfstudio example (rendering a NeRF):
model = NerfactoModel(config=config)
outputs = model.get_outputs(ray_bundle)
rgb = outputs["rgb"]
depth = outputs["depth"]
Both libraries offer powerful 3D rendering capabilities, but PyTorch3D provides a more general-purpose toolkit for 3D deep learning, while Nerfstudio focuses specifically on NeRF implementations and optimizations.
A Code Release for Mip-NeRF 360, Ref-NeRF, and RawNeRF
Pros of multinerf
- Developed by Google Research, potentially benefiting from extensive resources and expertise
- Focuses specifically on multi-view neural radiance fields, which may offer more specialized functionality
- Implements advanced techniques like mip-NeRF 360 and ref-NeRF for improved rendering quality
Cons of multinerf
- Less active development and community support compared to nerfstudio
- More complex setup and usage, potentially requiring more technical expertise
- Limited documentation and tutorials, which may make it harder for beginners to get started
Code Comparison
multinerf:
config = configs.Config(
dataset=configs.dataset('blender'),
model=configs.model('mipnerf'),
train=configs.train(batch_size=4096),
)
nerfstudio:
from nerfstudio.pipelines.base_pipeline import VanillaPipeline
from nerfstudio.data.datamanagers.base_datamanager import VanillaDataManager
from nerfstudio.models.nerfacto import NerfactoModel
pipeline = VanillaPipeline(
datamanager=VanillaDataManager(),
model=NerfactoModel()
)
The code comparison shows that nerfstudio offers a more modular and user-friendly API, while multinerf's configuration approach may be more concise but potentially less flexible for customization.
Instant neural graphics primitives: lightning fast NeRF and more
Pros of instant-ngp
- Extremely fast rendering and training times
- Highly optimized CUDA implementation for real-time performance
- Supports a wide range of 3D representations beyond just NeRFs
Cons of instant-ngp
- Less user-friendly and harder to set up for beginners
- Limited flexibility for customization and experimentation
- Primarily focused on NVIDIA GPUs, limiting hardware compatibility
Code Comparison
instant-ngp:
__global__ void render_kernel(
const uint32_t n_elements,
const uint32_t n_rays,
BoundingBox aabb,
const uint32_t n_cascades,
...
) {
// Complex CUDA kernel implementation
}
nerfstudio:
@dataclass
class NerfactoModelConfig(ModelConfig):
_target: Type = field(default_factory=lambda: NerfactoModel)
near_plane: float = 0.05
far_plane: float = 1000.0
background_color: Literal["random", "last_sample", "white", "black"] = "last_sample"
...
The code snippets highlight the difference in implementation approaches, with instant-ngp focusing on low-level CUDA optimization and nerfstudio providing a more high-level, Python-based interface for configuration and customization.
Code release for NeRF (Neural Radiance Fields)
Pros of nerf
- Original implementation of NeRF, serving as a foundational reference
- Lightweight and focused solely on the core NeRF algorithm
- Easier to understand and modify for research purposes
Cons of nerf
- Limited features and less user-friendly compared to nerfstudio
- Lacks support for more advanced NeRF variants and optimizations
- Less active development and community support
Code Comparison
nerf:
def create_nerf(args):
embed_fn, input_ch = get_embedder(args.multires, args.i_embed)
embeddirs_fn, input_ch_views = get_embedder(args.multires_views, args.i_embed)
output_ch = 5 if args.N_importance > 0 else 4
skips = [4]
model = NeRF(D=args.netdepth, W=args.netwidth,
input_ch=input_ch, output_ch=output_ch, skips=skips,
input_ch_views=input_ch_views, use_viewdirs=args.use_viewdirs)
return model
nerfstudio:
class VanillaNerfModel(Model):
config: VanillaNerfModelConfig
def populate_modules(self):
self.field = VanillaNeRFField(
position_encoding=self.config.position_encoding,
direction_encoding=self.config.direction_encoding,
)
self.renderer_rgb = RGBRenderer(background_color=self.config.background_color)
self.renderer_depth = DepthRenderer()
NeRF (Neural Radiance Fields) and NeRF in the Wild using pytorch-lightning
Pros of nerf_pl
- Lightweight and focused implementation, making it easier to understand and modify
- Supports multiple NeRF variants out of the box (e.g., NeRF, NeRF++, TensoRF)
- Utilizes PyTorch Lightning for streamlined training and deployment
Cons of nerf_pl
- Less extensive documentation and community support compared to nerfstudio
- Fewer built-in features and tools for experimentation and visualization
- May require more manual setup and configuration for advanced use cases
Code Comparison
nerf_pl:
class NeRF(nn.Module):
def __init__(self, D=8, W=256, input_ch=3, input_ch_views=3, output_ch=4, skips=[4], use_viewdirs=False):
super(NeRF, self).__init__()
self.D = D
self.W = W
self.input_ch = input_ch
self.input_ch_views = input_ch_views
self.skips = skips
self.use_viewdirs = use_viewdirs
nerfstudio:
class NeRFModel(Model):
config: ModelConfig
field: Field
def __init__(
self,
config: ModelConfig,
**kwargs,
) -> None:
super().__init__(config=config, **kwargs)
self.field = instantiate(config.field)
Both implementations define NeRF models, but nerfstudio uses a more modular approach with configuration-based instantiation, while nerf_pl opts for a more direct, lightweight implementation.
Plenoxels: Radiance Fields without Neural Networks
Pros of svox2
- Faster rendering speed, especially for large scenes
- Lower memory consumption during training and inference
- Supports both bounded and unbounded scenes
Cons of svox2
- Less extensive documentation and community support
- Fewer built-in features and tools for experimentation
- Limited compatibility with different types of NeRF variants
Code Comparison
svox2:
import svox2
grid = svox2.SparseGrid(reso=128, radius=1.0)
opt = svox2.Adam(grid.opt_params(), lr=1e-2)
renderer = svox2.VolumeRenderer(grid)
nerfstudio:
from nerfstudio.models.instant_ngp import InstantNGPModelConfig
from nerfstudio.pipelines.base_pipeline import VanillaPipelineConfig
config = VanillaPipelineConfig(
model=InstantNGPModelConfig()
)
Both repositories focus on Neural Radiance Fields (NeRF) implementations, but they have different approaches and target audiences. svox2 is more specialized and optimized for speed and memory efficiency, making it suitable for large-scale scenes and real-time applications. However, it may require more expertise to use effectively.
Nerfstudio, on the other hand, provides a more comprehensive framework with various NeRF implementations and tools for experimentation. It offers better documentation and community support, making it more accessible for beginners and researchers. However, it may not be as optimized for speed and memory usage as svox2 in certain scenarios.
Convert
designs to code with AI
Introducing Visual Copilot: A new AI model to turn Figma designs to high quality code using your components.
Try Visual CopilotREADME
A collaboration friendly studio for NeRFs
About
Itâs as simple as plug and play with nerfstudio!
Nerfstudio provides a simple API that allows for a simplified end-to-end process of creating, training, and testing NeRFs. The library supports a more interpretable implementation of NeRFs by modularizing each component. With more modular NeRFs, we hope to create a more user-friendly experience in exploring the technology.
This is a contributor-friendly repo with the goal of building a community where users can more easily build upon each other's contributions. Nerfstudio initially launched as an opensource project by Berkeley students in KAIR lab at Berkeley AI Research (BAIR) in October 2022 as a part of a research project (paper). It is currently developed by Berkeley students and community contributors.
We are committed to providing learning resources to help you understand the basics of (if you're just getting started), and keep up-to-date with (if you're a seasoned veteran) all things NeRF. As researchers, we know just how hard it is to get onboarded with this next-gen technology. So we're here to help with tutorials, documentation, and more!
Have feature requests? Want to add your brand-spankin'-new NeRF model? Have a new dataset? We welcome contributions! Please do not hesitate to reach out to the nerfstudio team with any questions via Discord.
Have feedback? We'd love for you to fill out our Nerfstudio Feedback Form if you want to let us know who you are, why you are interested in Nerfstudio, or provide any feedback!
We hope nerfstudio enables you to build faster :hammer: learn together :books: and contribute to our NeRF community :sparkling_heart:.
Sponsors
Sponsors of this work includes Luma AI and the BAIR commons.
Quickstart
The quickstart will help you get started with the default vanilla NeRF trained on the classic Blender Lego scene. For more complex changes (e.g., running with your own data/setting up a new NeRF graph), please refer to our references.
1. Installation: Setup the environment
Prerequisites
You must have an NVIDIA video card with CUDA installed on the system. This library has been tested with version 11.8 of CUDA. You can find more information about installing CUDA here
Create environment
Nerfstudio requires python >= 3.8
. We recommend using conda to manage dependencies. Make sure to install Conda before proceeding.
conda create --name nerfstudio -y python=3.8
conda activate nerfstudio
pip install --upgrade pip
Dependencies
Install PyTorch with CUDA (this repo has been tested with CUDA 11.7 and CUDA 11.8) and tiny-cuda-nn.
cuda-toolkit
is required for building tiny-cuda-nn
.
For CUDA 11.8:
pip install torch==2.1.2+cu118 torchvision==0.16.2+cu118 --extra-index-url https://download.pytorch.org/whl/cu118
conda install -c "nvidia/label/cuda-11.8.0" cuda-toolkit
pip install ninja git+https://github.com/NVlabs/tiny-cuda-nn/#subdirectory=bindings/torch
See Dependencies in the Installation documentation for more.
Installing nerfstudio
Easy option:
pip install nerfstudio
OR if you want the latest and greatest:
git clone https://github.com/nerfstudio-project/nerfstudio.git
cd nerfstudio
pip install --upgrade pip setuptools
pip install -e .
OR if you want to skip all installation steps and directly start using nerfstudio, use the docker image:
See Installation - Use docker image.
2. Training your first model!
The following will train a nerfacto model, our recommended model for real world scenes.
# Download some test data:
ns-download-data nerfstudio --capture-name=poster
# Train model
ns-train nerfacto --data data/nerfstudio/poster
If everything works, you should see training progress like the following:
Navigating to the link at the end of the terminal will load the webviewer. If you are running on a remote machine, you will need to port forward the websocket port (defaults to 7007).
Resume from checkpoint / visualize existing run
It is possible to load a pretrained model by running
ns-train nerfacto --data data/nerfstudio/poster --load-dir {outputs/.../nerfstudio_models}
Visualize existing run
Given a pretrained model checkpoint, you can start the viewer by running
ns-viewer --load-config {outputs/.../config.yml}
3. Exporting Results
Once you have a NeRF model you can either render out a video or export a point cloud.
Render Video
First we must create a path for the camera to follow. This can be done in the viewer under the "RENDER" tab. Orient your 3D view to the location where you wish the video to start, then press "ADD CAMERA". This will set the first camera key frame. Continue to new viewpoints adding additional cameras to create the camera path. We provide other parameters to further refine your camera path. Once satisfied, press "RENDER" which will display a modal that contains the command needed to render the video. Kill the training job (or create a new terminal if you have lots of compute) and run the command to generate the video.
Other video export options are available, learn more by running
ns-render --help
Generate Point Cloud
While NeRF models are not designed to generate point clouds, it is still possible. Navigate to the "EXPORT" tab in the 3D viewer and select "POINT CLOUD". If the crop option is selected, everything in the yellow square will be exported into a point cloud. Modify the settings as desired then run the command at the bottom of the panel in your command line.
Alternatively you can use the CLI without the viewer. Learn about the export options by running
ns-export pointcloud --help
4. Using Custom Data
Using an existing dataset is great, but likely you want to use your own data! We support various methods for using your own data. Before it can be used in nerfstudio, the camera location and orientations must be determined and then converted into our format using ns-process-data
. We rely on external tools for this, instructions and information can be found in the documentation.
Data | Capture Device | Requirements | ns-process-data Speed |
---|---|---|---|
ð· Images | Any | COLMAP | ð¢ |
ð¹ Video | Any | COLMAP | ð¢ |
ð 360 Data | Any | COLMAP | ð¢ |
ð± Polycam | IOS with LiDAR | Polycam App | ð |
ð± KIRI Engine | IOS or Android | KIRI Engine App | ð |
ð± Record3D | IOS with LiDAR | Record3D app | ð |
ð± Spectacular AI | IOS, OAK, others | App / sai-cli | ð |
ð¥ Metashape | Any | Metashape | ð |
ð¥ RealityCapture | Any | RealityCapture | ð |
ð¥ ODM | Any | ODM | ð |
ð Aria | Aria glasses | Project Aria | ð |
ð Custom | Any | Camera Poses | ð |
5. Advanced Options
Training models other than nerfacto
We provide other models than nerfacto, for example if you want to train the original nerf model, use the following command
ns-train vanilla-nerf --data DATA_PATH
For a full list of included models run ns-train --help
.
Modify Configuration
Each model contains many parameters that can be changed, too many to list here. Use the --help
command to see the full list of configuration options.
ns-train nerfacto --help
Tensorboard / WandB / Viewer
We support four different methods to track training progress, using the viewertensorboard, Weights and Biases, and ,Comet. You can specify which visualizer to use by appending --vis {viewer, tensorboard, wandb, comet viewer+wandb, viewer+tensorboard, viewer+comet}
to the training command. Simultaneously utilizing the viewer alongside wandb or tensorboard may cause stuttering issues during evaluation steps. The viewer only works for methods that are fast (ie. nerfacto, instant-ngp), for slower methods like NeRF, use the other loggers.
Learn More
And that's it for getting started with the basics of nerfstudio.
If you're interested in learning more on how to create your own pipelines, develop with the viewer, run benchmarks, and more, please check out some of the quicklinks below or visit our documentation directly.
Section | Description |
---|---|
Documentation | Full API documentation and tutorials |
Viewer | Home page for our web viewer |
ð Educational | |
Model Descriptions | Description of all the models supported by nerfstudio and explanations of component parts. |
Component Descriptions | Interactive notebooks that explain notable/commonly used modules in various models. |
ð Tutorials | |
Getting Started | A more in-depth guide on how to get started with nerfstudio from installation to contributing. |
Using the Viewer | A quick demo video on how to navigate the viewer. |
Using Record3D | Demo video on how to run nerfstudio without using COLMAP. |
ð» For Developers | |
Creating pipelines | Learn how to easily build new neural rendering pipelines by using and/or implementing new modules. |
Creating datasets | Have a new dataset? Learn how to run it with nerfstudio. |
Contributing | Walk-through for how you can start contributing now. |
ð Community | |
Discord | Join our community to discuss more. We would love to hear from you! |
Follow us on Twitter @nerfstudioteam to see cool updates and announcements | |
Feedback Form | We welcome any feedback! This is our chance to learn what you all are using Nerfstudio for. |
Supported Features
We provide the following support structures to make life easier for getting started with NeRFs.
If you are looking for a feature that is not currently supported, please do not hesitate to contact the Nerfstudio Team on Discord!
- :mag_right: Web-based visualizer that allows you to:
- Visualize training in real-time + interact with the scene
- Create and render out scenes with custom camera trajectories
- View different output types
- And more!
- :pencil2: Support for multiple logging interfaces (Tensorboard, Wandb), code profiling, and other built-in debugging tools
- :chart_with_upwards_trend: Easy-to-use benchmarking scripts on the Blender dataset
- :iphone: Full pipeline support (w/ Colmap, Polycam, or Record3D) for going from a video on your phone to a full 3D render.
Built On
- Easy-to-use config system
- Developed by Brent Yi
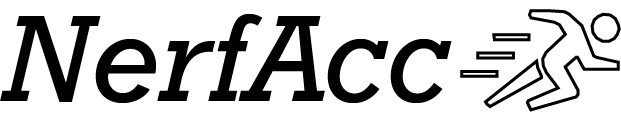
- Library for accelerating NeRF renders
- Developed by Ruilong Li
Citation
You can find a paper writeup of the framework on arXiv.
If you use this library or find the documentation useful for your research, please consider citing:
@inproceedings{nerfstudio,
title = {Nerfstudio: A Modular Framework for Neural Radiance Field Development},
author = {
Tancik, Matthew and Weber, Ethan and Ng, Evonne and Li, Ruilong and Yi, Brent
and Kerr, Justin and Wang, Terrance and Kristoffersen, Alexander and Austin,
Jake and Salahi, Kamyar and Ahuja, Abhik and McAllister, David and Kanazawa,
Angjoo
},
year = 2023,
booktitle = {ACM SIGGRAPH 2023 Conference Proceedings},
series = {SIGGRAPH '23}
}
Contributors
Top Related Projects
PyTorch3D is FAIR's library of reusable components for deep learning with 3D data
A Code Release for Mip-NeRF 360, Ref-NeRF, and RawNeRF
Instant neural graphics primitives: lightning fast NeRF and more
Code release for NeRF (Neural Radiance Fields)
NeRF (Neural Radiance Fields) and NeRF in the Wild using pytorch-lightning
Plenoxels: Radiance Fields without Neural Networks
Convert
designs to code with AI
Introducing Visual Copilot: A new AI model to turn Figma designs to high quality code using your components.
Try Visual Copilot